Deep Learning Model to Identify Potential Acetylcholinesterase Inhibitors: A Case Study of Isolated Compounds From Pongamia pinnata (L.) Pierre
NATURAL PRODUCT COMMUNICATIONS(2022)
摘要
Acetylcholinesterase (AChE) plays an essential role in the cholinergic pathways in Alzheimer's disease. This study used a deep learning model as a powerful virtual tool for discovering AChE inhibitors. The model showed 94.3% accuracy, 97.1% precision, 95.9% recall, and 86.2% specificity. A list of bioactive compounds extracted from Pongamia pinnata (L.) Pierre was selected as the test dataset. Four candidates were selected for in vitro: pongapin, ovalichromene B, gamatin, and pongaglabrone. These flavonoids showed inhibitory effects with half-maximal inhibitory concentration (IC50) values between 19.8 and 63.5 mu g/mL. In molecular analyses, these compounds showed noticeable interactions with the AChE catalytic residues Ser203 and His447 and satisfied acceptable drug-like properties and other druglikeness parameters. This study has shown that a deep learning approach can accurately predict potential compounds targeting AChE, and P. pinnata is a promising medical plant for Alzheimer's disease.
更多查看译文
关键词
deep learning, acetylcholinesterase, Alzheimer's disease, Pongamia pinnata, molecular docking, ADME
AI 理解论文
溯源树
样例
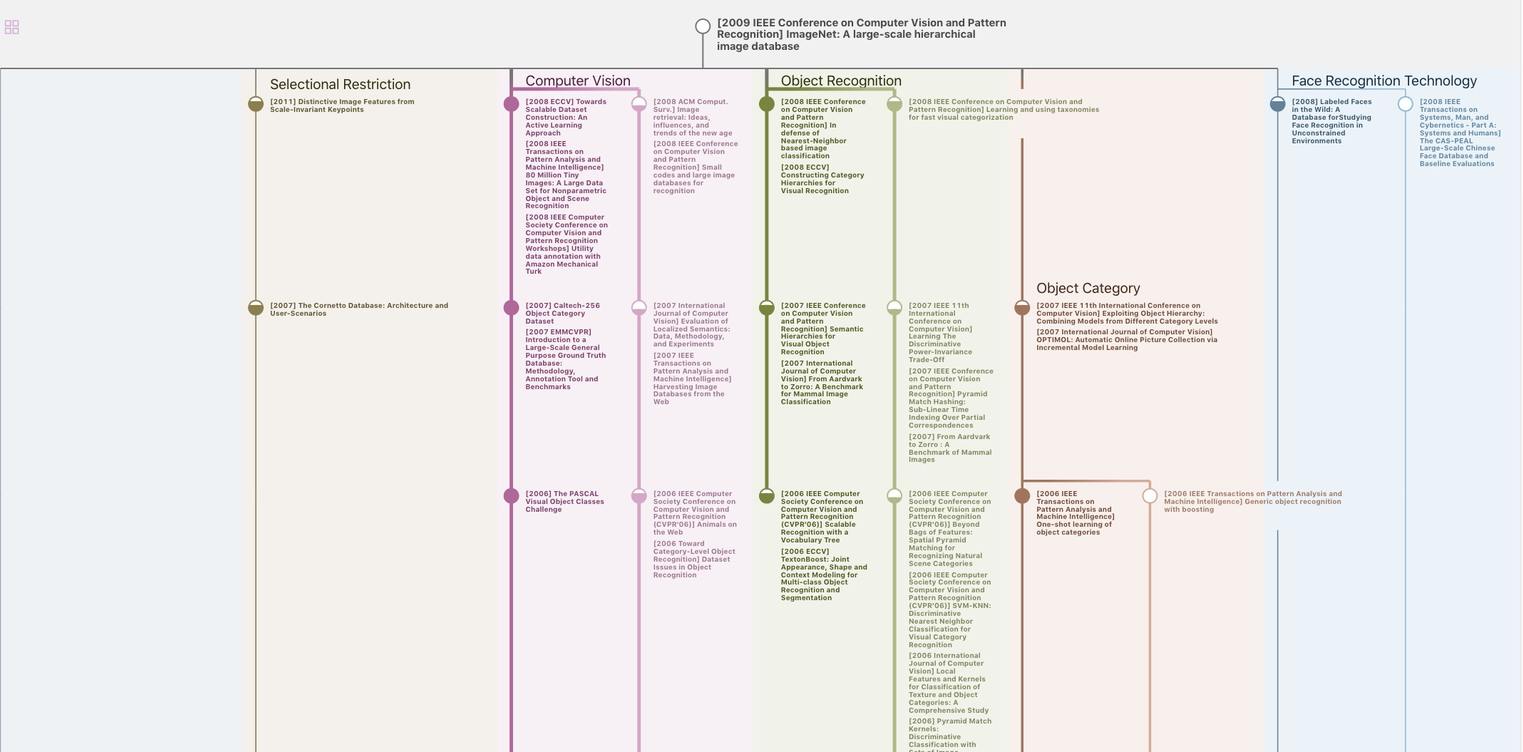
生成溯源树,研究论文发展脉络
Chat Paper
正在生成论文摘要