OSCAR: Optimal subset cardinality regression using the L0-pseudonorm with applications to prognostic modelling of prostate cancer.
PLoS computational biology(2023)
摘要
In many real-world applications, such as those based on electronic health records, prognostic prediction of patient survival is based on heterogeneous sets of clinical laboratory measurements. To address the trade-off between the predictive accuracy of a prognostic model and the costs related to its clinical implementation, we propose an optimized L0-pseudonorm approach to learn sparse solutions in multivariable regression. The model sparsity is maintained by restricting the number of nonzero coefficients in the model with a cardinality constraint, which makes the optimization problem NP-hard. In addition, we generalize the cardinality constraint for grouped feature selection, which makes it possible to identify key sets of predictors that may be measured together in a kit in clinical practice. We demonstrate the operation of our cardinality constraint-based feature subset selection method, named OSCAR, in the context of prognostic prediction of prostate cancer patients, where it enables one to determine the key explanatory predictors at different levels of model sparsity. We further explore how the model sparsity affects the model accuracy and implementation cost. Lastly, we demonstrate generalization of the presented methodology to high-dimensional transcriptomics data.
更多查看译文
关键词
cardinality regression,prognostic modelling,prostate cancer
AI 理解论文
溯源树
样例
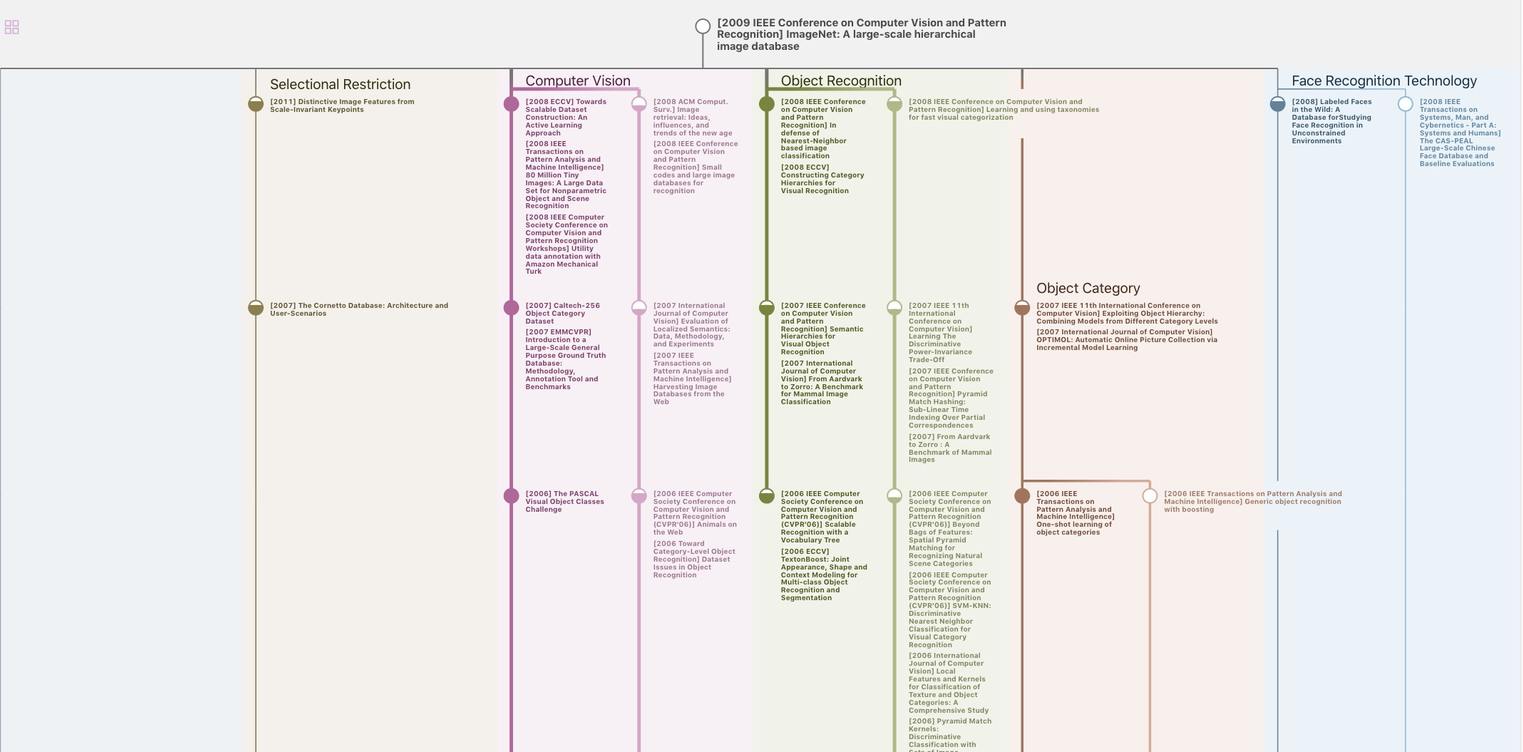
生成溯源树,研究论文发展脉络
Chat Paper
正在生成论文摘要