Studying the explanations for the automated prediction of bug and non-bug issues using LIME and SHAP
arxiv(2022)
摘要
Context: The identification of bugs within the reported issues in an issue tracker is crucial for the triage of issues. Machine learning models have shown promising results regarding the performance of automated issue type prediction. However, we have only limited knowledge beyond our assumptions how such models identify bugs. LIME and SHAP are popular technique to explain the predictions of classifiers. Objective: We want to understand if machine learning models provide explanations for the classification that are reasonable to us as humans and align with our assumptions of what the models should learn. We also want to know if the prediction quality is correlated with the quality of explanations. Method: We conduct a study where we rate LIME and SHAP explanations based on their quality of explaining the outcome of an issue type prediction model. For this, we rate the quality of the explanations themselves, i.e., if they align with our expectations and if they help us to understand the underlying machine learning model.
更多查看译文
关键词
explanations,prediction,issues,shap,non-bug
AI 理解论文
溯源树
样例
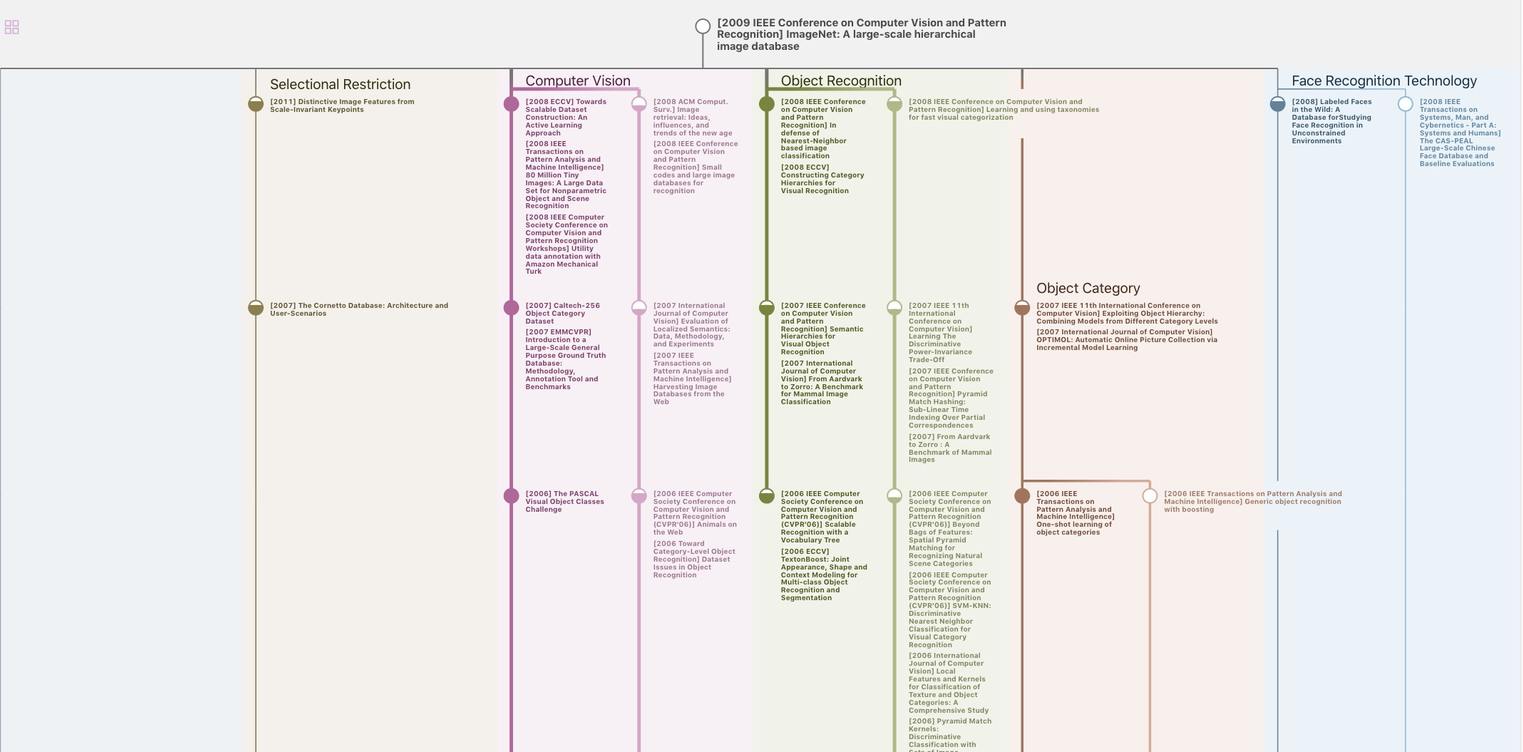
生成溯源树,研究论文发展脉络
Chat Paper
正在生成论文摘要