Explainable Artificial Intelligence: Evaluating the Objective and Subjective Impacts of xAI on Human-Agent Interaction
INTERNATIONAL JOURNAL OF HUMAN-COMPUTER INTERACTION(2023)
摘要
Intelligent agents must be able to communicate intentions and explain their decision-making processes to build trust, foster confidence, and improve human-agent team dynamics. Recognizing this need, academia and industry are rapidly proposing new ideas, methods, and frameworks to aid in the design of more explainable AI. Yet, there remains no standardized metric or experimental protocol for benchmarking new methods, leaving researchers to rely on their own intuition or ad hoc methods for assessing new concepts. In this work, we present the first comprehensive (n = 286) user study testing a wide range of approaches for explainable machine learning, including feature importance, probability scores, decision trees, counterfactual reasoning, natural language explanations, and case-based reasoning, as well as a baseline condition with no explanations. We provide the first large-scale empirical evidence of the effects of explainability on human-agent teaming. Our results will help to guide the future of explainability research by highlighting the benefits of counterfactual explanations and the shortcomings of confidence scores for explainability. We also propose a novel questionnaire to measure explainability with human participants, inspired by relevant prior work and correlated with human-agent teaming metrics.
更多查看译文
AI 理解论文
溯源树
样例
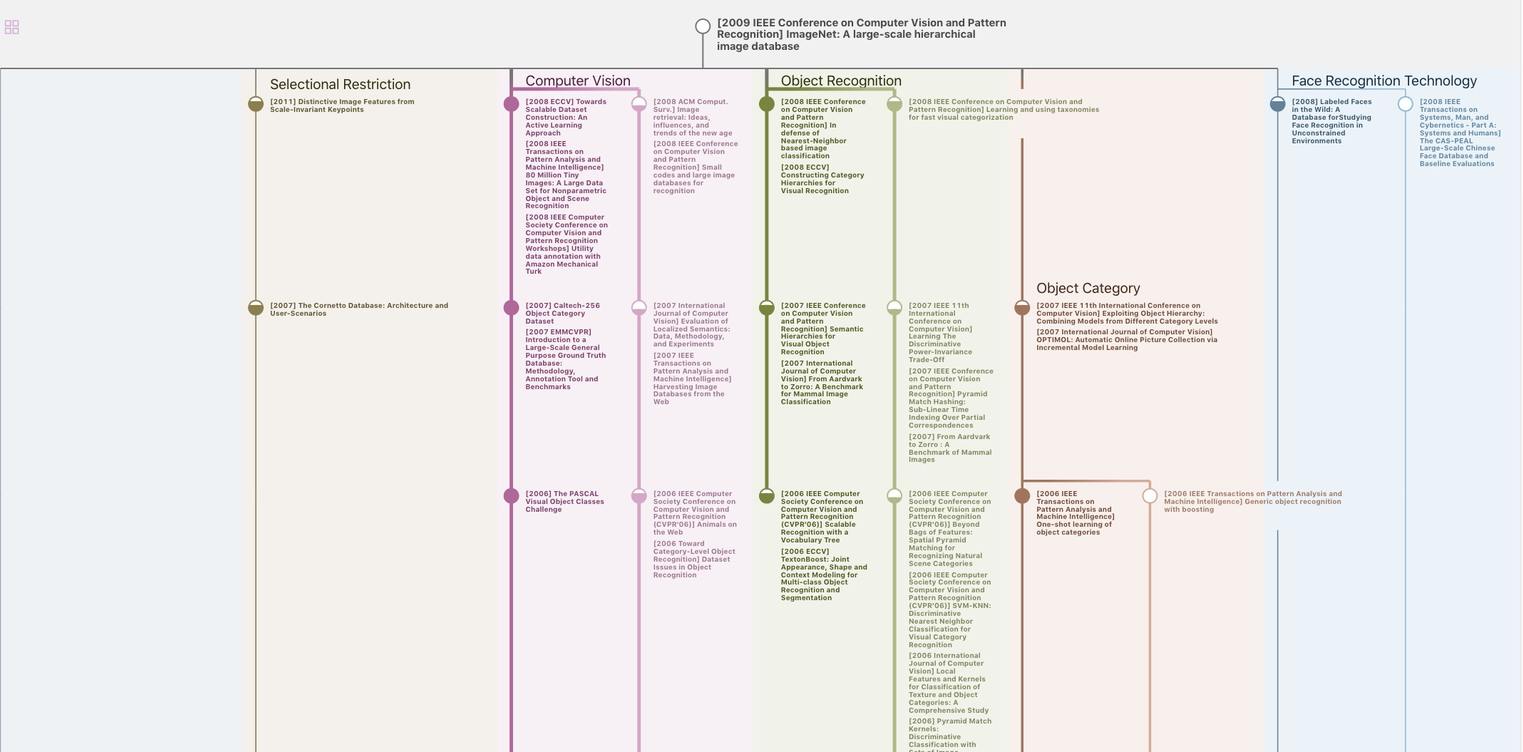
生成溯源树,研究论文发展脉络
Chat Paper
正在生成论文摘要