Performance Prediction for Semantic Segmentation by a Self-Supervised Image Reconstruction Decoder
IEEE Conference on Computer Vision and Pattern Recognition(2022)
摘要
In supervised learning, a deep neural network’s performance is measured using ground truth data. In semantic segmentation, ground truth data is sparse, requires an expensive annotation process, and, most importantly, it is not available during online operation. To tackle this problem, recent works propose various forms of performance prediction. However, they either rely on inference data histograms, additional sensors, or additional training data. In this paper, we propose a novel per-image performance prediction for semantic segmentation, with (i) no need for additional sensors (sensor efficiency), (ii) no need for additional training data (data efficiency), and (iii) no need for a dedicated retraining of the semantic segmentation (training efficiency). Specifically, we extend an already trained semantic segmentation network having fixed parameters with an image reconstruction decoder. After training and a subsequent regression, the image reconstruction quality is evaluated to predict the semantic segmentation performance. We demonstrate our method’s effectiveness with a new state-of-the-art benchmark both on KITTI and Cityscapes for image-only input methods, on Cityscapes even excelling a LiDAR-supported benchmark.
更多查看译文
关键词
self-supervised image reconstruction decoder,supervised learning,deep neural network,ground truth data,expensive annotation process,inference data histograms,additional training data,per-image performance prediction,additional sensors,sensor efficiency,data efficiency,trained semantic segmentation network,image reconstruction quality,semantic segmentation performance,image-only input methods,performance prediction,fixed parameters,image reconstruction decoder,subsequent regression,KITTI,Cityscapes,LiDAR-supported benchmark
AI 理解论文
溯源树
样例
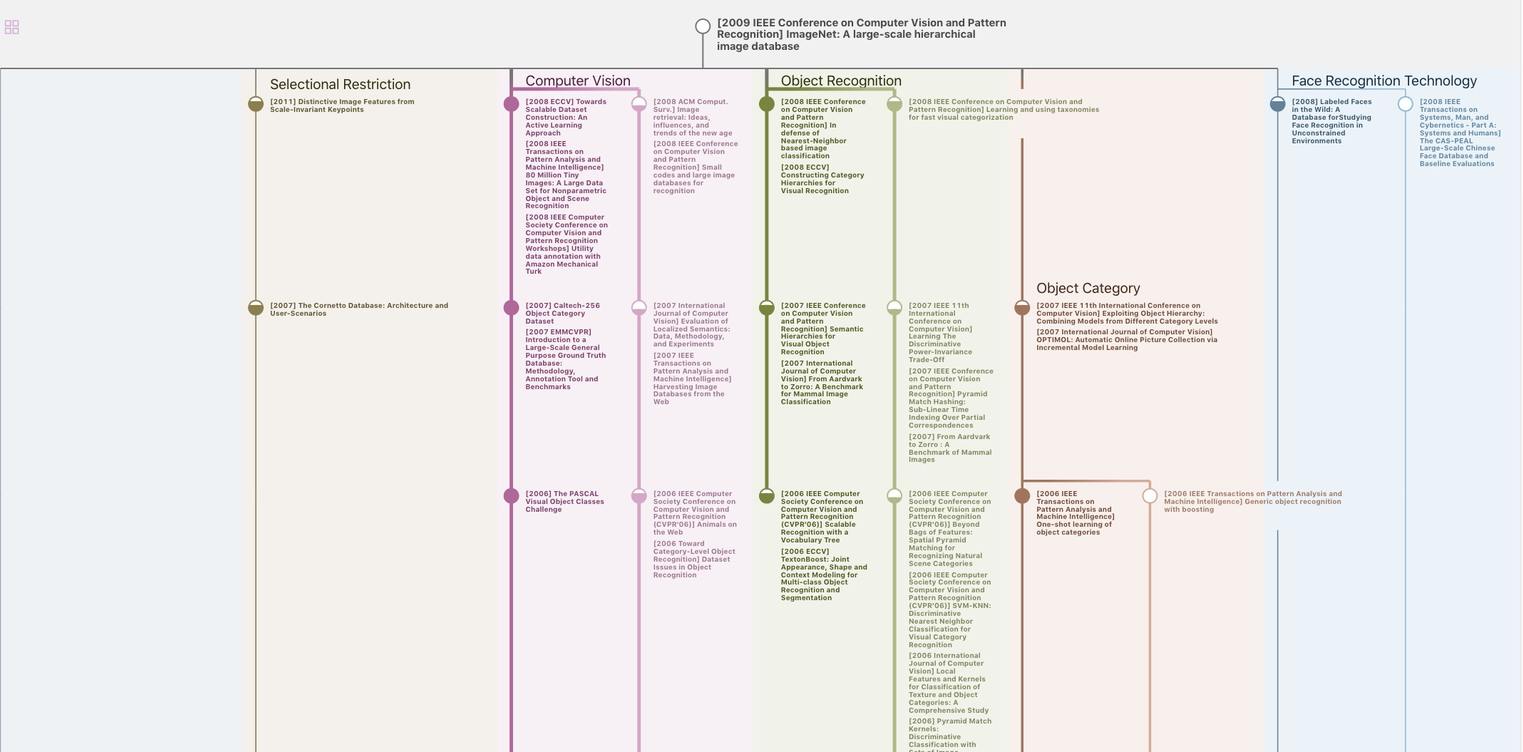
生成溯源树,研究论文发展脉络
Chat Paper
正在生成论文摘要