Density-aware NeRF Ensembles: Quantifying Predictive Uncertainty in Neural Radiance Fields
arxiv(2022)
摘要
We show that ensembling effectively quantifies model uncertainty in Neural Radiance Fields (NeRFs) if a density-aware epistemic uncertainty term is considered. The naive ensembles investigated in prior work simply average rendered RGB images to quantify the model uncertainty caused by conflicting explanations of the observed scene. In contrast, we additionally consider the termination probabilities along individual rays to identify epistemic model uncertainty due to a lack of knowledge about the parts of a scene unobserved during training. We achieve new state-of-the-art performance across established uncertainty quantification benchmarks for NeRFs, outperforming methods that require complex changes to the NeRF architecture and training regime. We furthermore demonstrate that NeRF uncertainty can be utilised for next-best view selection and model refinement.
更多查看译文
关键词
conflicting explanations,density-aware epistemic uncertainty term,density-aware NeRF ensembles,epistemic model uncertainty,established uncertainty quantification benchmarks,model refinement,naive ensembles,NeRF architecture,NeRF uncertainty,NeRFs,Neural Radiance Fields,observed scene,quantifying predictive uncertainty,RGB images,view selection
AI 理解论文
溯源树
样例
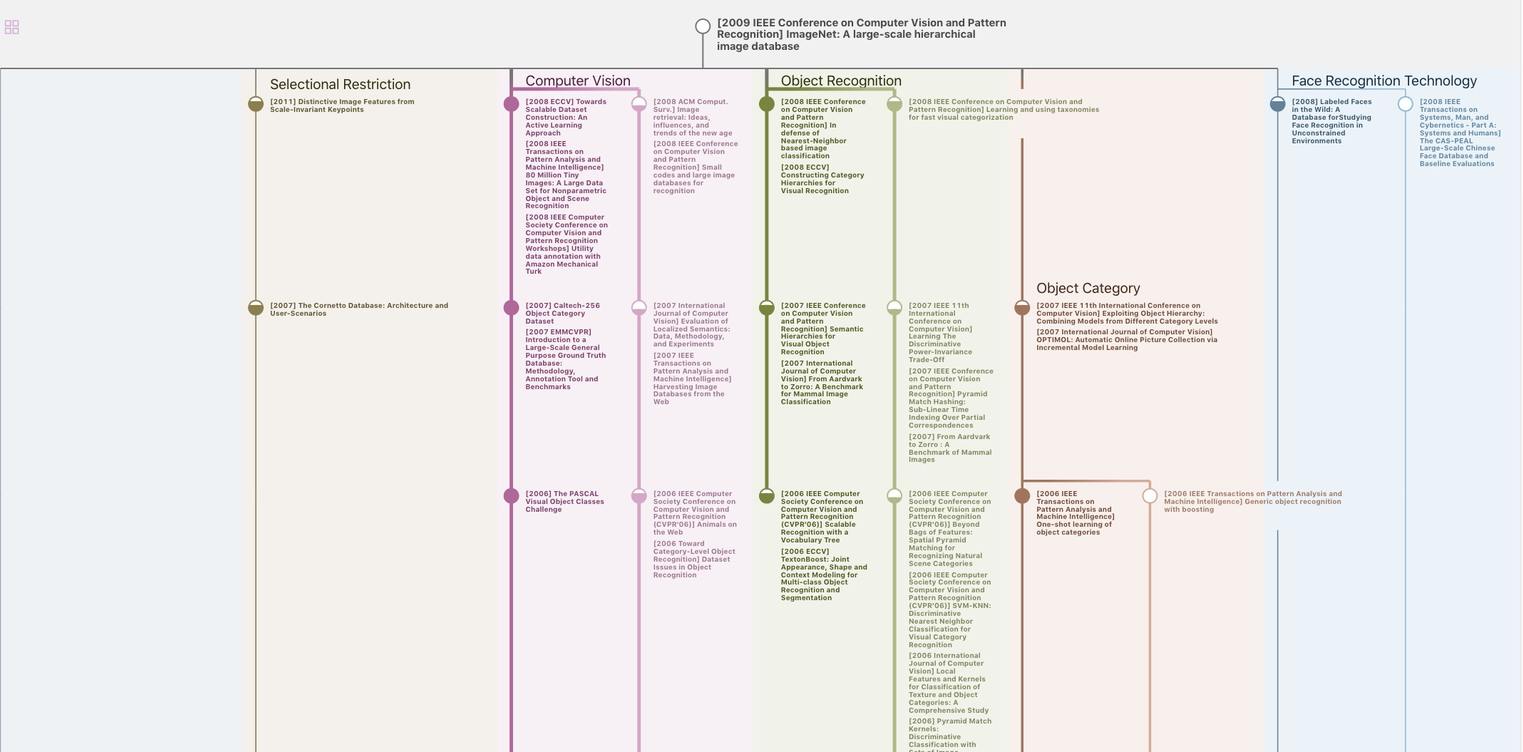
生成溯源树,研究论文发展脉络
Chat Paper
正在生成论文摘要