Strategies and Techniques for Quality Control and Semantic Enrichment with Multimodal Data: A Case Study in Colorectal Cancer with eHDPrep
biorxiv(2022)
摘要
Background Integration of data from multiple domains can greatly enhance the quality and applicability of knowledge generated in analysis workflows. However, working with health data is challenging, requiring careful preparation in order to support meaningful interpretation and robust results. Ontologies encapsulate relationships between variables that can enrich the semantic content of health datasets to enhance interpretability and inform downstream analyses.
Findings We developed an R package for electronic Health Data preparation ‘eHDPrep’, demonstrated upon a multi-modal colorectal cancer dataset (n=661 patients, n=155 variables; Colo-661). eHDPrep offers user-friendly methods for quality control, including internal consistency checking and redundancy removal with information-theoretic variable merging. Semantic enrichment functionality is provided, enabling generation of new informative ‘meta-variables’ according to ontological common ancestry between variables, demonstrated with SNOMED CT and the Gene Ontology in the current study. eHDPrep also facilitates numerical encoding, variable extraction from free-text, completeness analysis and user review of modifications to the dataset.
Conclusion eHDPrep provides effective tools to assess and enhance data quality, laying the foundation for robust performance and interpretability in downstream analyses. Application to a multi-modal colorectal cancer dataset resulted in improved data quality, structuring, and robust encoding, as well as enhanced semantic information. We make eHDPrep available as an R package from CRAN [[URL will go here]].
### Competing Interest Statement
IO has provided consultancy for Mevox Ltd for work unrelated to this publication.
* CRC
: colorectal cancer
QC
: quality control
GO
: gene ontology
SNOMED CT
: systematized nomenclature of medicine clinical terms
IC
: information content
MIC
: mutual information content
MICA
: most informative common ancestor.
更多查看译文
关键词
multimodal data,semantic enrichment,colorectal cancer,quality control
AI 理解论文
溯源树
样例
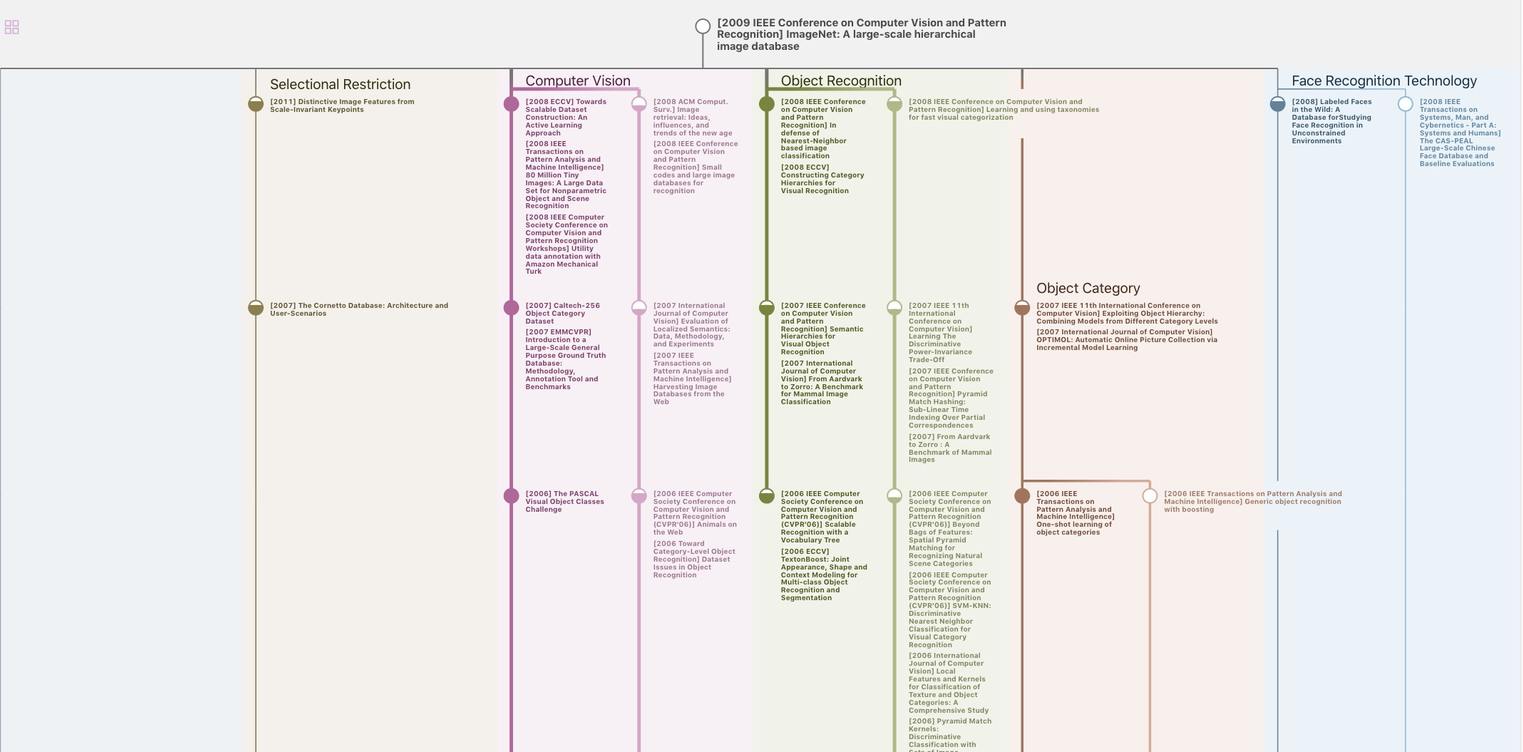
生成溯源树,研究论文发展脉络
Chat Paper
正在生成论文摘要