Undersampling reconstruction in parallel and single coil imaging with COMPaS -- COnvolutional Magnetic Resonance Image Prior with Sparsity regularization
arxiv(2022)
摘要
Purpose: To propose COMPaS, a learning-free Convolutional Network, that combines Deep Image Prior (DIP) with transform-domain sparsity constraints to reconstruct undersampled Magnetic Resonance Imaging (MRI) data without previous training of the network. Methods: COMPaS uses a U-Net as DIP for undersampledMRdata in the image domain. Reconstruction is constrained by data fidelity to k-space measurements and transform-domain sparsity, such as Total Variation (TV) or Wavelet transform sparsity. Two-dimensional MRI data from the public FastMRI dataset with Cartesian undersampling in phase-encoding direction were reconstructed for different acceleration rates (R) from R = 2 to R = 8 for single coil and multicoil data. Performance of the proposed architecture was compared to Parallel Imaging with Compressed Sensing (PICS). Results: COMPaS outperforms standard PICS algorithms by reducing ghosting artifacts and yielding higher quantitative reconstruction quality metrics in multicoil imaging settings and especially in single coil k-space reconstruction. Furthermore, COMPaS can reconstruct multicoil data without explicit knowledge of coil sensitivity profiles. Conclusion: COMPaS utilizes a training-free convolutional network as a DIP in MRI reconstruction and transforms it with transform-domain sparsity regularization. It is a competitive algorithm for parallel imaging and a novel tool for accelerating single coil MRI.
更多查看译文
AI 理解论文
溯源树
样例
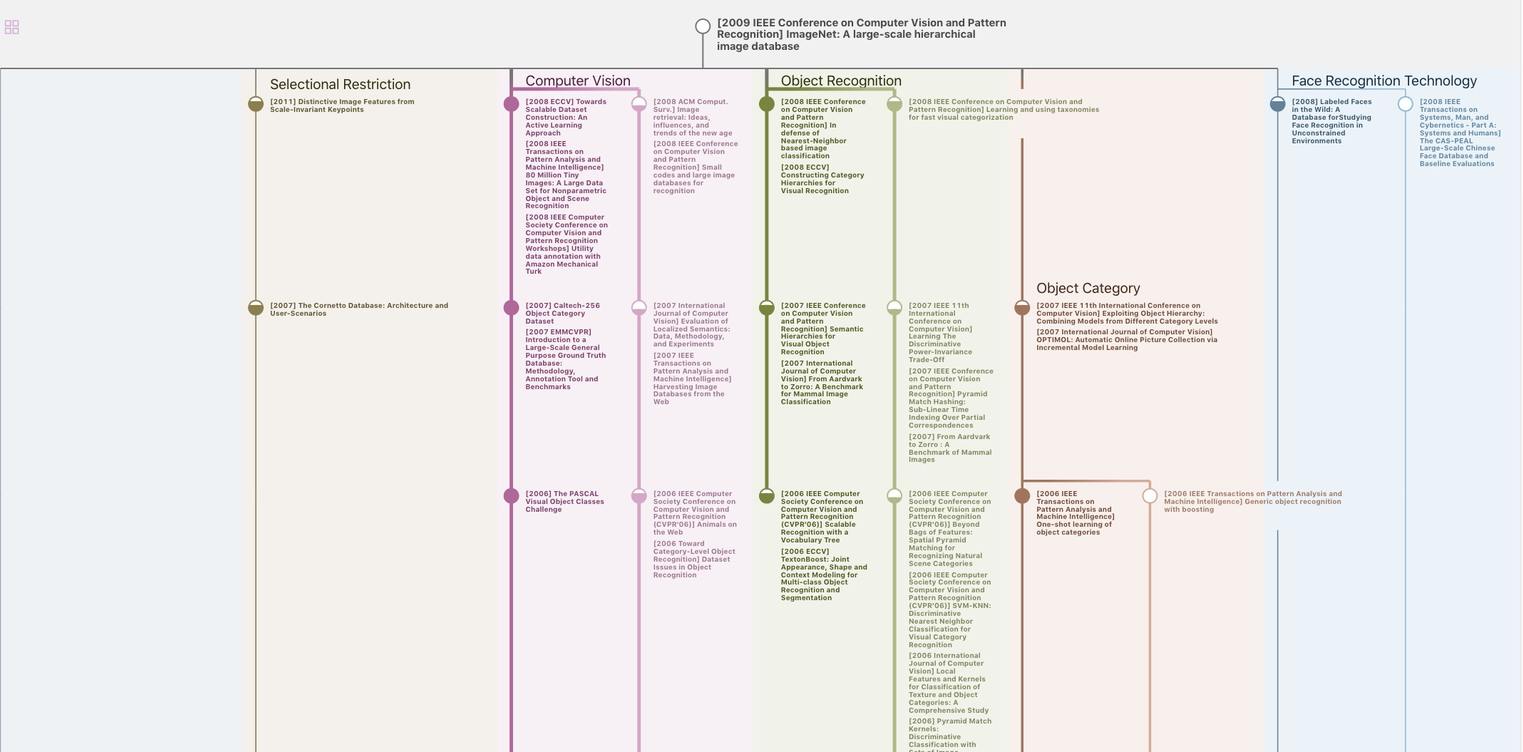
生成溯源树,研究论文发展脉络
Chat Paper
正在生成论文摘要