Accurate Extraction of Ground Objects from Remote Sensing Image Based on Mark Clustering Point Process
ISPRS INTERNATIONAL JOURNAL OF GEO-INFORMATION(2022)
Abstract
The geometric features of ground objects can reflect the shape, contour, length, width, and pixel distribution of ground objects and have important applications in the process of object detection and recognition. However, the geometric features of objects usually present irregular geometric shapes. In order to fit the irregular geometry accurately, this paper proposes the mark clustering point process. Firstly, the random points in the parent process are used to determine the location of the ground object, and the irregular graph constructed by the clustering points in the sub-process is used as the identification to fit the geometry of the ground object. Secondly, assuming that the spectral measurement values of ground objects obey the independent and unified multivalued Gaussian distribution, the spectral measurement model of remote sensing image data is constructed. Then, the geometric extraction model of the ground object is constructed under the framework of Bayesian theory and combined with the reversible jump Markov chain Monte Carlo (RJMCMC) algorithm to simulate the posterior distribution and estimate the parameters. Finally, the optimal object extraction model is solved according to the maximum a posteriori (MAP) probability criterion. This paper experiments on color remote sensing images. The experimental results show that the proposed method can not only determine the position of the object but also fit the geometric features of the object accurately.
MoreTranslated text
Key words
mark clustering point process, object extraction, Bayesian theory, reversible jump Markov chain Monte Carlo (RJMCMC)
AI Read Science
Must-Reading Tree
Example
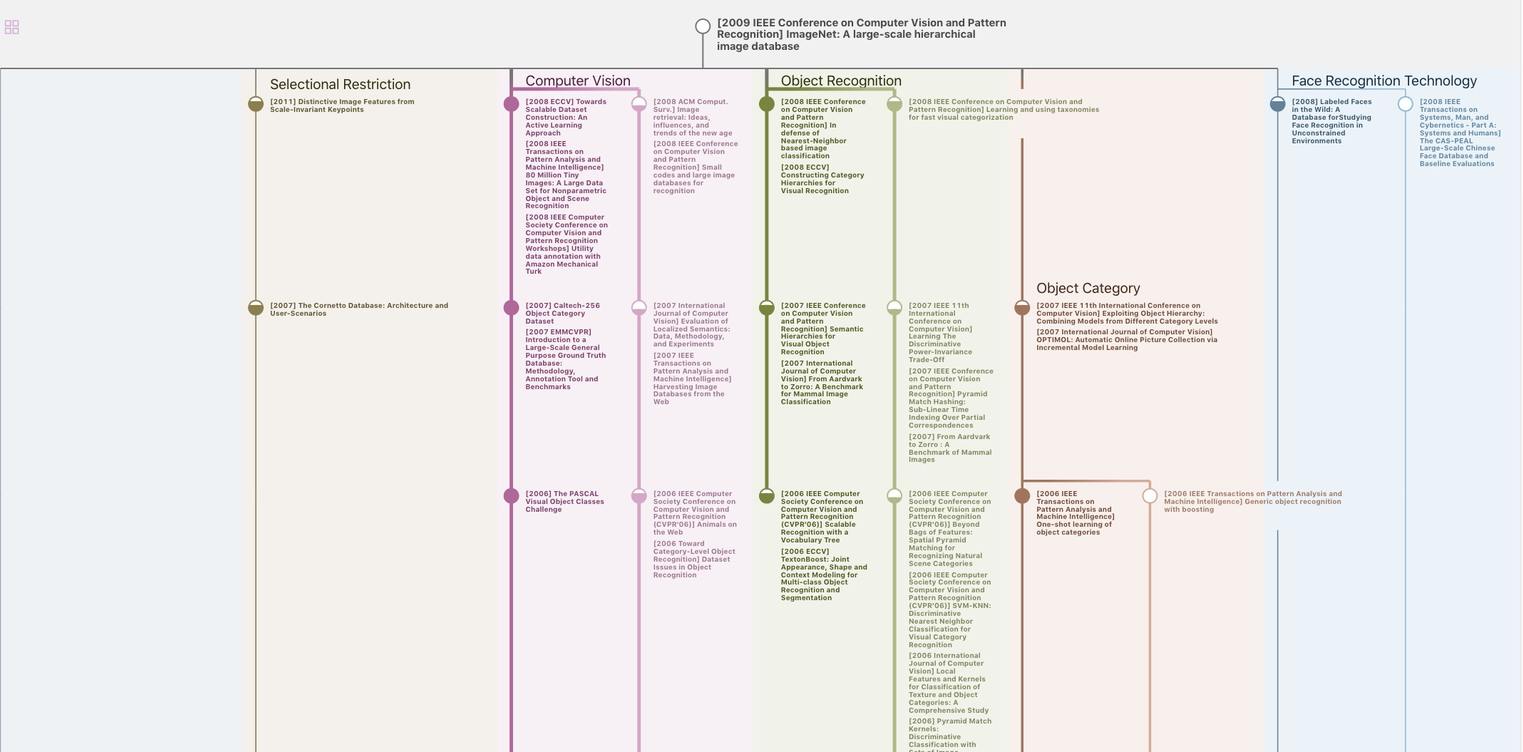
Generate MRT to find the research sequence of this paper
Chat Paper
Summary is being generated by the instructions you defined