Phase-resolved Real-Time Ocean Wave Prediction with Quantified Uncertainty Based on Variational Bayesian Machine Learning
Applied energy(2022)
摘要
Phase-resolved wave prediction is of vital importance for the real-time control of wave energy converters. In this paper, a novel wave prediction method is proposed, which, to the authors’ knowledge, achieves the real-time nonlinear wave prediction with quantified uncertainty (including both aleatory and model uncertainties) for the first time. Moreover, the proposed method achieves the prediction of the predictable zone without assuming linear sea states, while all previous works on predictable zone determination were based on linear wave theory (which produces overly conservative estimations). The proposed method is developed based on the Bayesian machine learning approach, which can take advantage of machine learning model’s ability in tackling complex nonlinear problems, while taking various forms of uncertainties into account via the Bayesian framework. A set of wave tank experiments are carried out for evaluation of the method. The results show that the wave elevations at the location of interest are predicted accurately based on the measurements at sensor location, and the prediction uncertainty and its variations across the time horizon are well captured. The comparison with other wave prediction methods shows that the proposed method outperforms them in terms of both prediction accuracy and the length of the predictable zone. Particularly, for short-term wave forecasting, the prediction error by the proposed method is as much as 55.4% and 11.7% lower than the linear wave theory and deterministic machine learning approaches, and the predictable zone is expanded by the proposed method by as much as 74.6%.
更多查看译文
关键词
Bayesian neural network,Deterministic sea wave prediction,Predictable zone,Probabilistic machine learning,Uncertainty quantification,Wave tank experiment
AI 理解论文
溯源树
样例
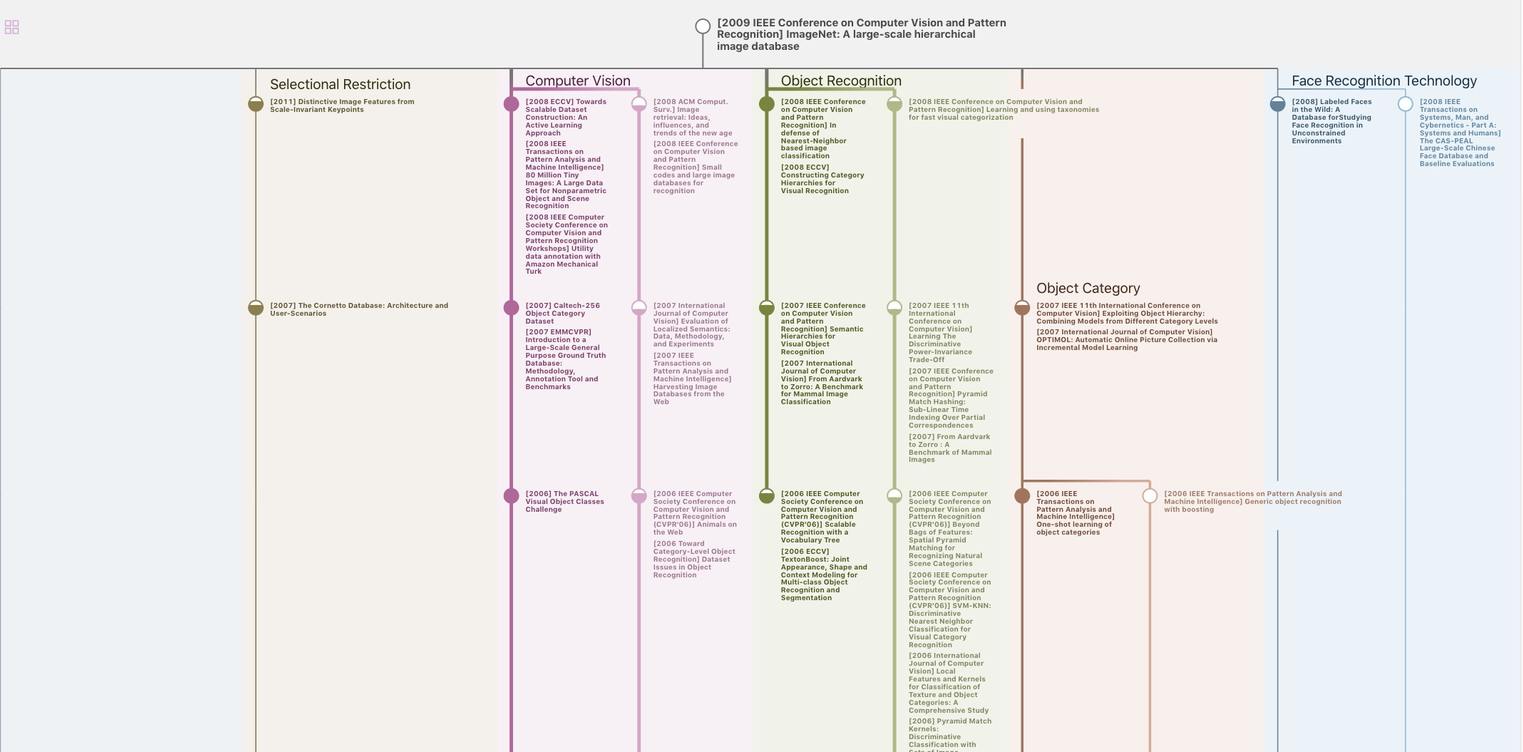
生成溯源树,研究论文发展脉络
Chat Paper
正在生成论文摘要