Multi‐hazard Typhoon and Earthquake Collapse Fragility Models for Transmission Towers: an Active Learning Reliability Approach Using Gradient Boosting Classifiers
Earthquake engineering and structural dynamics/Earthquake engineering & structural dynamics(2022)
摘要
Natural hazards such as earthquakes and typhoons pose a considerable threat to the power transmission grid. In regions prone to both hazards, the overhead transmission infrastructure may experience multi-hazard effects where two consecutive hazards can occur within an interval shorter than the time that would be needed to repair the system for damages occurred in the first event. This consequently increases the susceptibility to damage during the subsequent hazard. In this context, this paper presents the first set of multi-hazard fragility models for typhoons and earthquakes. The computational cost of numerical simulations for multi-hazard analyses is high due to the complex nonlinear behavior of transmission towers, numerous sources of material and geometric uncertainty, and the multifaceted effects of multi-hazard events. To tackle this challenge, we introduce a novel active learning approach for the probabilistic classification of physics-based collapse data. An initial pool of labeled collapse data is generated by performing a limited number of nonlinear time history analyses on random realizations of a high-fidelity finite element model of a common double circuit tower. A machine learning-based extreme gradient boosting (XGBoost) classification model is trained via the query by committee (QBC) for the task of predicting the probability of belonging to the class of collapse (i.e., probability of collapse). Active learning is established by leveraging the disagreement information between the learners and the query points are sequentially labeled and added to the initial pool of collapse/survival data. Using this approach, multi-hazard typhoon and earthquake collapse fragility surfaces of transmission towers in different directions and sequences of hazards are developed. Results point to the significance of multi-hazard effects on the fragility of transmission structures. They also reveal the efficacy of the introduced active learning approach in reducing the computational complexity of multi-hazard fragility assessments. The fragility models developed in this study constitute a key step toward multi-hazard resilience assessment and optimal management of the power transmission grid.
更多查看译文
关键词
active learning,earthquake,fragility analysis,gradient boosting,transmission towers,typhoon
AI 理解论文
溯源树
样例
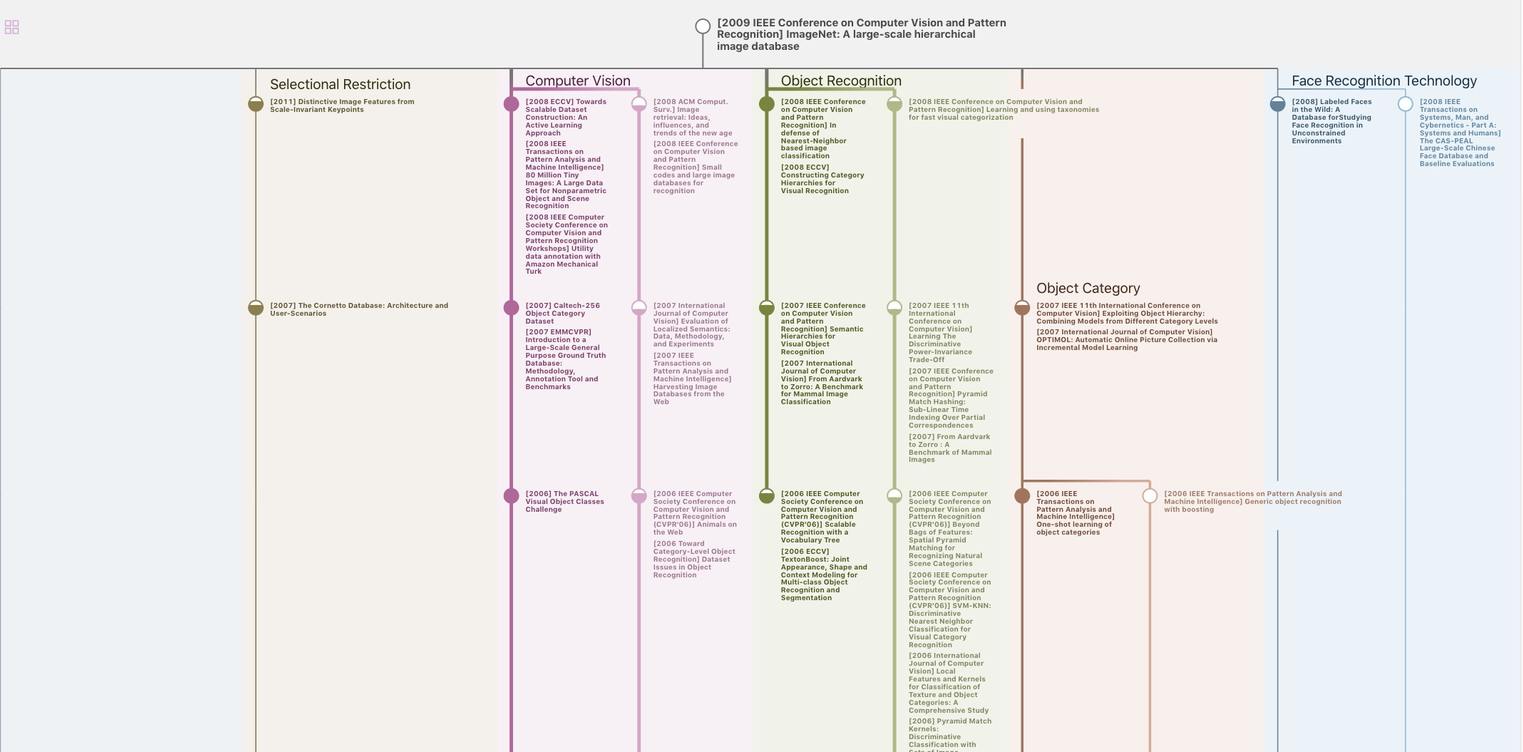
生成溯源树,研究论文发展脉络
Chat Paper
正在生成论文摘要