MsmcNet: A Modular Few-Shot Learning Framework for Signal Modulation Classification
IEEE TRANSACTIONS ON SIGNAL PROCESSING(2022)
摘要
With the development of deep learning (DL) technology, the feature extraction capability of networks has gradually been enhanced, and high accuracy has been achieved in signal modulation classification (SMC) tasks. DL requires numerous training samples to achieve high classification accuracy. However, in non-cooperative cases, only a few labeled data are usually available. To solve this problem, we propose a modular few-shot learning framework for SMC, called MsmcNet. MsmcNet comprises an IQ fusion (IQF) module, 1D signal feature processing (1D-SFP) modules, and a classifier. The number of the 1D-SFP modules is variable and is determined by a GCN-based method. Experimental results on RadioML2016.04c and RadioML2016.10a show that the proposed approach outperforms the baselines in all aspects. In particular, for the RadioML2016.10a dataset, when the SNR is 6 dB and the training data account for more than 2% of the test data, the classification accuracy of MsmcNet is greater than 70%. To further meet the requirements in the non-cooperative case, we explore the performance of the proposed MsmcNet in the one-shot learning case and find that it maintains its high performance advantage. In addition to the SMC task, we explore the performance of MsmcNet in the transmitter identification task to verify the universality of the framework. Compared to the baselines, MsmcNet achieves outstanding results.
更多查看译文
关键词
Modulation, Task analysis, Feature extraction, Convolution, Neural networks, Deep learning, Radio transmitters, Deep learning, few-shot learning, one-shot learning, signal modulation classification, transmitter identification
AI 理解论文
溯源树
样例
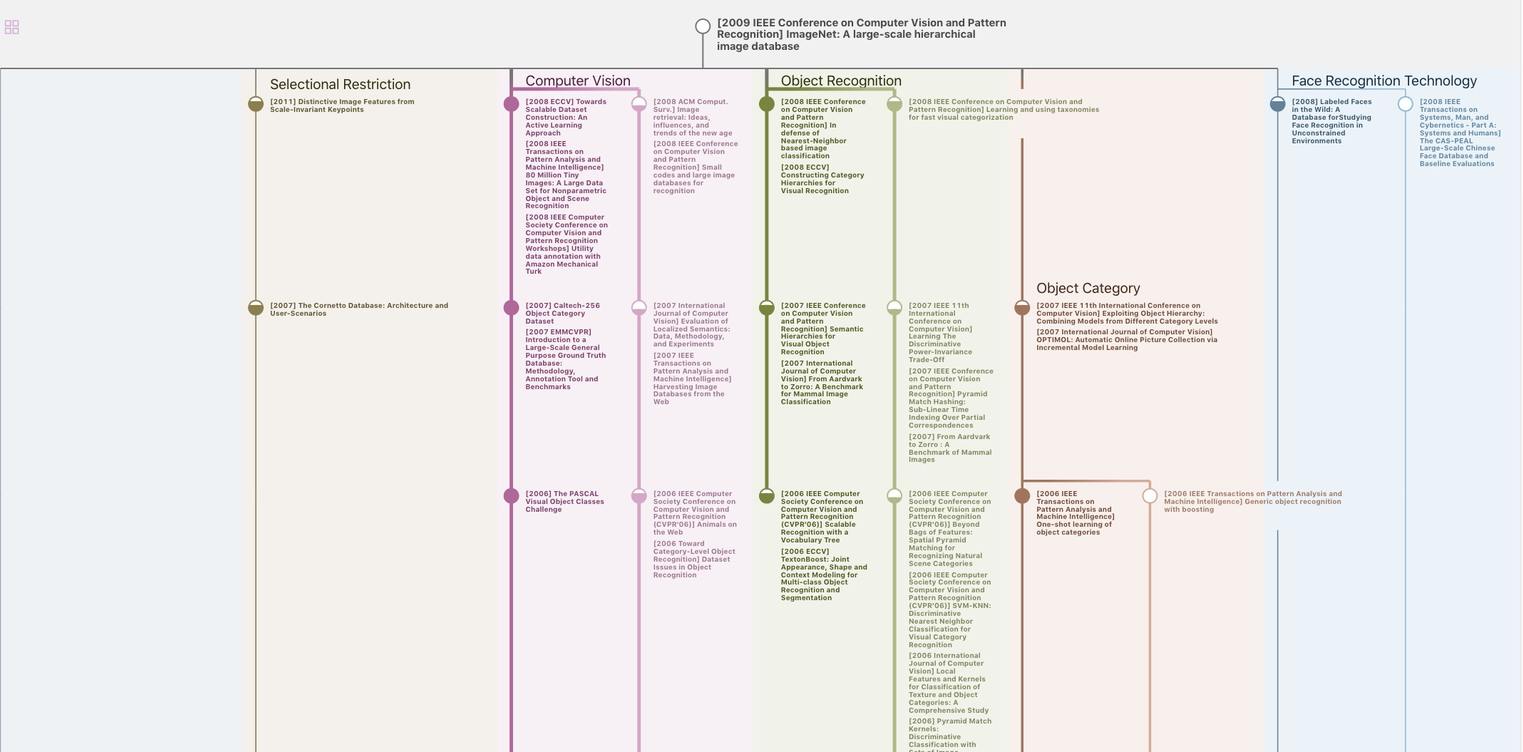
生成溯源树,研究论文发展脉络
Chat Paper
正在生成论文摘要