Deblurring Ghost Imaging Reconstruction Based on Underwater Dataset Generated by Few-Shot Learning
SENSORS(2022)
摘要
Underwater ghost imaging based on deep learning can effectively reduce the influence of forward scattering and back scattering of water. With the help of data-driven methods, high-quality results can be reconstructed. However, the training of the underwater ghost imaging requires enormous paired underwater datasets, which are difficult to obtain directly. Although the Cycle-GAN method solves the problem to some extent, the blurring degree of the fuzzy class of the paired underwater datasets generated by Cycle-GAN is relatively unitary. To solve this problem, a few-shot underwater image generative network method is proposed. Utilizing the proposed few-shot learning image generative method, the generated paired underwater datasets are better than those obtained by the Cycle-GAN method, especially under the condition of few real underwater datasets. In addition, to reconstruct high-quality results, an underwater deblurring ghost imaging method is proposed. The reconstruction method consists of two parts: reconstruction and deblurring. The experimental and simulation results show that the proposed reconstruction method has better performance in deblurring at a low sampling rate, compared with existing underwater ghost imaging methods based on deep learning. The proposed reconstruction method can effectively increase the clarity degree of the underwater reconstruction target at a low sampling rate and promotes the further applications of underwater ghost imaging.
更多查看译文
关键词
few-shot learning, underwater deblurring ghost imaging, paired underwater datasets, low sampling rate
AI 理解论文
溯源树
样例
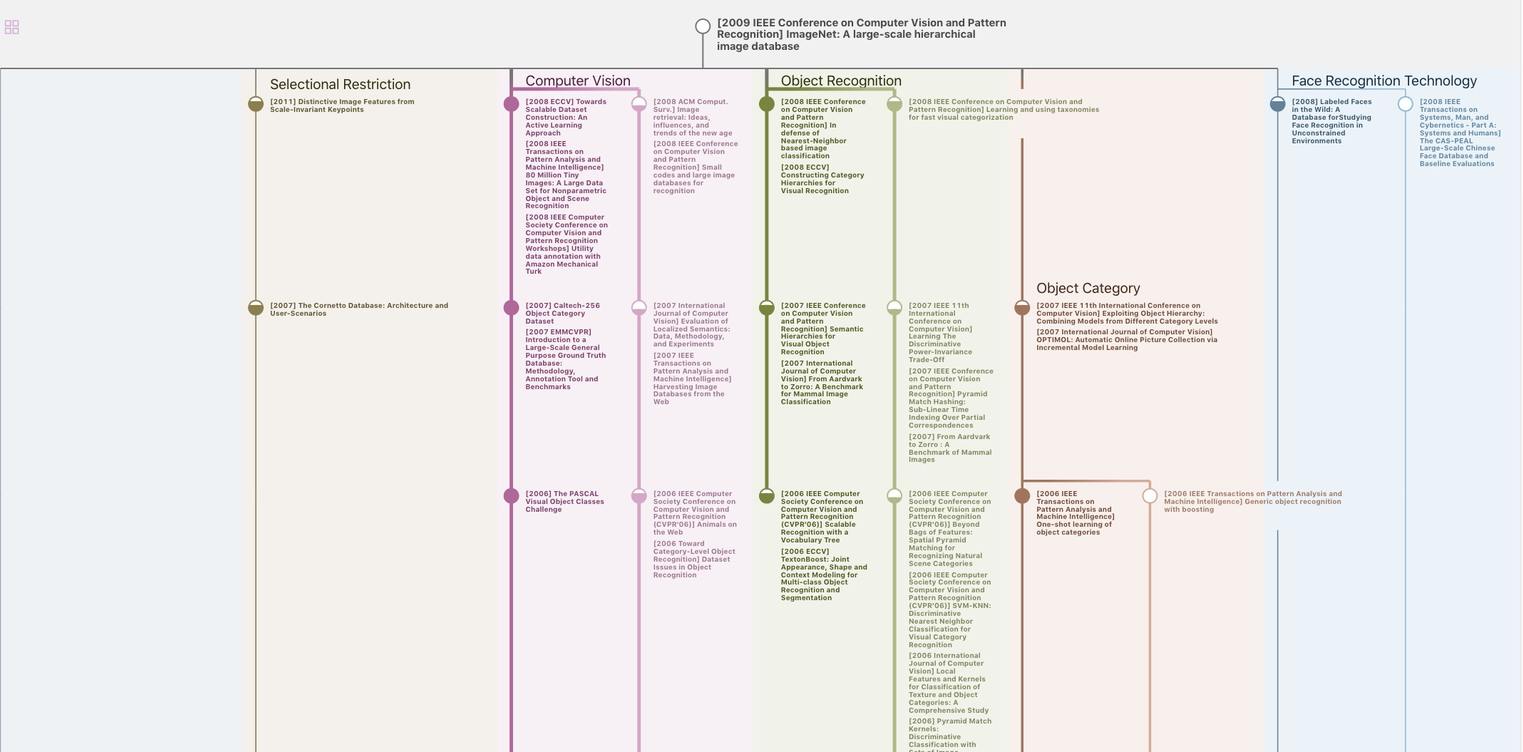
生成溯源树,研究论文发展脉络
Chat Paper
正在生成论文摘要