Deep or Not Deep: Supervised Learning Approaches to Modeling the Pedestal Density
arxiv(2022)
摘要
Pedestal is the key to conventional high performance plasma scenarios in tokamaks. However, high fidelity simulations of pedestal plasmas are extremely challenging due to the multiple physical processes and scales that are encompassed by tokamak pedestals. The leading paradigm for predicting the pedestal top pressure is encompassed by EPED-like models. However, EPED does not predict the pedestal top density, $n_\text{e,ped}$, but requires it as an input. EUROPED employs simplified models, such as log-linear regression, to constrain $n_\text{e,ped}$ with tokamak machine control parameters in an EPED-like model. However, these simplified models for $n_\text{e,ped}$ often show disagreements with experimental observations and do not use all of the available numerical and categorical machine control information. In this work it is observed that using the same input parameters, decision tree ensembles and deep learning models improve the predictive quality of $n_\text{e,ped}$ by about 23% relative to that obtained with log-linear scaling laws, measured by root mean square error. Including all of the available tokamak machine control parameters, both numerical and categorical, leads to further improvement of about 13%. Finally, predictive quality was tested when including global normalized plasma pressure and effective charge state as inputs, as these parameters are known to impact pedestals. Surprisingly, these parameters lead to only a few percent further improvement of the predictive quality.
更多查看译文
关键词
supervised learning approaches,density,modeling
AI 理解论文
溯源树
样例
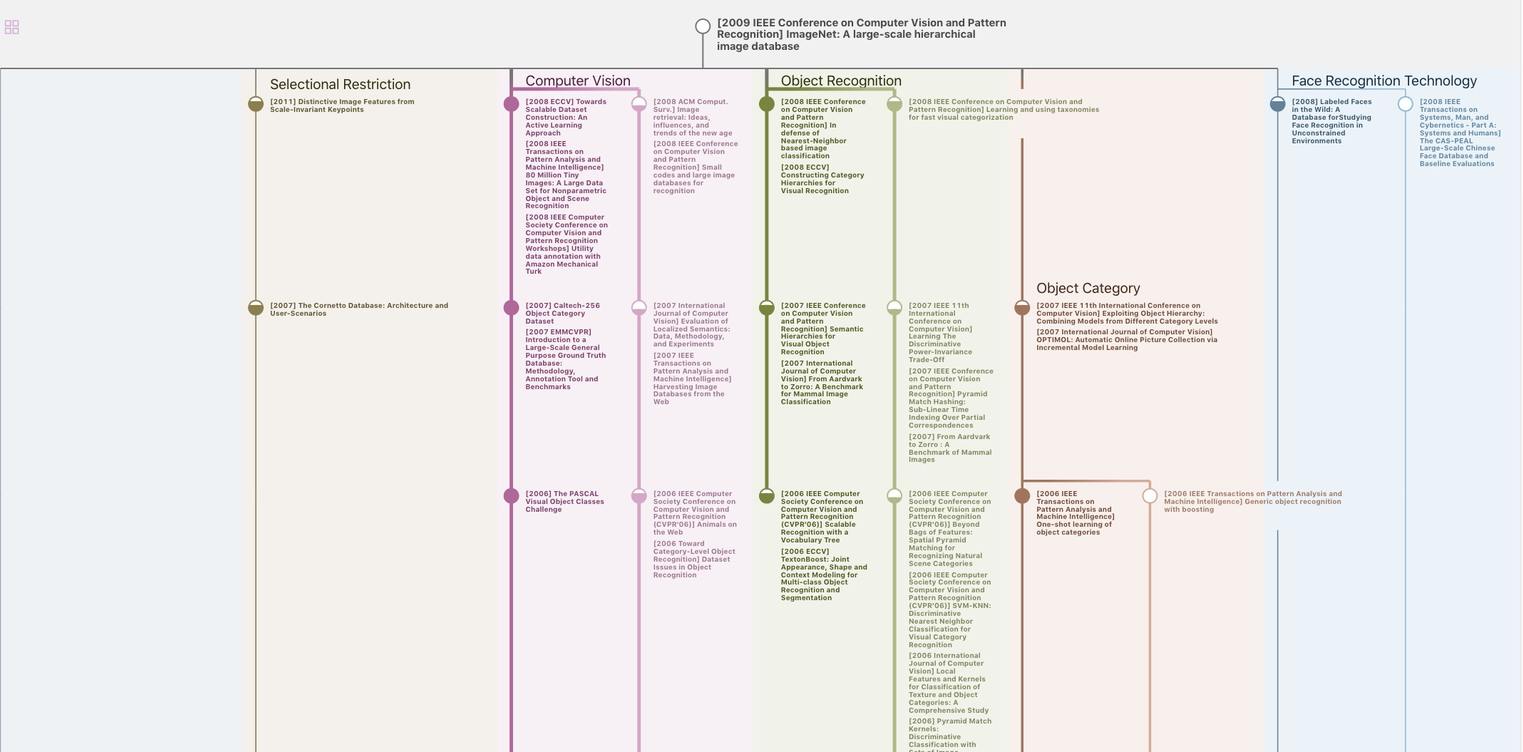
生成溯源树,研究论文发展脉络
Chat Paper
正在生成论文摘要