Improving Knowledge Distillation With a Customized Teacher
IEEE TRANSACTIONS ON NEURAL NETWORKS AND LEARNING SYSTEMS(2024)
摘要
Knowledge distillation (KD) is a widely used approach to transfer knowledge from a cumbersome network (also known as a teacher) to a lightweight network (also known as a student). However, even though the accuracies of different teachers are similar, the fixed student's accuracies are significantly different. We find that teachers with more dispersed secondary soft probabilities are more qualified to play their roles. Therefore, an indicator, i.e., the standard deviation sigma of secondary soft probabilities, is introduced to choose the teacher. Moreover, to make a teacher's secondary soft probabilities more dispersed, a novel method, dubbed pretraining the teacher under dual supervision (PTDS), is proposed to pretrain a teacher under dual supervision. In addition, we put forward an asymmetrical transformation function (ATF) to further enhance the dispersion degree of the pretrained teachers' secondary soft probabilities. The combination of PTDS and ATF is termed knowledge distillation with a customized teacher (KDCT). Extensive empirical experiments and analyses are conducted on three computer vision tasks, including image classification, transfer learning, and semantic segmentation, to substantiate the effectiveness of KDCT.
更多查看译文
关键词
Knowledge distillation (KD),knowledge transfer,neural network acceleration,neural network compression
AI 理解论文
溯源树
样例
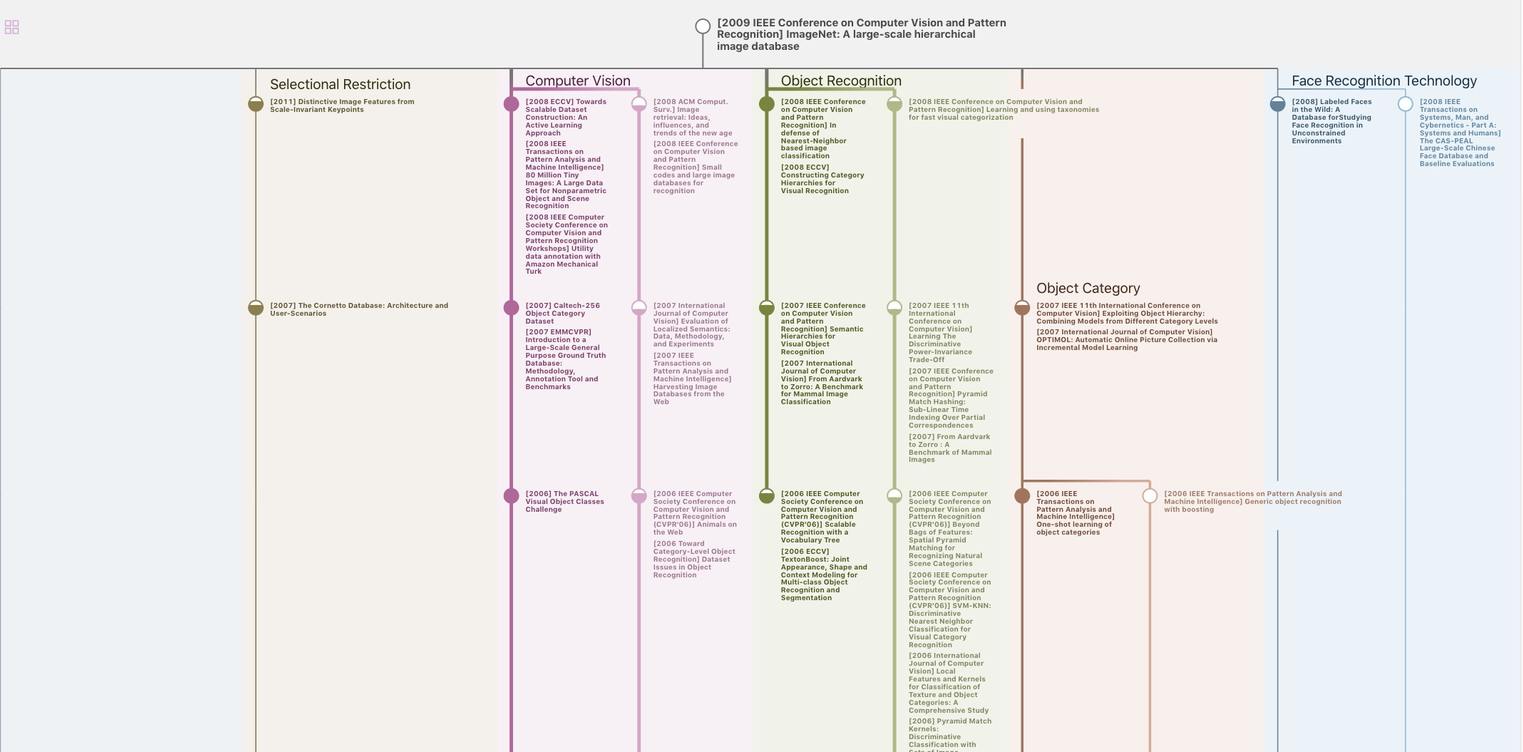
生成溯源树,研究论文发展脉络
Chat Paper
正在生成论文摘要