Bayesian Learning-Based Multiuser Detection for Grant-Free NOMA Systems
IEEE Transactions on Wireless Communications(2022)
摘要
Grant-Free Non-Orthogonal Multiple Access (GF-NOMA) is considered as a promising technology to support the massive connectivity of Machine-Type Communications (MTC). The design of efficient and high-performance multi-user detection (MUD) scheme is a challenging issue of GF-NOMA, especially when the number of active users is unknown and relatively high. This paper adopts Sparse Bayesian Learning (SBL) approaches to solve the MUD problem of GF-NOMA in MTC. The MUD problem within a certain access slot is formulated as a Single Measurement Vector (SMV) model and efficiently solved via SBL-based methods. To further improve the MUD performance, we set up a Multiple Measurement Vector (MMV) model and develop block SBL-based MUD methods, by exploiting the temporal correlation of user activity over successive access slots. Then to extend the usage of the aforementioned algorithms to the scenarios with relatively high, or quasi-sparse, user activity, we propose novel SBL-based MUD algorithms via post sparse error recovery methodology, for both the SMV and MMV problem models. Simulation results show that the proposed SBL-based MUD algorithms achieve substantial performance gain over traditional ones, especially when the number of active users is unknown and relatively high.
更多查看译文
关键词
Multiuser detection,Uplink,Wireless communication,NOMA,Detectors,Bayes methods,Compressed sensing,Machine-type communications,multiuser detection,Bayesian compressive sensing,single measurement vector,multiple measurement vector
AI 理解论文
溯源树
样例
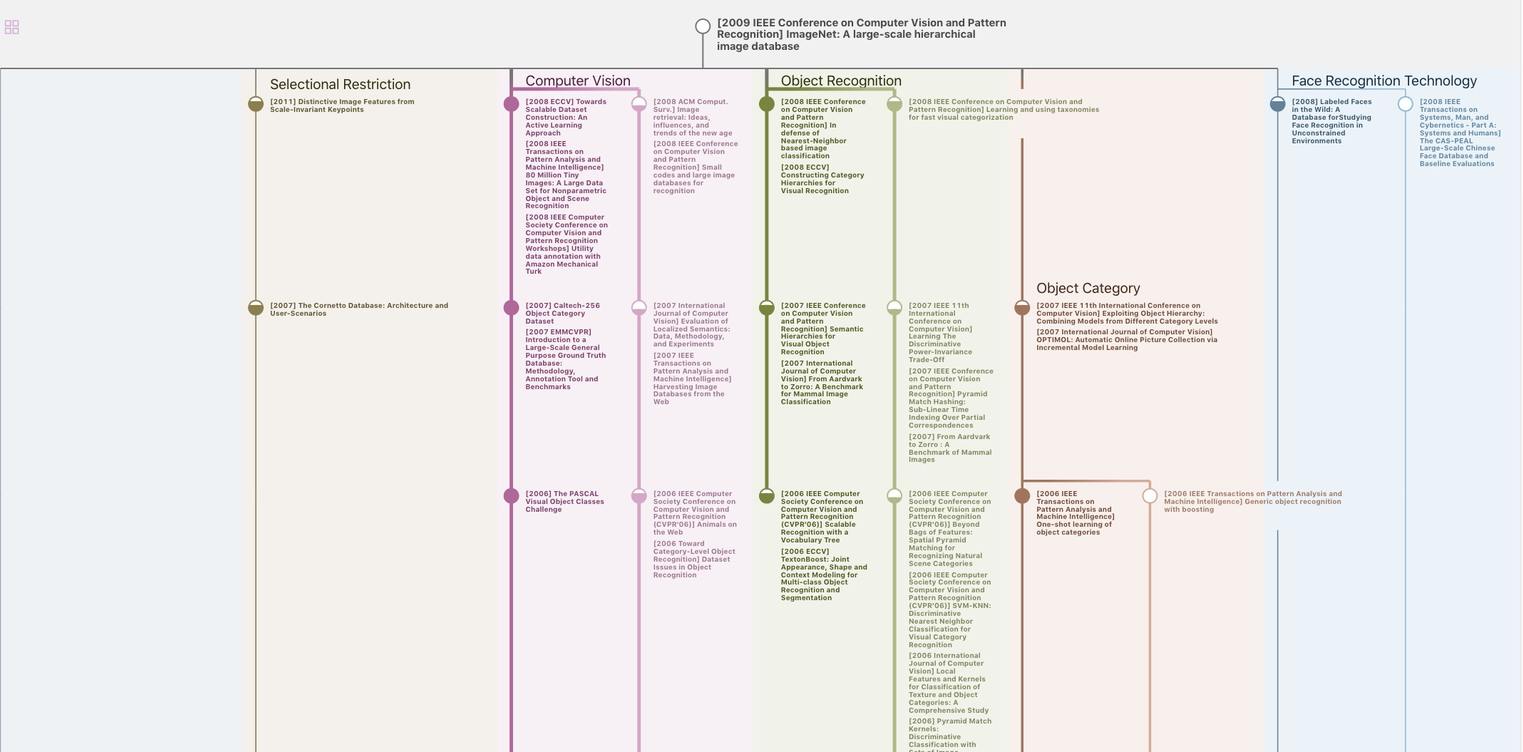
生成溯源树,研究论文发展脉络
Chat Paper
正在生成论文摘要