Continual few-shot learning with Hippocampal-inspired replay
CoRR(2022)
摘要
Continual learning and few-shot learning are important frontiers in the quest to improve Machine Learning. There is a growing body of work in each frontier, but very little combining the two. Recently however, Antoniou et al. arXiv:2004.11967 introduced a Continual Few-shot Learning framework, CFSL, that combines both. In this study, we extended CFSL to make it more comparable to standard continual learning experiments, where usually a much larger number of classes are presented. We also introduced an `instance test' to classify very similar specific instances - a capability of animal cognition that is usually neglected in ML. We selected representative baseline models from the original CFSL work and compared to a model with Hippocampal-inspired replay, as the Hippocampus is considered to be vital to this type of learning in animals. As expected, learning more classes is more difficult than the original CFSL experiments, and interestingly, the way in which they are presented makes a difference to performance. Accuracy in the instance test is comparable to the classification tasks. The use of replay for consolidation improves performance substantially for both types of tasks, particularly the instance test.
更多查看译文
AI 理解论文
溯源树
样例
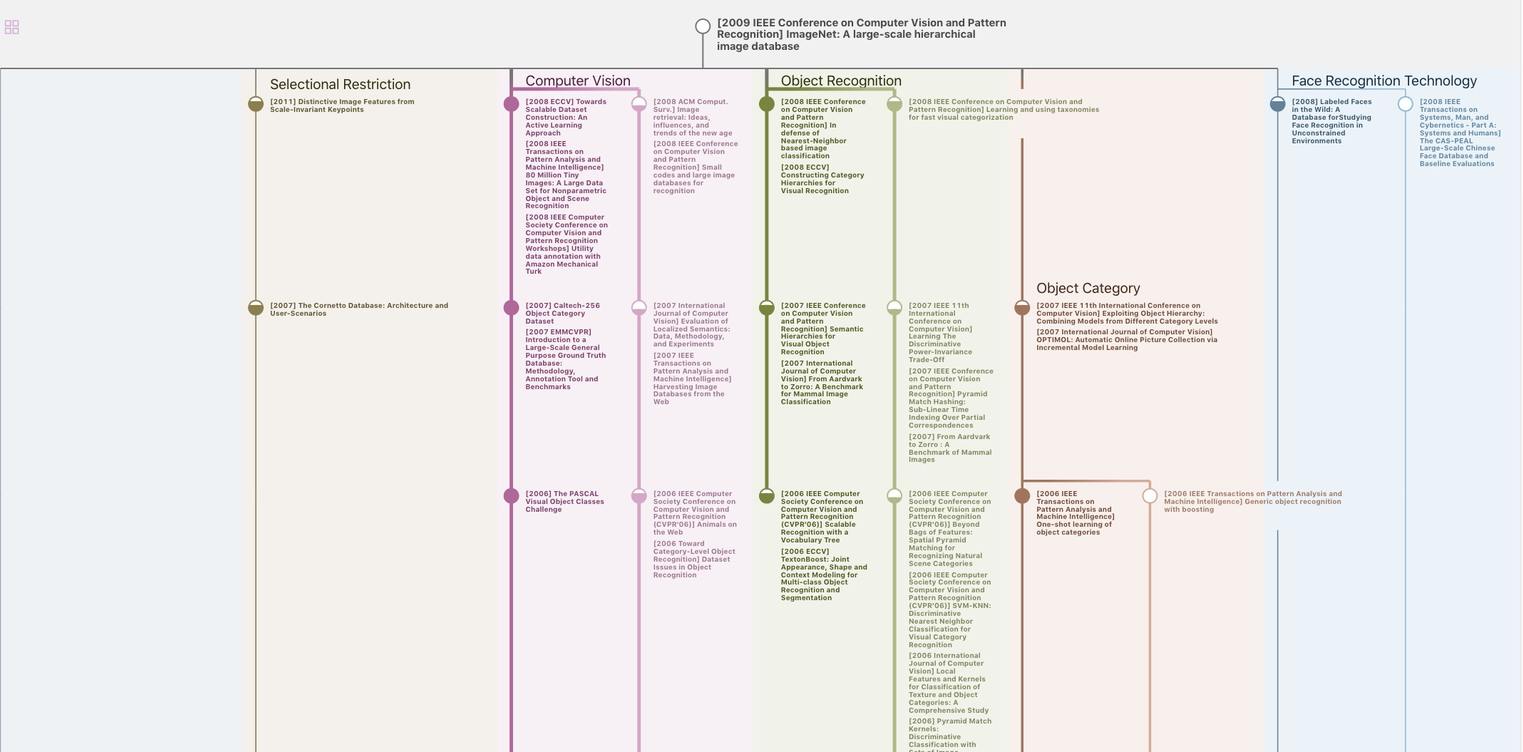
生成溯源树,研究论文发展脉络
Chat Paper
正在生成论文摘要