Efficient KPI Anomaly Detection Through Transfer Learning for Large-Scale Web Services
IEEE Journal on Selected Areas in Communications(2022)
摘要
Timely anomaly detection of key performance indicators (KPIs),
e.g.
, service response time, error rate, is of utmost importance to Web services. Over the years, many unsupervised deep learning-based anomaly detection approaches have been proposed. To achieve good performance, they require a long period of KPI data for model training, which is not easy to guarantee with frequent service changes. Additionally, the training overhead is too significant for the vast number of KPIs in large-scale Web services. To address the problems, we propose an unsupervised KPI anomaly detection approach, named
AnoTransfer
, by combining a novel Variational Auto-Encoder (VAE)-based KPI clustering algorithm with an adaptive transfer learning strategy. Extensive evaluation experiments using real-world data collected from several large-scale Web service providers demonstrate that
AnoTransfer
reduces the average initialization time by 65.71% and improves the training efficiency by 50.62 times, without significantly degrading anomaly detection accuracy.
更多查看译文
关键词
Key performance indicator,anomaly detection,time series clustering,transfer learning
AI 理解论文
溯源树
样例
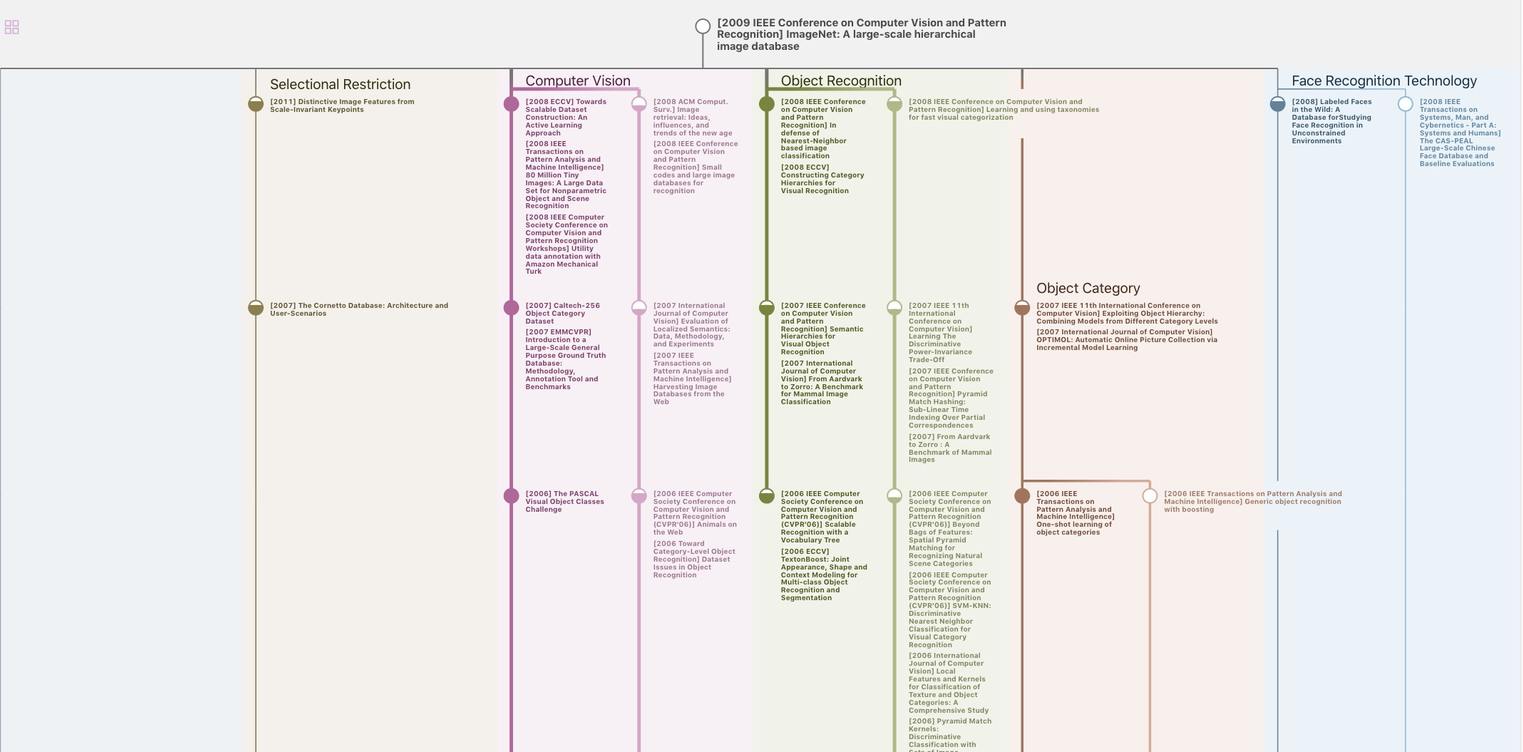
生成溯源树,研究论文发展脉络
Chat Paper
正在生成论文摘要