Generating dynamic carbon-dioxide from the respiratory-volume time series: A feasibility study using neural networks
biorxiv(2022)
摘要
In the context of fMRI, carbon dioxide (CO2) is a well-known vasodilator that has been widely used to monitor and interrogate vascular physiology. Moreover, spontaneous fluctuations in end-tidal carbon dioxide (PETCO2) reflects changes in arterial CO2 and has been demonstrated as the largest physiological noise source in the low-frequency range of the resting-state fMRI (rs-fMRI) signal. Increasing appreciation for the role of CO2 in fMRI has given rise to methods that use it for physiological denoising or estimating cerebrovascular reactivity. However, the majority of rs-fMRI studies do not involve CO2 recordings, and most often only heart rate and respiration are recorded. While the intrinsic link between these latter metrics and CO2 led to suggested possible analytical models, they have not been widely applied. In this proof-of-concept study, we propose a deep learning approach to reconstruct CO2 and PETCO2 data from respiration waveforms in the resting state. We demonstrate that the one-to-one mapping between respiration and CO2 recordings can be well predicted using fully convolutional networks (FCNs), achieving a Pearson correlation coefficient (r) of 0.946 ± 0.056 with the ground truth CO2. Moreover, dynamic PETCO2 can be successfully derived from the predicted CO2, achieving r of 0.512 ± 0.269 with the ground truth. Importantly, the FCN-based methods outperform previously proposed analytical methods. In addition, we provide guidelines for quality assurance of respiration recordings for the purposes of CO2 prediction. Our results demonstrate that dynamic CO2 can be obtained from respiration-volume using neural networks, complementing the still few reports in deep-learning of physiological fMRI signals, and paving the way for further research in deep-learning based bio-signal processing.
### Competing Interest Statement
The authors have declared no competing interest.
更多查看译文
AI 理解论文
溯源树
样例
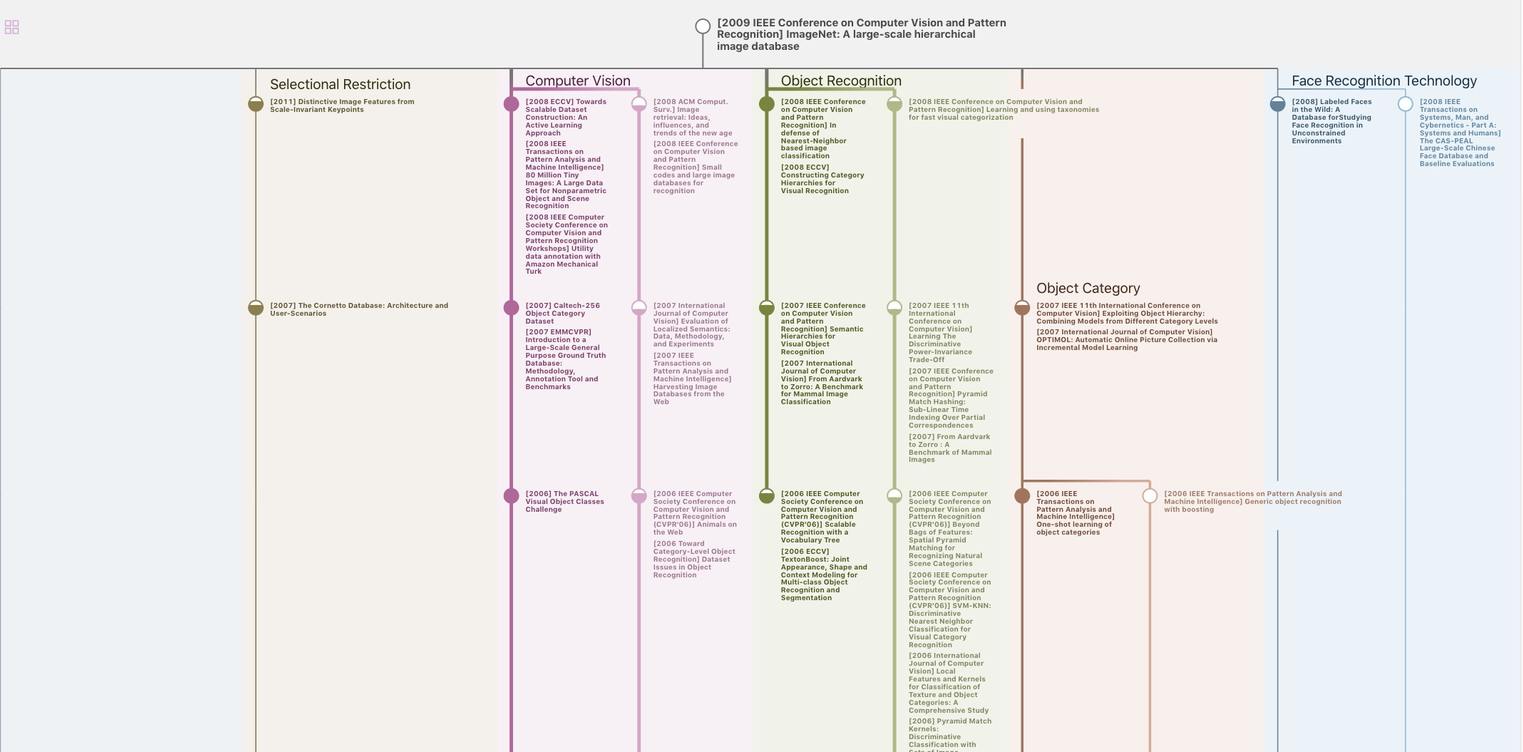
生成溯源树,研究论文发展脉络
Chat Paper
正在生成论文摘要