Extraction of Continuous and Discrete Spatial Heterogeneities: Fusion Model of Spatially Varying Coefficient Model and Sparse Modelling
ISPRS INTERNATIONAL JOURNAL OF GEO-INFORMATION(2022)
摘要
Geospatial phenomena often have spatial heterogeneity, which is caused by differences in the data generation process from place to place. There are two types of spatial heterogeneity: continuous and discrete, and there has been much discussion about how to analyze one type of spatial heterogeneity. Although geospatial phenomena can have both types of spatial heterogeneities, previous studies have not sufficiently discussed how to consider these two different types of spatial heterogeneity simultaneously and how to detect them separately, which may lead to biased estimates and the wrong interpretation of geospatial phenomena. This study proposes a new approach for the analysis of spatial data with both heterogeneities by combining the eigenvector spatial filtering-based spatially varying coefficient (ESF-SVC) model, which assumes the continuous spatial heterogeneity and generalized lasso (GL) estimation, which assumes discrete spatial heterogeneity and proposes the ESF-GL-SVC model. The performance of ESF-GL-SVC was evaluated through experiments based on a Monte Carlo simulation and confirms that the ESF-GL-SVC showed better performance in estimating coefficients with both types of spatial heterogeneity than the previous two models. The application of the apartment rent data showed that the ESF-GL-SVC outputs the result with the smallest BIC value, and the estimated coefficients depict continuous and discrete spatial heterogeneity in the dataset. Reasonable coefficients were estimated using the ESF-GL-SVC, although some coefficients by ESF-SVC were not.
更多查看译文
关键词
spatial heterogeneity, eigenvector spatial filtering-based spatially varying coefficient model, generalized lasso
AI 理解论文
溯源树
样例
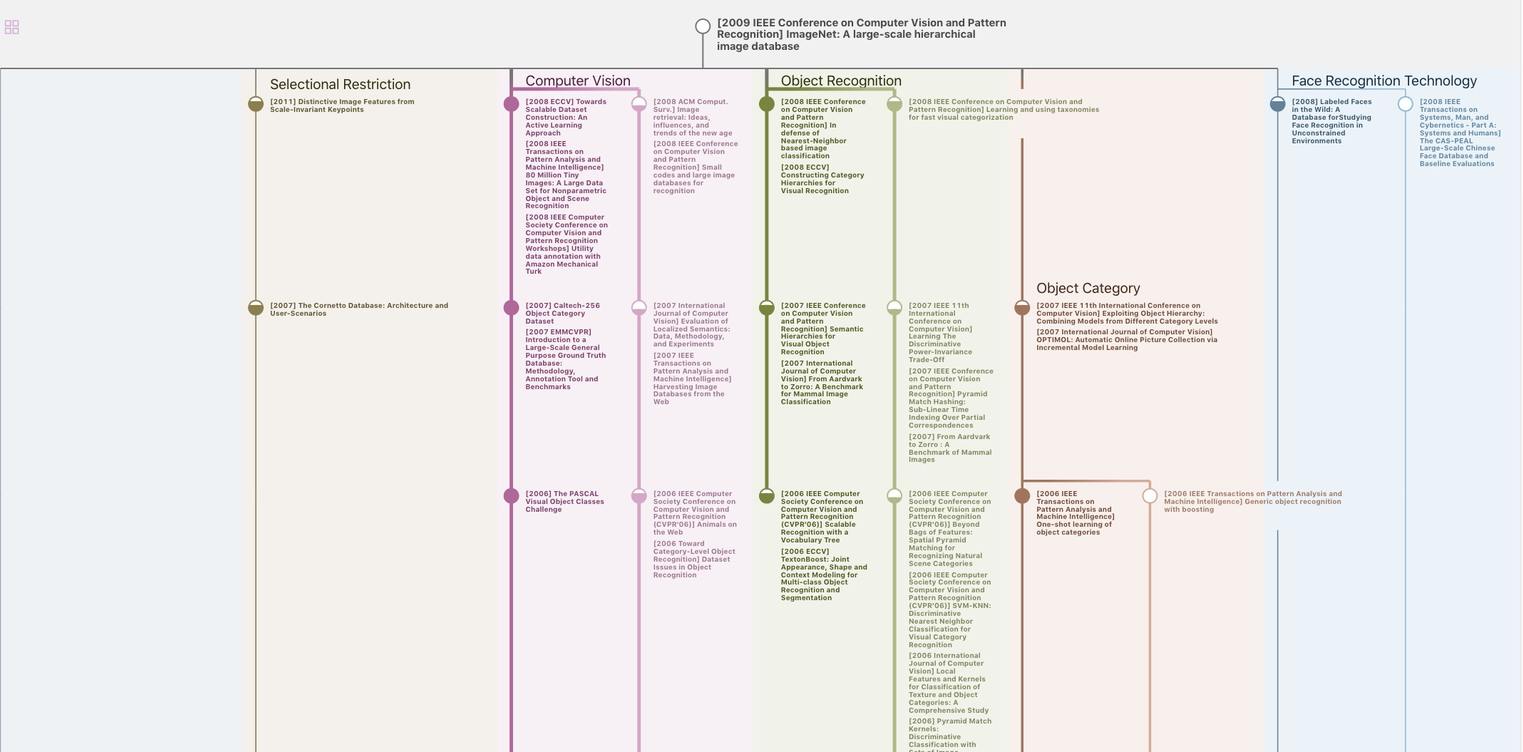
生成溯源树,研究论文发展脉络
Chat Paper
正在生成论文摘要