EARS: efficiency-aware russian roulette and splitting
ACM Transactions on Graphics(2022)
摘要
ussian roulette and splitting are widely used techniques to increase the efficiency of Monte Carlo estimators. But, despite their popularity, there is little work on how to best apply them. Most existing approaches rely on simple heuristics based on, e.g., surface albedo and roughness. Their efficiency often hinges on user-controlled parameters. We instead iteratively learn optimal Russian roulette and splitting factors during rendering, using a simple and lightweight data structure. Given perfect estimates of variance and cost, our fixed-point iteration provably converges to the optimal Russian roulette and splitting factors that maximize the rendering efficiency. In our application to unidirectional path tracing, we achieve consistent and significant speed-ups over the state of the art.
更多查看译文
关键词
global illumination, importance sampling, path guiding
AI 理解论文
溯源树
样例
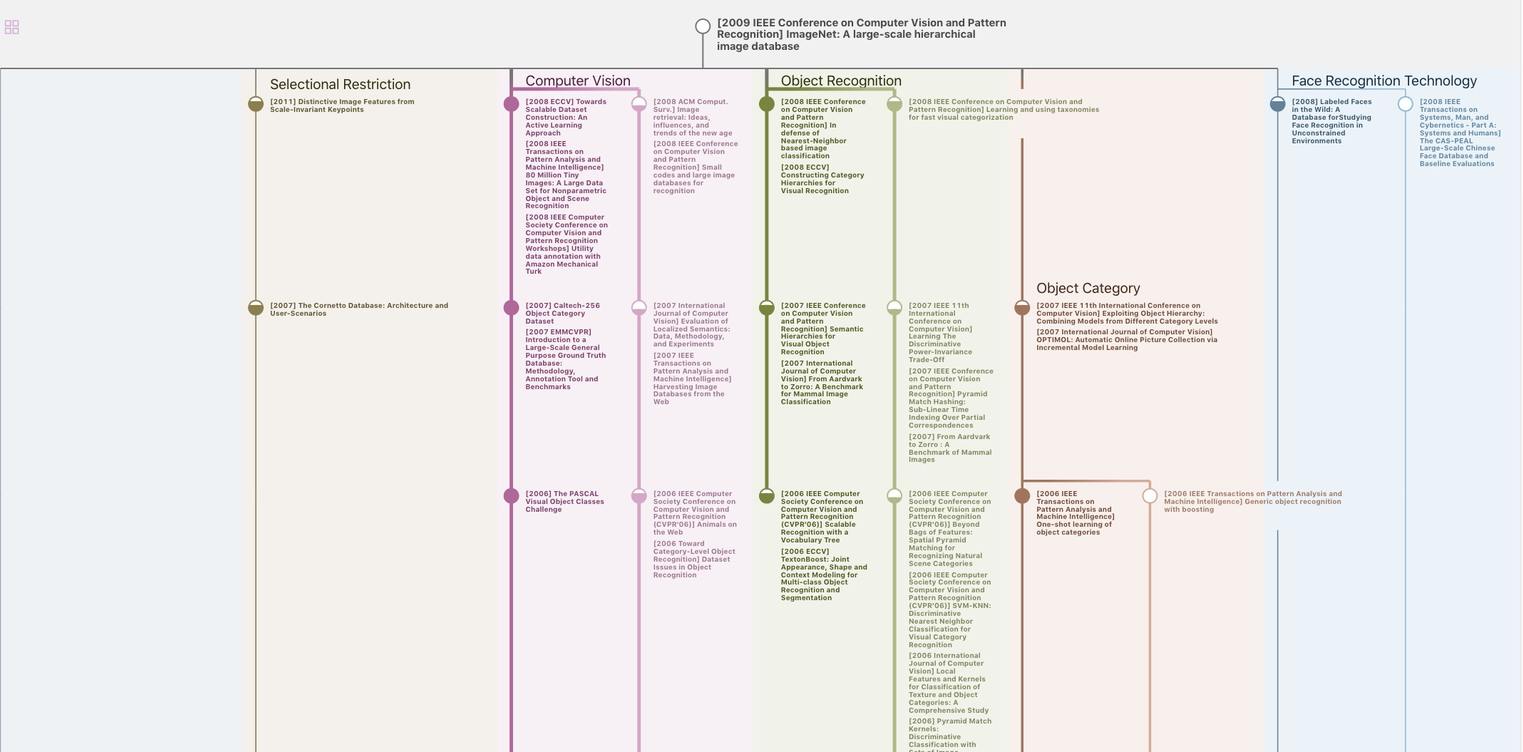
生成溯源树,研究论文发展脉络
Chat Paper
正在生成论文摘要