A statistical reference-free genomic algorithm subsumes common workflows and enables novel discovery
biorxiv(2022)
摘要
We introduce a probabilistic model that enables study of myriad, disparate and fundamental problems in genome science and expands the scope of inference currently possible. Our model formulates an unrecognized unifying goal of many biological studies – to discover sample-specific sequence diversification – and subsumes many application-specific models. With it, we develop a novel algorithm, NOMAD, that performs valid statistical inference on raw reads, completely bypassing references and sample metadata. NOMAD’s reference-free approach enables data-scientifically driven discovery with previously unattainable generality, illustrated with de novo prediction of adaptation in SARS-CoV-2, novel single-cell resolved, cell-type-specific isoform expression, including in the major histocompatibility complex, and de novo identification of V(D)J recombination. NOMAD is a unifying, provably valid and highly efficient algorithmic solution that enables expansive discovery.
One-Sentence Summary We present a unifying formulation of disparate genomic problems and design an efficient, reference-free solution.
### Competing Interest Statement
K.C., T.Z.B., and J.S. are inventors on provisional patents related to this work. The authors declare no other competing interests.
更多查看译文
AI 理解论文
溯源树
样例
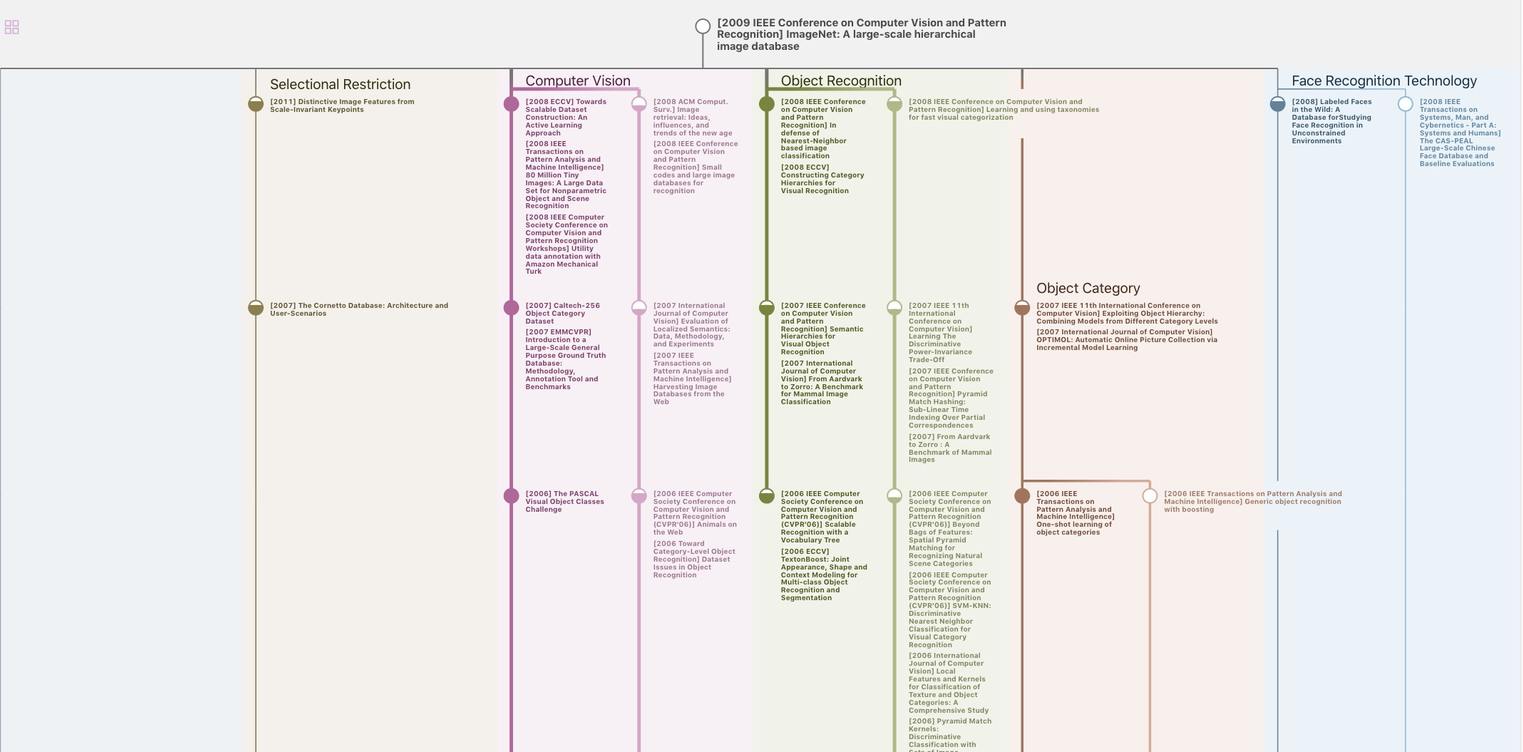
生成溯源树,研究论文发展脉络
Chat Paper
正在生成论文摘要