Autoencoders - A Comparative Analysis in the Realm of Anomaly Detection
IEEE Conference on Computer Vision and Pattern Recognition(2022)
摘要
We applied convolutional versions of a "standard" autoencoder (CAE), a variational autoencoder (VAE) and an adversarial autoencoder (AAE) to two different publicly available datasets and compared their anomaly detection performances. We used the MNIST dataset [14] as a simple anomaly detection scenario. The CIFAR10 dataset [13] was used to examine the autoencoders in a more complex anomaly detection task. The anomaly detection performance of our different autoencoder types is compared in a qualitative and quantitative manner. The time needed for training the models is measured to capture their complexity. The simplest model demanding the simplest training, the CAE, computes results which are nearly as accurate and for some cases even better than results achieved by the VAE and AAE. We show that all three autoencoder types computed convincing anomaly detection results for the more simple-structured MNIST scenario. However, none of the autoencoder types proved to capture a good representation of the relevant features of the more complex CIFAR10 dataset, leading to moderately good anomaly detection performances.
更多查看译文
关键词
detection
AI 理解论文
溯源树
样例
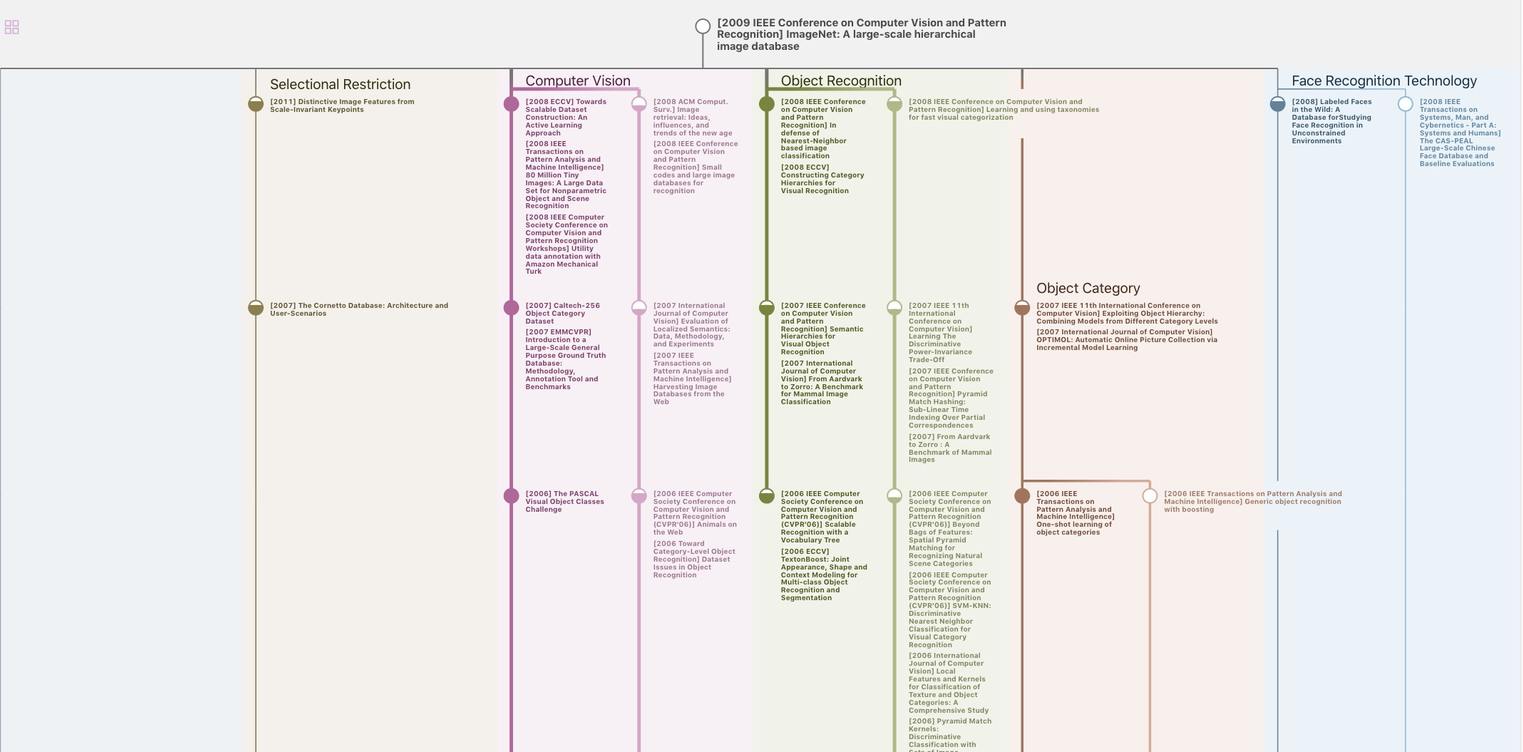
生成溯源树,研究论文发展脉络
Chat Paper
正在生成论文摘要