Survival Regression with Proper Scoring Rules and Monotonic Neural Networks
INTERNATIONAL CONFERENCE ON ARTIFICIAL INTELLIGENCE AND STATISTICS, VOL 151(2022)
摘要
We consider frequently used scoring rules for right-censored survival regression models such as time-dependent concordance, survival-CRPS, integrated Brier score and integrated binomial log-likelihood, and prove that neither of them is a proper scoring rule. This means that the true survival distribution may be scored worse than incorrect distributions, leading to inaccurate estimation. We prove that, in contrast to these scores, the right-censored log-likelihood is a proper scoring rule, i.e., the highest expected score is achieved by the true distribution. Despite this, modern feed-forward neural-network-based survival regression models are unable to train and validate directly on the right-censored log-likelihood, due to its intractability, and resort to the aforementioned alternatives, i.e., non-proper scoring rules. We therefore propose a simple novel survival regression method capable of directly optimizing log-likelihood using a monotonic restriction on the time-dependent weights, coined SurvivalMonotonic-net (SuMo-net). SuMo-net achieves state-of-the-art log-likelihood scores across several datasets with 20-100x computational speedup on inference over existing state-of-the-art neural methods, and is readily applicable to datasets with several million observations.
更多查看译文
关键词
survival,monotonic neural
AI 理解论文
溯源树
样例
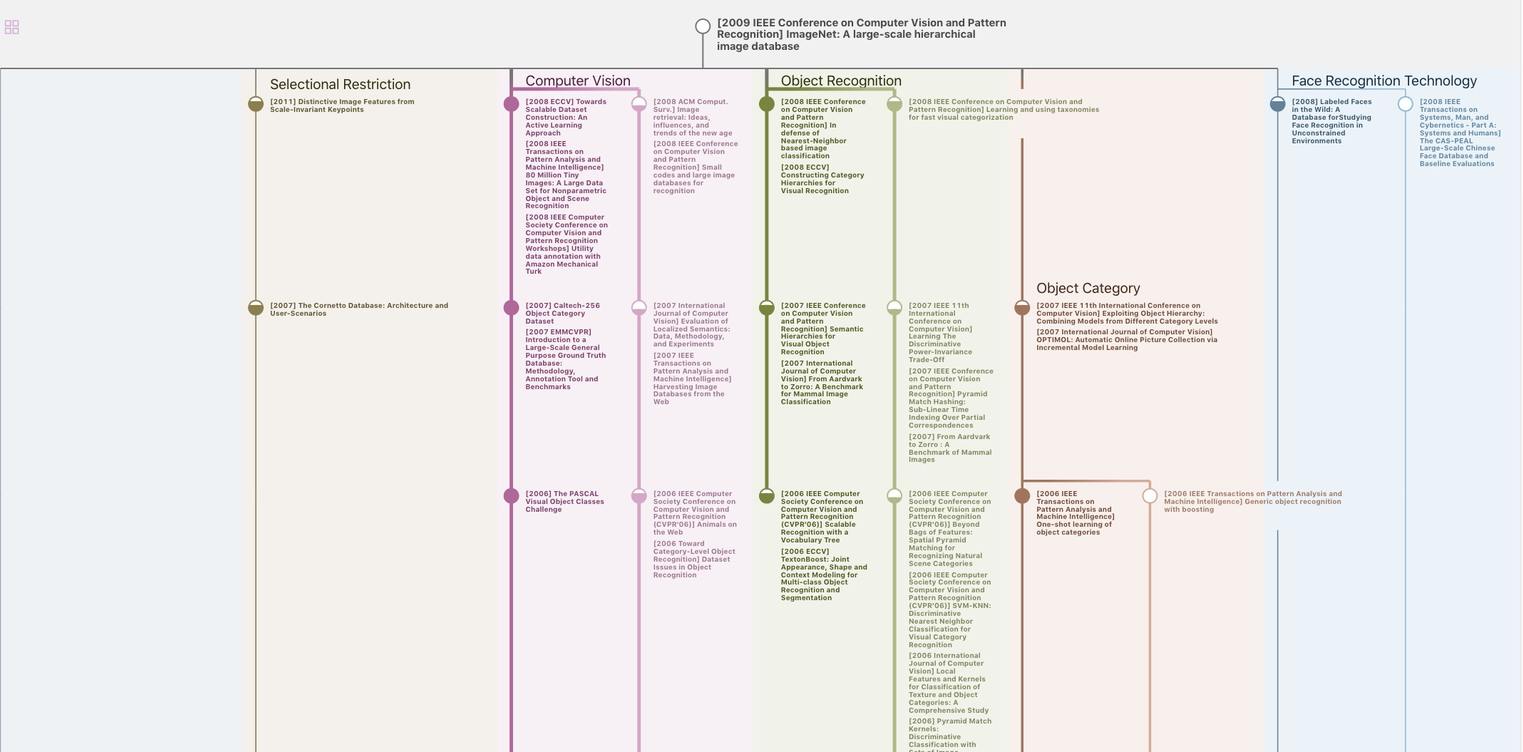
生成溯源树,研究论文发展脉络
Chat Paper
正在生成论文摘要