Developing a wind power forecasting system based on deep learning with attention mechanism
ENERGY(2022)
摘要
Large-scale wind power grid integration is challenging, requiring accurate and stable wind power forecasting to reduce operational risk and improve the scheduling efficiency of the grid. Previous studies have emphasized data preprocessing and parameter optimization. Studies on feature mining for the input layer, particularly the hidden layer, are limited, hindering further forecasting accuracy and stability improvements. To address this gap, a novel wind power forecasting system consisting of feature decomposition, self-attention, forecasting, optimization, and performance evaluation modules, is developed in this study. The feature decomposition module is used to remove superfluous noise components inherent in wind power data. A dual-stage self-attention mechanism is proposed in the self-attention module to determine the importance of driving features in the input and hidden layers, rather than focusing on all f/eatures equally. A deep learning model is used with the optimization module, built into the forecasting module. A performance evaluation module is constructed to provide a synthetical evaluation of the forecasting system. Numerical experiments are conducted to validate the effectiveness of the system and demonstrate its superiority. (C) 2022 Elsevier Ltd. All rights reserved.
更多查看译文
关键词
Attention mechanism, Deep learning, Forecasting, Optimization, Wind power
AI 理解论文
溯源树
样例
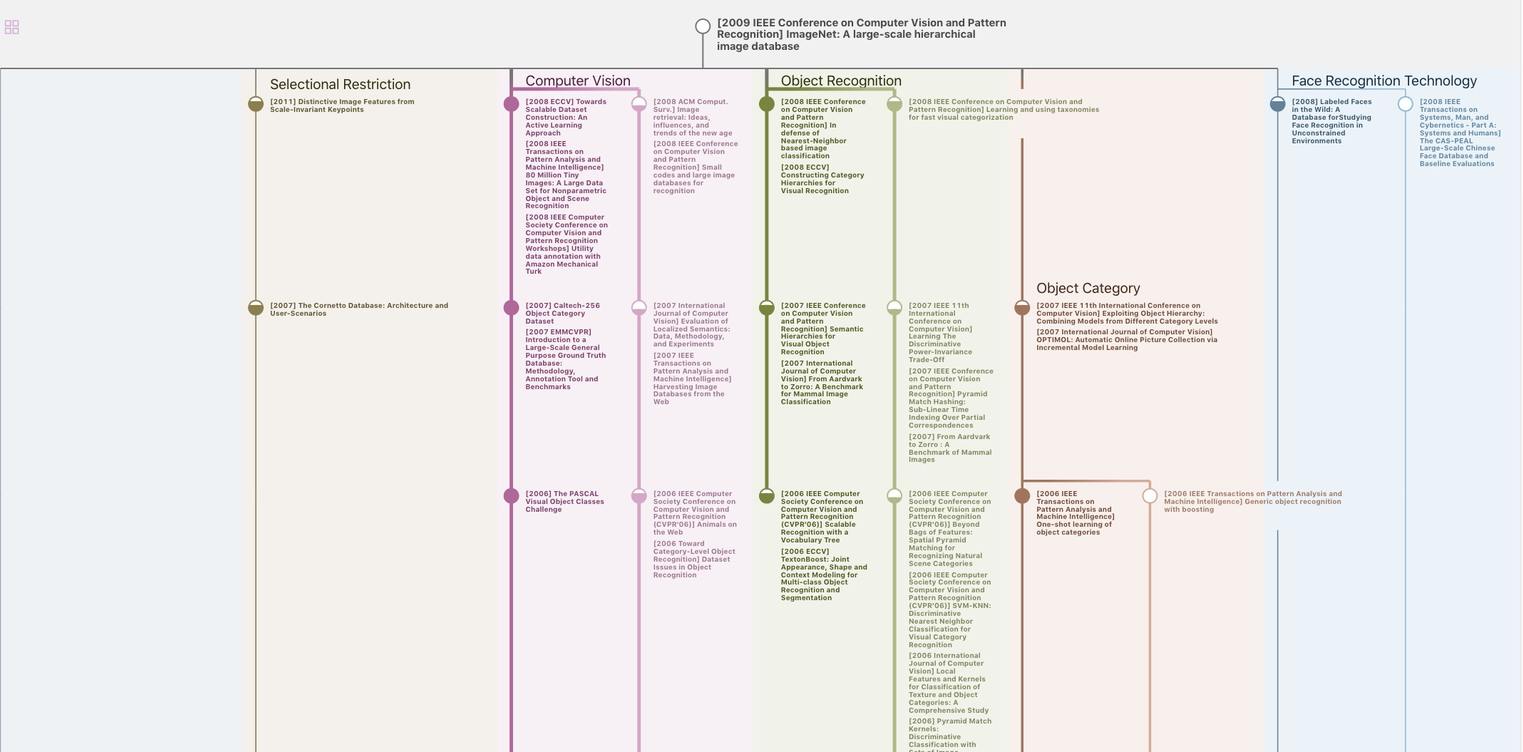
生成溯源树,研究论文发展脉络
Chat Paper
正在生成论文摘要