Adaptive enhanced swin transformer with U-net for remote sensing image segmentation*
Computers and Electrical Engineering(2022)
摘要
Semantic segmentation of remote sensing images often faces complex situations, such as variable scale objects, large intra-class differences, and imbalanced distribution among classes. Convolutional Neural Network (CNN) based models have been widely used in remote sensing image segmentation tasks for its powerful feature extraction capability. Due to intrinsic locality of CNN architectures, it is difficult to understand the long-range dependencies among image patches. Recently, the transformer leverages long-range dependencies and performs well in computer vision tasks. To take advantages of both CNN and Transformer, a novel Adaptive Enhanced Swin Transformer with U-Net (AESwin-UNet) is proposed for remote sensing segmentation. AESwinUNet uses a hybrid Transformer-based U-type Encoder-Decoder architecture with skip connections to extract local and global semantic features. Specifically, the Enhanced Swin Transformer (E-Swin Transformer) contains Enhanced Multi-head Self-Attention and Deformable Adaptive Patch Merging layer in encoder. A symmetric cascaded decoder is designed for up-sampling to obtain higher resolution feature maps. Experiments on two public benchmark datasets, WHDLD and LoveDA, demonstrate that the proposed AESwin-UNet performs well in semantic segmentation.
更多查看译文
关键词
Remote sensing,Semantic segmentation,Unet,Transformer,CNN
AI 理解论文
溯源树
样例
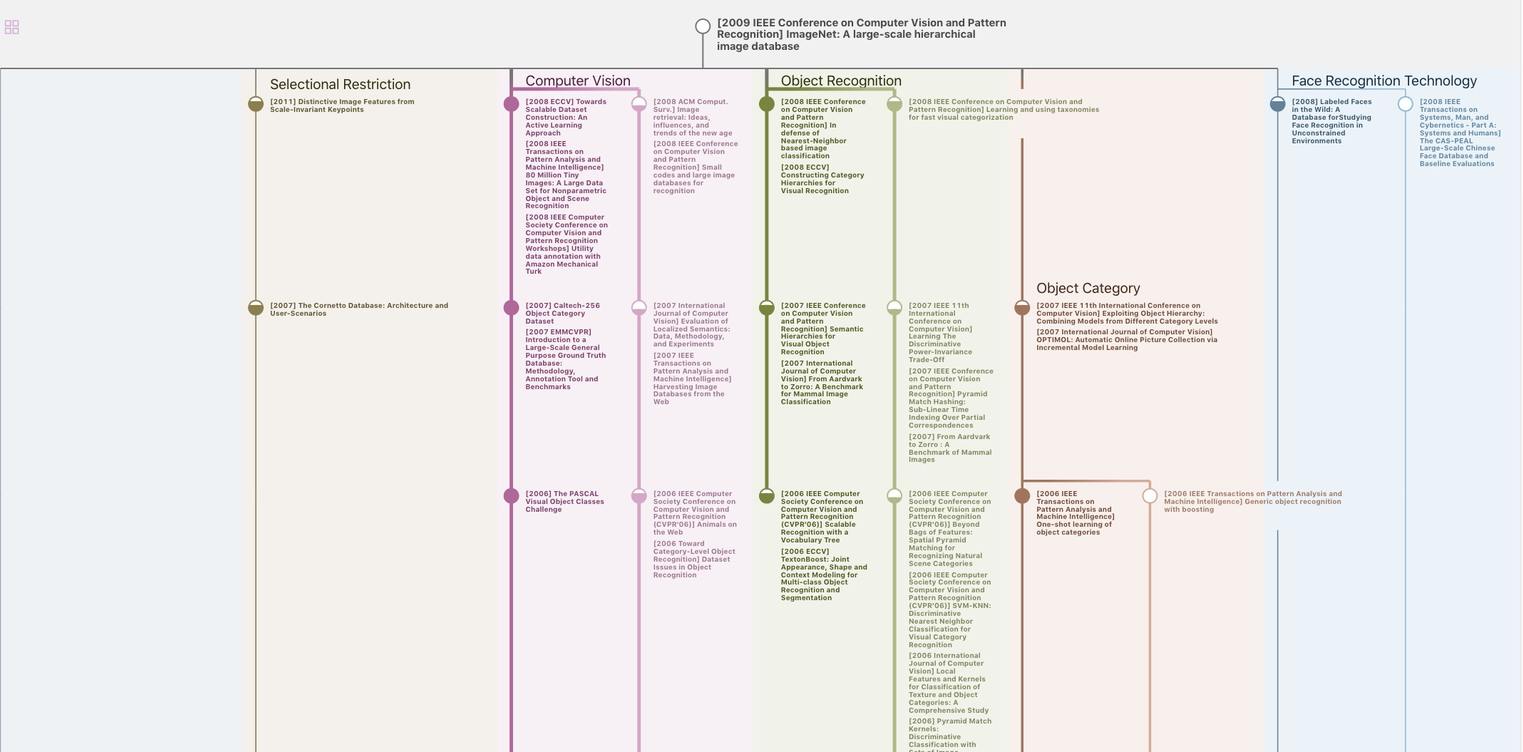
生成溯源树,研究论文发展脉络
Chat Paper
正在生成论文摘要