Estimating chemical concentrations from compressed hyperspectral images
ALGORITHMS, TECHNOLOGIES, AND APPLICATIONS FOR MULTISPECTRAL AND HYPERSPECTRAL IMAGING XXVIII(2022)
摘要
In this paper we derive two algorithms for estimating concentrations of a known chemical compound from compressed measurements of a hyperspectral image (HSI). It is assumed that each resolved pixel in a scene contains a chemical of known spectral signature, at an unknown concentration. The problem is to estimate the concentration directly from the compressed measurements. Estimated concentrations are either displayed or used as detection scores in a threshold test for presence or absence of chemical. In the first algorithm we use matched filtering and l(1) regularization to extract an image of concentrations, directly from compressed data. In the second we model the image of concentrations in a fixed-resolution subspace of the 2D Haar wavelet domain, estimate its parameters in this space, and reconstruct the image of concentrations at a macro-pixel resolution. We evaluate our algorithms by applying them to several long-wave infrared (LWIR) HSI data sets, either synthetically generated or recorded by Physical Sciences Inc. Synthetically-generated data is compressed with a mathematically-defined linear compressor; real HSI data is compressed with PSI's Digital Micromirror Device (DMD), which is a physical implementation of a mathematically-defined compressor; Fabry-Perot data is raw HSI data recorded by PSI, which is then compressed with a mathematically-defined compressor. We demonstrate for these data sets that estimating concentrations through matched filtering and l(1) inversion of compressed measurements yields detection performance that is as good as previously proposed methods that first reconstruct a hyperspectral data cube from compressed data, and then estimate or detect chemical concentrations. The proposed methods save on memory and computation. We demonstrate that detection performance is maintained when resolving concentration maps at a lower resolution, so long as the resolution is not too low.
更多查看译文
关键词
Compressed sensing, Hyperspectral Imaging, Detection, Multi-resolution, Haar wavelets, Walsh-Hadamard, Transform Space, MVDR, ACE, LWIR
AI 理解论文
溯源树
样例
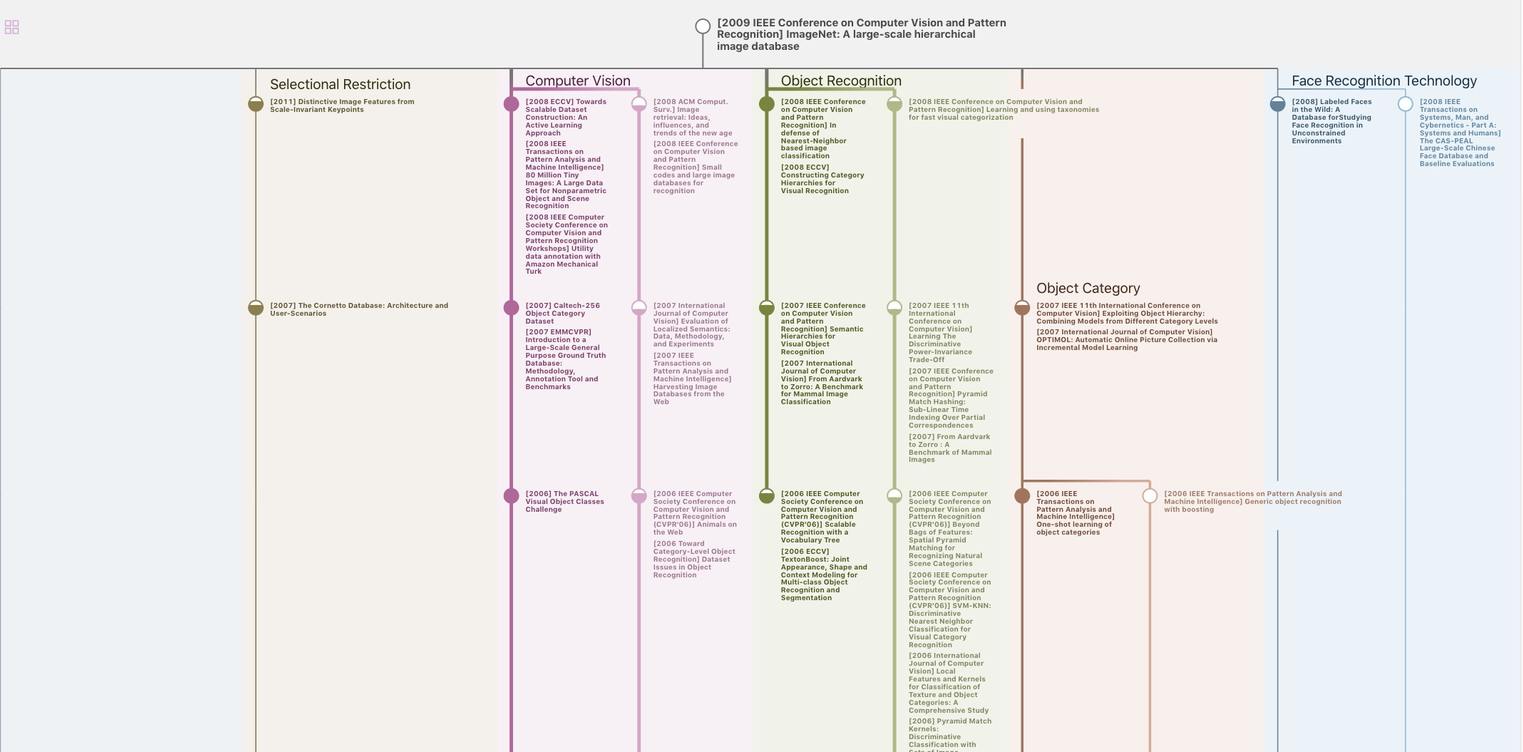
生成溯源树,研究论文发展脉络
Chat Paper
正在生成论文摘要