A transformer model for predicting cognitive impairment from sleep
biorxiv(2022)
摘要
Sleep disturbances are known to be aggravated with normal aging. Additionally, sleep disruptions have a potentially bidirectional causal relationship with dementia due to neurodegenerative diseases like Alzheimer’s disease. Predictive techniques that can automatically detect cognitive impairment from an individual’s sleep data have broad clinical and biological significance. Here, we present a deep learning approach based on a transformer architecture to predict cognitive status from sleep electroencephalography (EEG) data. This work uses data from N = 1, 502 subjects from the Multi-Ethnic Study of Atherosclerosis (MESA) cohort. Our transformer model achieves 70.22% accuracy at the binary classification task for distinguishing cognitively normal and impaired subjects based on their sleep EEG. Our method outperforms traditional feature handcrafting, which has an overall accuracy of 57.61% for the same task. We use a sparse regression model to understand and interpret the information captured by each learned feature from our transformer model. To our knowledge, this is the first effort to use deep learning to predict cognitive impairment from sleep metrics.
### Competing Interest Statement
The authors have declared no competing interest.
更多查看译文
关键词
cognitive impairment,transformer model,sleep
AI 理解论文
溯源树
样例
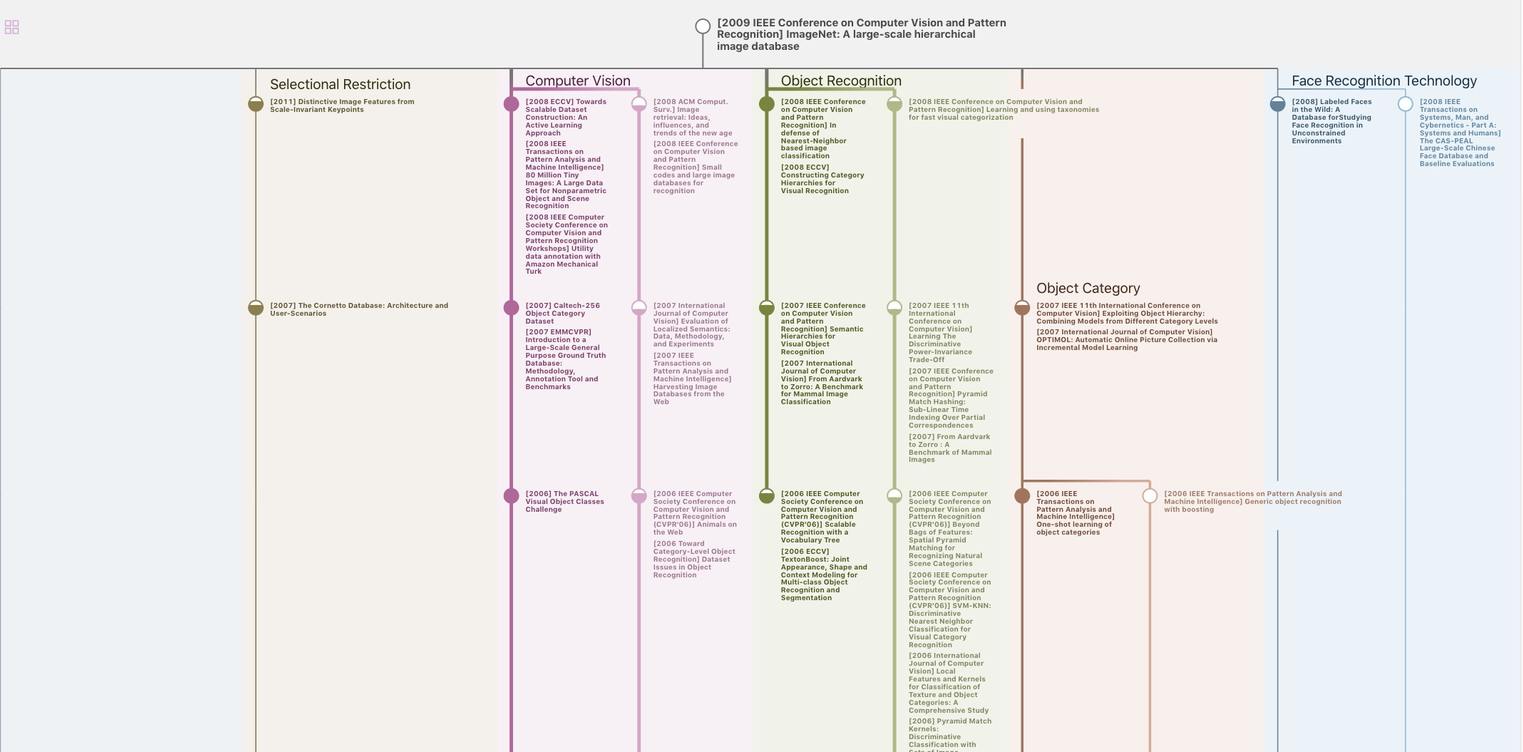
生成溯源树,研究论文发展脉络
Chat Paper
正在生成论文摘要