Accountable Prediction of Drug ADMET Properties with Molecular Descriptors
biorxiv(2022)
摘要
Drugs are chemical substances of low molecular weights that require to travel from the site of administration to the site of action. For safety and effective permeability, drugs are required to exhibit ideal absorption, distribution, metabolism, excretion and toxicity (ADMET) properties. Given the Simplified Molecular Input Line Entry System (SMILES) representation of drugs, we aim to predict their ADMET properties. By feeding molecular descriptors (as global features) to traditional machine learning models, we show that ADMET properties of drug molecules can be predicted with an accuracy competitive with the state-of-the-art deep learning models. We demonstrate that the proposed approach with only 31 molecular descriptors beats the state-of-the-art for 3 datasets. Moreover, it stands the second best for 2 other datasets, where none of the best provides a statistically significant improvement. We also demonstrate that two-dimensional descriptors can better represent absorption, distribution and excretion properties than the fingerprints widely used in the literature. However, they fail to distinguish metabolism and toxicity properties.
### Competing Interest Statement
The authors have declared no competing interest.
更多查看译文
关键词
drug admet properties,molecular
AI 理解论文
溯源树
样例
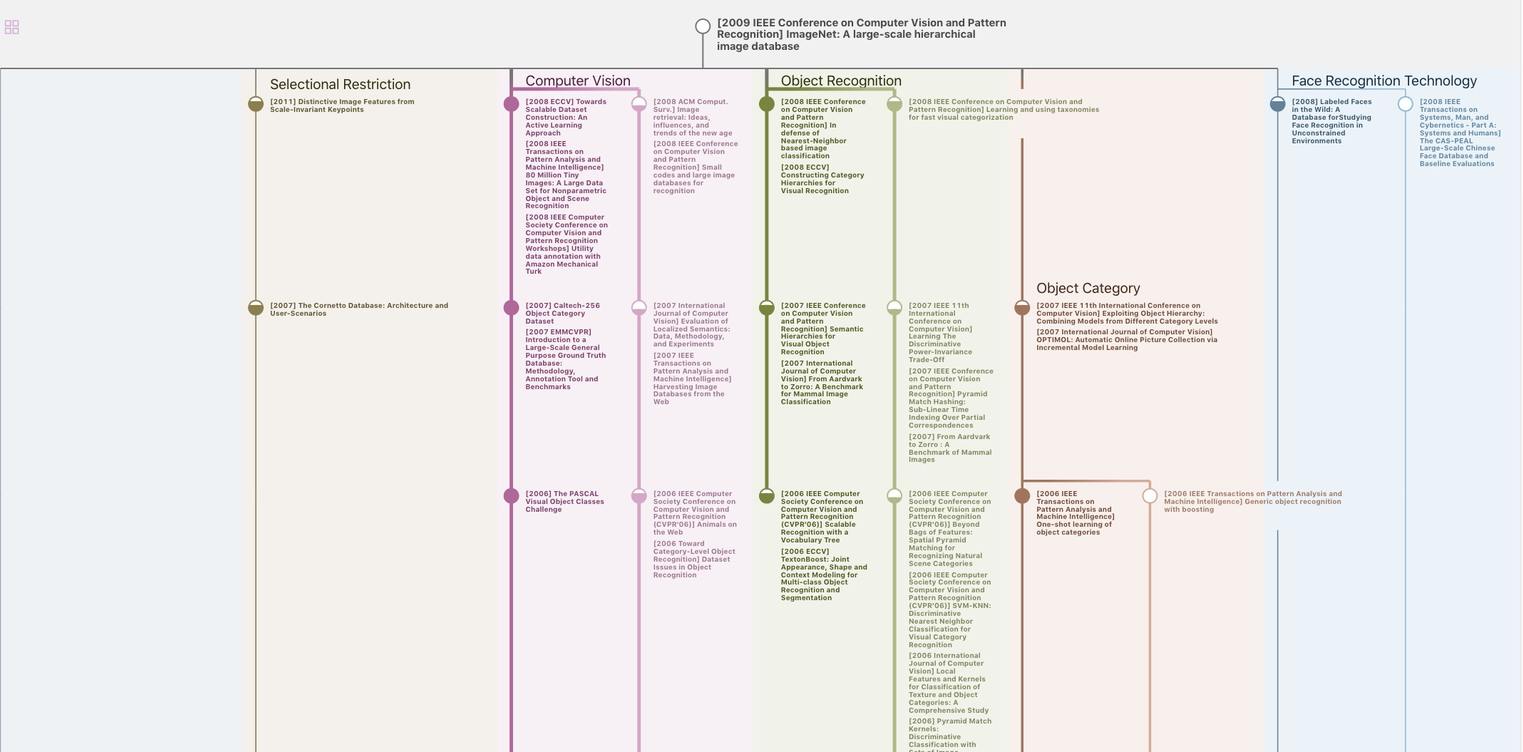
生成溯源树,研究论文发展脉络
Chat Paper
正在生成论文摘要