Protein Structure Prediction with Expectation Reflection
biorxiv(2022)
摘要
Sequence covariation in multiple sequence alignments of homologous proteins has been used extensively to obtain insights into protein structure. However, global statistical inference is required in order to ascertain direct relationships between amino acid positions in these sequences that are not simply secondary correlations induced by interactions with a third residue. Methods for statistical inference of such covariation have been developed to exploit the growing availability of sequence data. These hints about the folded protein structure provide critical a priori information for more detailed 3D predictions by neural networks. We present a novel method for protein structure inference using an iterative parameter-free model estimator which uses the formalism of statistical physics. With no tunable learning rate, our method scales to large system sizes while providing improved performance in the regime of small sample sizes. We apply this method to 40974 PDB structures and compare its performance to that of other methods. Our method outperforms existing methods for 76% of analysed proteins.
### Competing Interest Statement
The authors have declared no competing interest.
更多查看译文
关键词
protein,prediction,structure,reflection
AI 理解论文
溯源树
样例
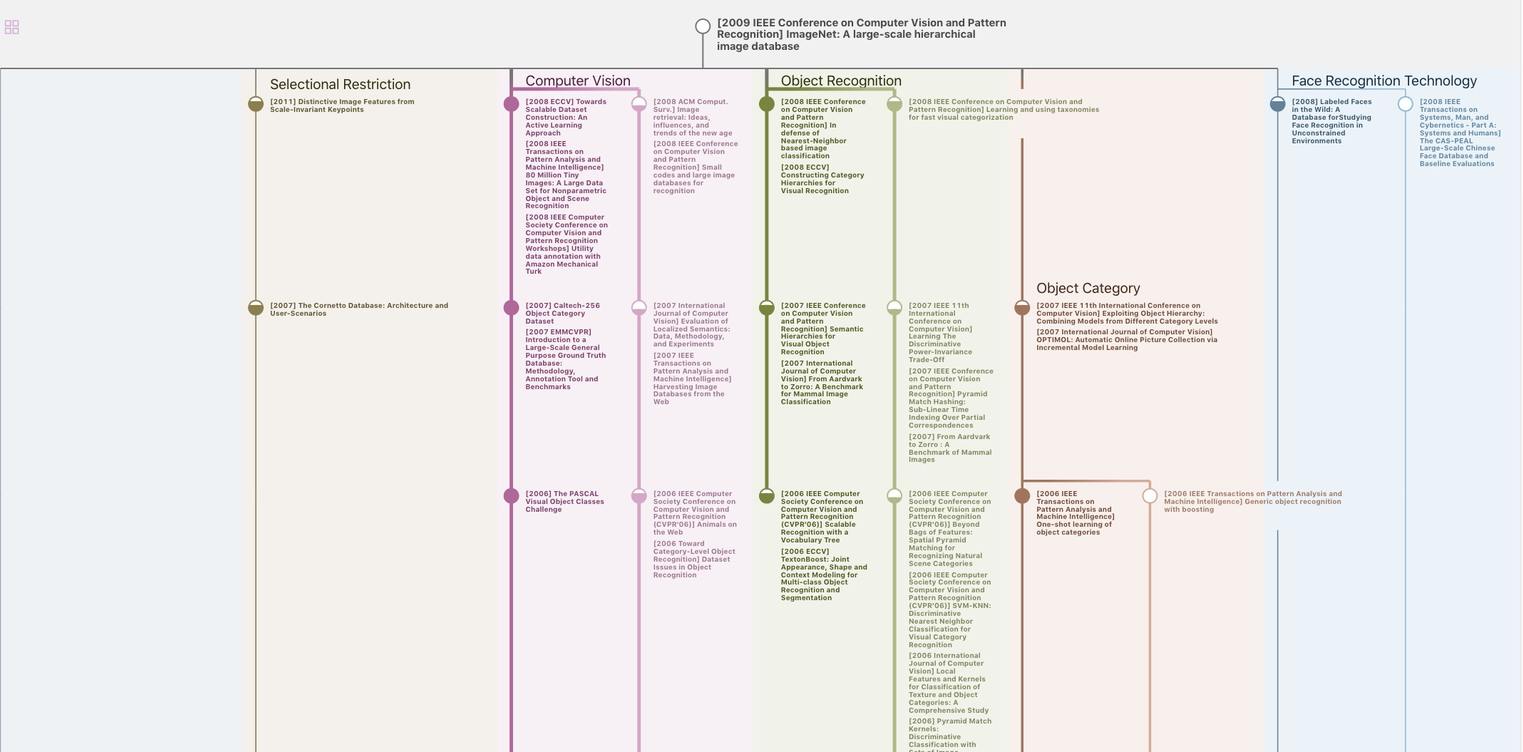
生成溯源树,研究论文发展脉络
Chat Paper
正在生成论文摘要