Deep Image Clustering for Standardization of Radiological Workflows
MEDICAL IMAGING 2022: IMAGE-GUIDED PROCEDURES, ROBOTIC INTERVENTIONS, AND MODELING(2022)
摘要
Artificial Intelligence (AI) has revolutionized medical imaging by producing state-of-the-art results in various tasks with performance comparable to human readers. However, AI models are often task- and data-specific, requiring input images with specific modalities and sequences. Although DICOM metadata can provide such information about the data, it is inconsistent and with many missing values in different fields of metadata. In this paper, we present a deep learning-based unsupervised clustering algorithm to group radiological images solely based on their pixel data. We start by extracting semantically meaningful features through a representation learning approach, and we calculate the nearest neighbors of the images. Next, the image and its neighbors are used as inputs for training an unsupervised clustering algorithm. A human reader then labels each of the clusters by examining the modality, body part, and orientation. At the test time, after using the trained clustering model to identify the group the image belongs to, we can assign consistent and correct metadata labels. Experimental evaluation shows that we can successfully cluster images with 90% accuracy without using any labeled data. We envision our approach to be utilized as a pre-processing step for a variety of tasks that require determining the underlying nature of the data in the DICOM file, even if the metadata is incorrectly formatted.
更多查看译文
关键词
Deep Learning,Representation Learning,Clustering,DICOM
AI 理解论文
溯源树
样例
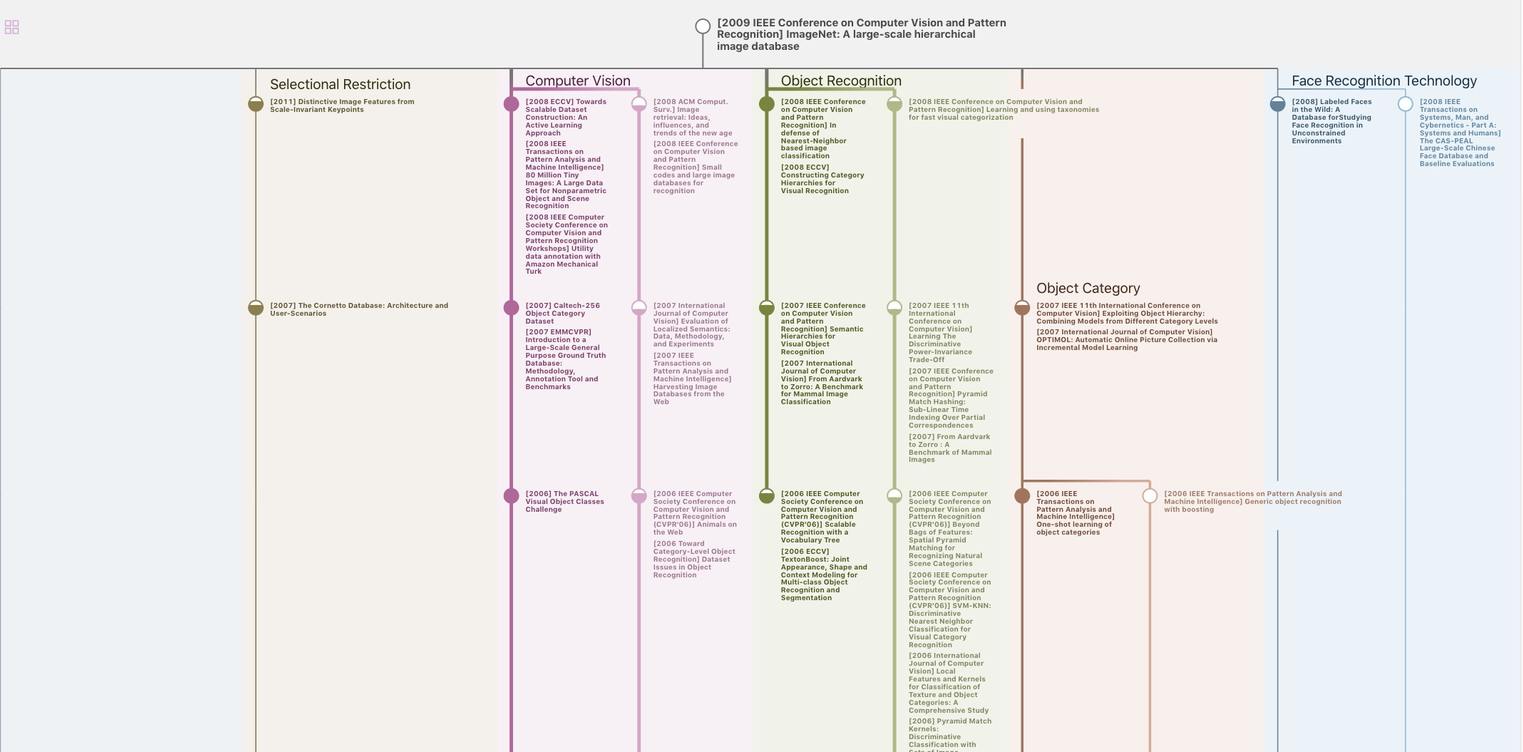
生成溯源树,研究论文发展脉络
Chat Paper
正在生成论文摘要