TODE-Trans: Transparent Object Depth Estimation with Transformer
2023 IEEE INTERNATIONAL CONFERENCE ON ROBOTICS AND AUTOMATION, ICRA(2023)
摘要
Transparent objects are widely used in industrial automation and daily life. However, robust visual recognition and perception of transparent objects have always been a major challenge. Currently, most commercial-grade depth cameras are still not good at sensing the surfaces of transparent objects due to the refraction and reflection of light. In this work, we present a transformer-based transparent object depth estimation approach from a single RGB-D input. We observe that the global characteristics of the transformer make it easier to extract contextual information to perform depth estimation of transparent areas. In addition, to better enhance the fine-grained features, a feature fusion module (FFM) is designed to assist coherent prediction. Our empirical evidence demonstrates that our model delivers significant improvements in recent popular datasets, e.g., 25% gain on RMSE and 21% gain on REL compared to previous state-of-the-art convolutional-based counterparts in ClearGrasp dataset. Extensive results show that our transformer-based model enables better aggregation of the object's RGB and inaccurate depth information to obtain a better depth representation. Our code and the pre-trained model are available at https://github.com/yuchendoudou/TODE.
更多查看译文
关键词
commercial-grade depth cameras,depth representation,inaccurate depth information,robust visual recognition,transformer-based model,transformer-based transparent object depth estimation approach,transparent areas,transparent objects
AI 理解论文
溯源树
样例
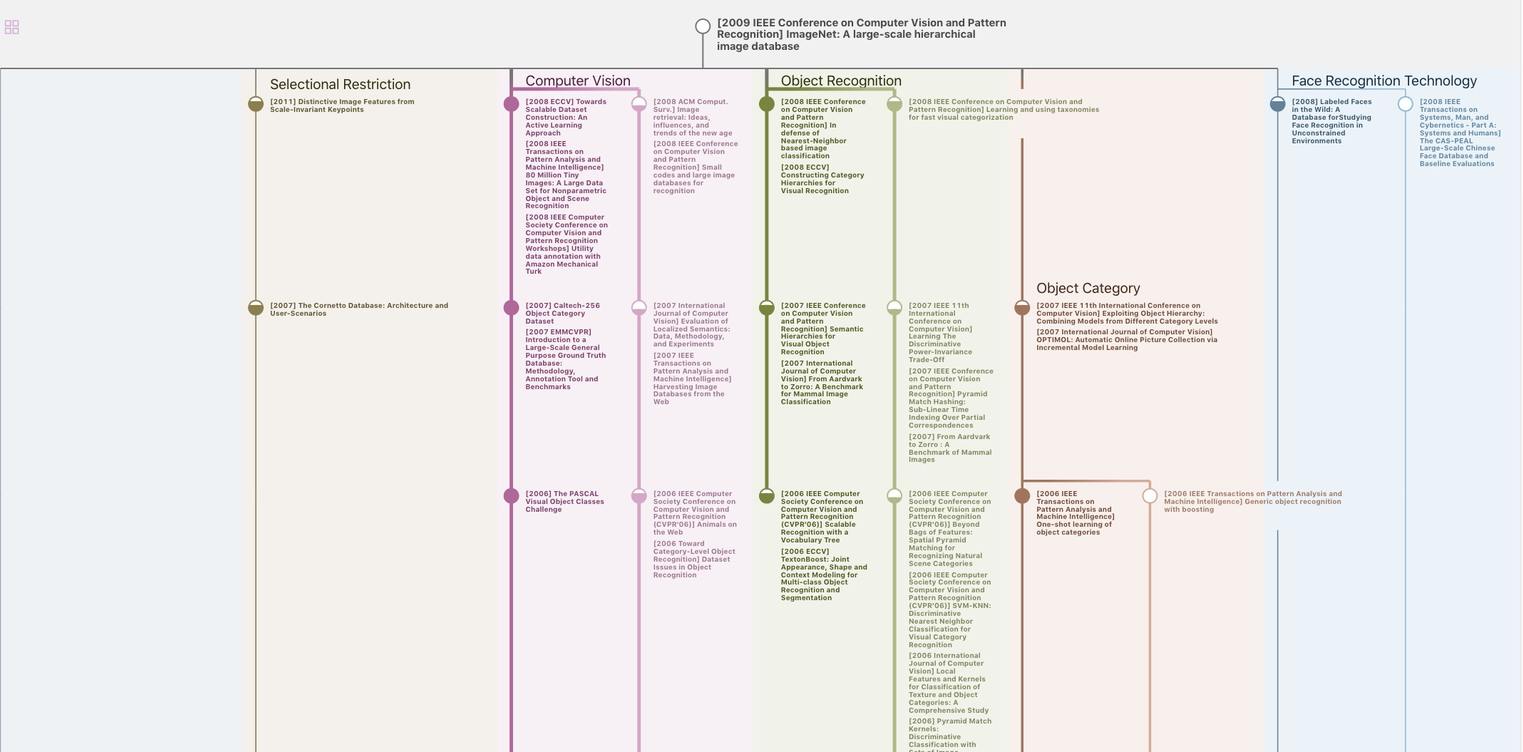
生成溯源树,研究论文发展脉络
Chat Paper
正在生成论文摘要