Transformer-based attention network for stock movement prediction
Expert Systems with Applications(2022)
摘要
Stock movement prediction is an important field of study that can help market traders make better trading decisions and earn more profit. The fusion of text from social media platforms such as Twitter and actual stock prices is an effective but difficult approach for stock movement prediction. Although some previous methods have explored this approach, there are still difficulties with the temporal dependence of financial data and insufficient effectiveness of fusing text and stock prices. To solve these problems, we propose the novel Transformer Encoder-based Attention Network (TEANet) framework, which is based on precise description through small-sample feature engineering and uses a small sample of 5 calendar days to capture the temporal dependence of financial data. In addition, this deep learning framework uses the Transformer model and multiple attention mechanisms to achieve feature extraction and effective analysis of financial data to achieve accurate prediction. Extensive experiments on four datasets demonstrate the effectiveness of our framework. Further simulations show that an actual trading strategy based on our proposed model can significantly increase profit and has practical application value.
更多查看译文
关键词
Stock movement prediction,Deep learning,Transformer,Attention
AI 理解论文
溯源树
样例
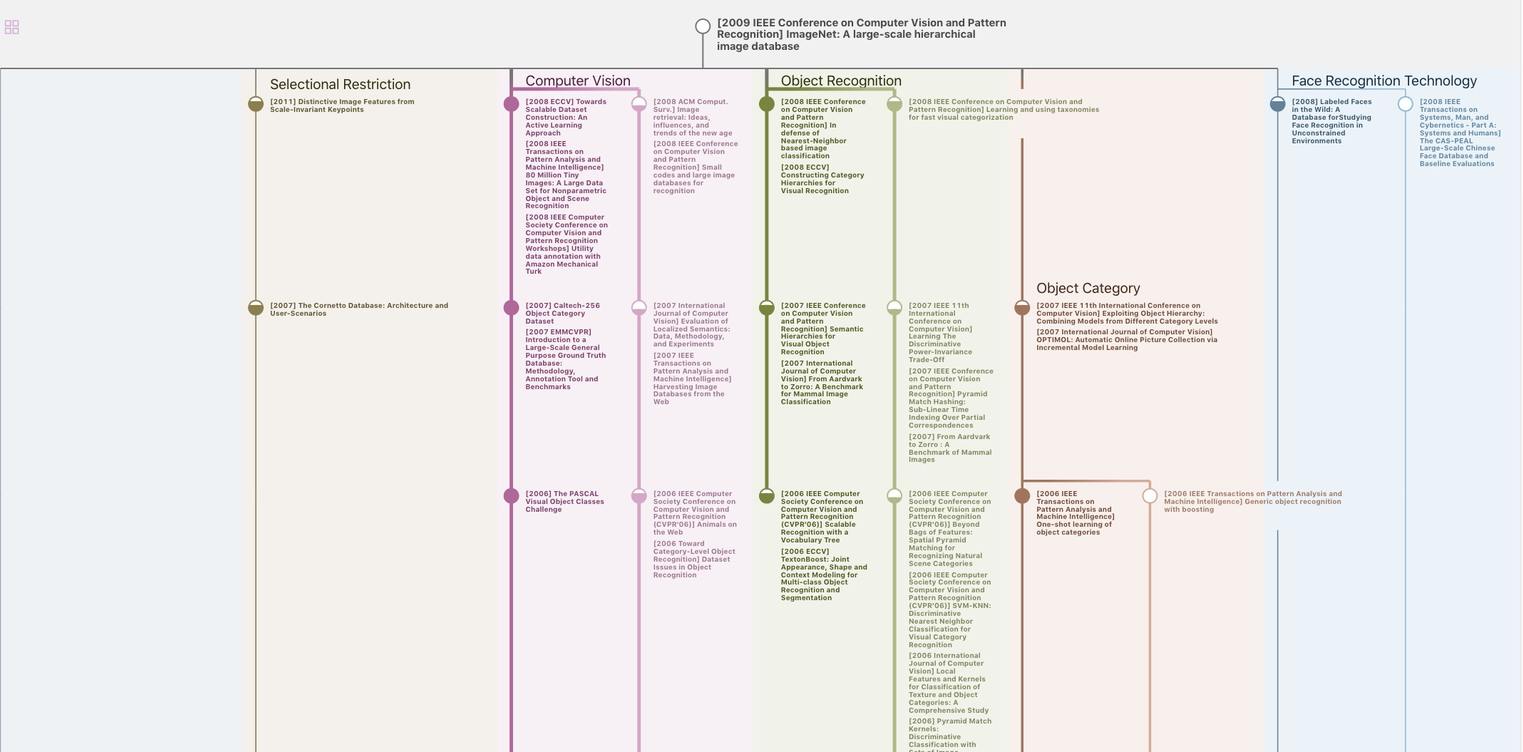
生成溯源树,研究论文发展脉络
Chat Paper
正在生成论文摘要