Pupil Dynamics-derived Sleep Stage Classification of a Head-fixed Mouse Using a Recurrent Neural Network
biorxiv(2023)
摘要
The standard method for sleep state classification is thresholding the amplitudes of electroencepha- lography (EEG) and electromyography (EMG) data, followed by manual correction by an expert. Al- though popular, this method has some shortcomings: (1) the time-consuming manual correction by hu- man experts is sometimes a bottleneck hindering sleep studies, (2) EEG electrodes on the skull interfere with wide-field imaging of the cortical activity of a head-fixed mouse under a microscope, (3) invasive surgery to fix the electrodes on the thin mouse skull risks brain tissue injury, and (4) metal electrodes for EEG and EMG recording are difficult to apply to some experimental apparatus such as that for functional magnetic resonance imaging. To overcome these shortcomings, we propose a pupil dynamics-based vigilance state classification method for a head-fixed mouse using a long short-term memory (LSTM) model, a variant of a recurrent neural network, for multi-class labeling of NREM, REM, and WAKE states. For supervisory hypnography, EEG and EMG recording were performed on head-fixed mice. This setup was combined with left eye pupillometry using a USB camera and a markerless track -ing toolbox, DeepLabCut. Our open-source LSTM model with feature inputs of pupil diameter, pupil location, pupil velocity, and eyelid opening for 10 s at a 10 Hz sampling rate achieved vigilance state estimation with a higher classification performance (macro F1 score, 0.77; accuracy, 86%) than a feed-forward neural network. Findings from a diverse range of pupillary dynamics implied possible subdivi- sion of the vigilance states defined by EEG and EMG. Pupil dynamics-based hypnography can expand the scope of alternatives for sleep stage scoring of head-fixed mice.
更多查看译文
关键词
deep learning,eyelids,open source,pupil dynamics,rodents,sleep staging
AI 理解论文
溯源树
样例
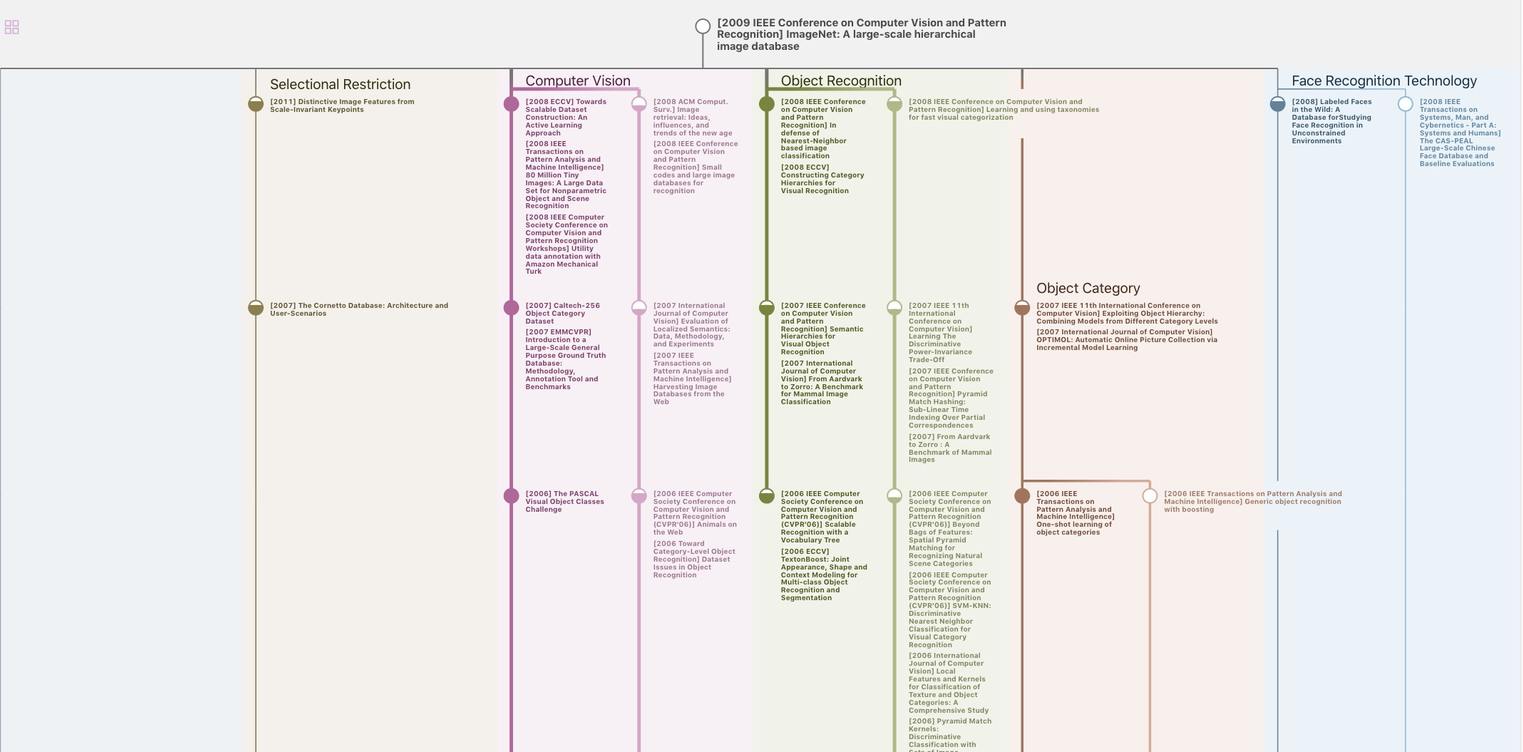
生成溯源树,研究论文发展脉络
Chat Paper
正在生成论文摘要