An Autonomous Storage Optimization LRM Generation Strategy Based on Cascaded NURBS
SYMMETRY-BASEL(2022)
摘要
Automatic control of intelligent vehicles depends heavily on accurate lane-level location information. The traditional digital map cannot meet the automatic driving requirements, and it is very difficult for lane-level road maps (LRMs) to achieve a good balance between the representation accuracy and data storage volume. To tackle this problem, we obtain the recursive definition of a lane line by analyzing road lanes using the fractal geometry theory. Subsequently, we construct a recursive feedback system and model it. On this basis, an autonomous storage optimization LRM generation strategy based on cascaded NURBS is devised. According to the input data density, this framework generates an LRM with the minimum data storage volume within the error constraint. It is mainly composed of two modules: (1) a random fractal and (2) an adaptive iteration memory optimizer, based on cascaded NURBS error feedback. First, the whole lane is divided into several meta lane line modules. Second, data sampling/interpolation is carried out iteratively according to the NURBS fitting error and symmetry of road geometry. In this way, the storage space used to store lane lines and other information is automatically optimized. Last, a simulation experiment is carried out by using a dataset obtained from a park. The simulation results show that the proposed method can achieve high accuracy and high storage efficiency. Under the constraint of a 0.1 m curve fitting error, the average memory demand of the lane line elements is less than 2.4 bytes/m.
更多查看译文
关键词
lane-level road map, Non-Uniform Rational B-spline (NURBS), accuracy, memory efficiency
AI 理解论文
溯源树
样例
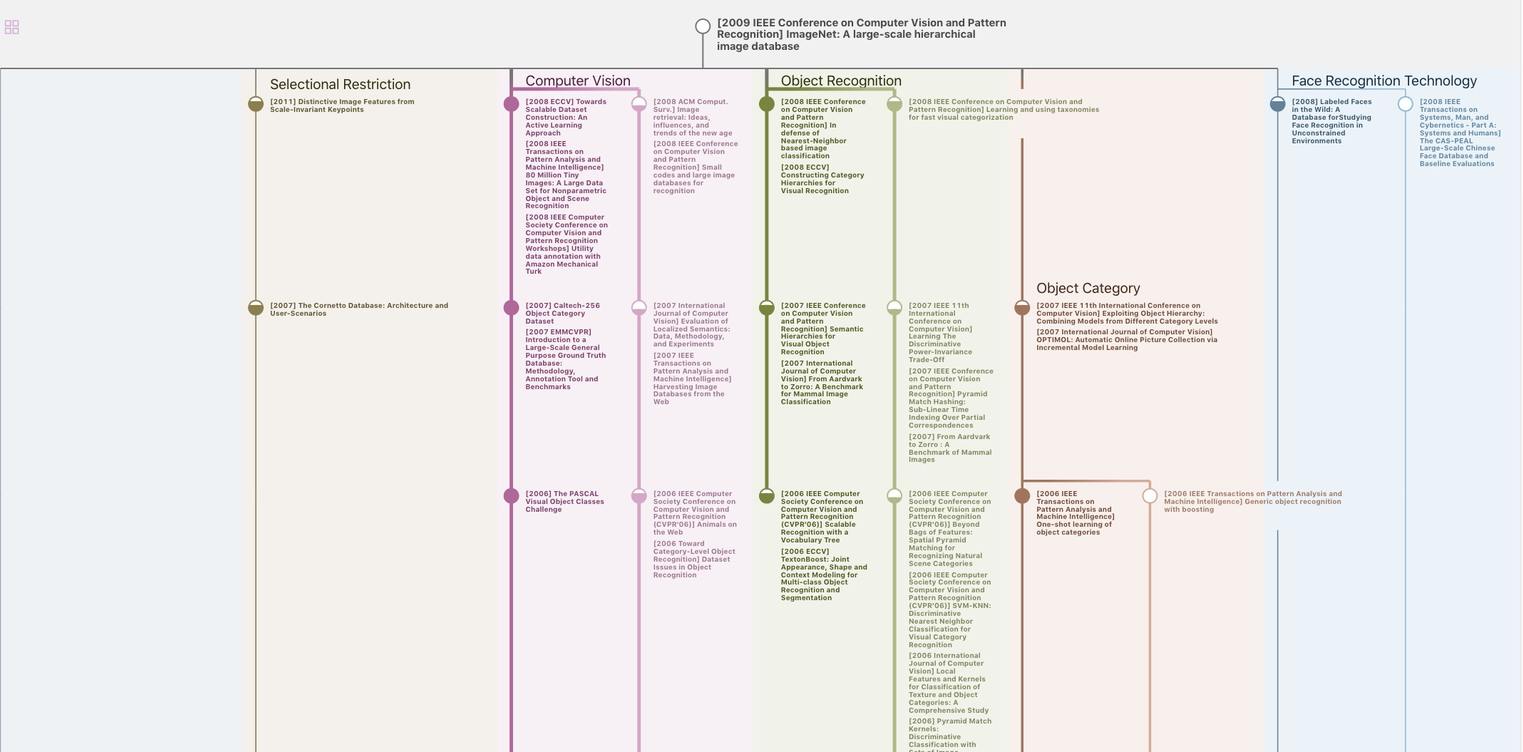
生成溯源树,研究论文发展脉络
Chat Paper
正在生成论文摘要