Importance Tempering: Group Robustness for Overparameterized Models
arxiv(2022)
摘要
Although overparameterized models have shown their success on many machine learning tasks, the accuracy could drop on the testing distribution that is different from the training one. This accuracy drop still limits applying machine learning in the wild. At the same time, importance weighting, a traditional technique to handle distribution shifts, has been demonstrated to have less or even no effect on overparameterized models both empirically and theoretically. In this paper, we propose importance tempering to improve the decision boundary and achieve consistently better results for overparameterized models. Theoretically, we justify that the selection of group temperature can be different under label shift and spurious correlation setting. At the same time, we also prove that properly selected temperatures can extricate the minority collapse for imbalanced classification. Empirically, we achieve state-of-the-art results on worst group classification tasks using importance tempering.
更多查看译文
关键词
group robustness,models,importance
AI 理解论文
溯源树
样例
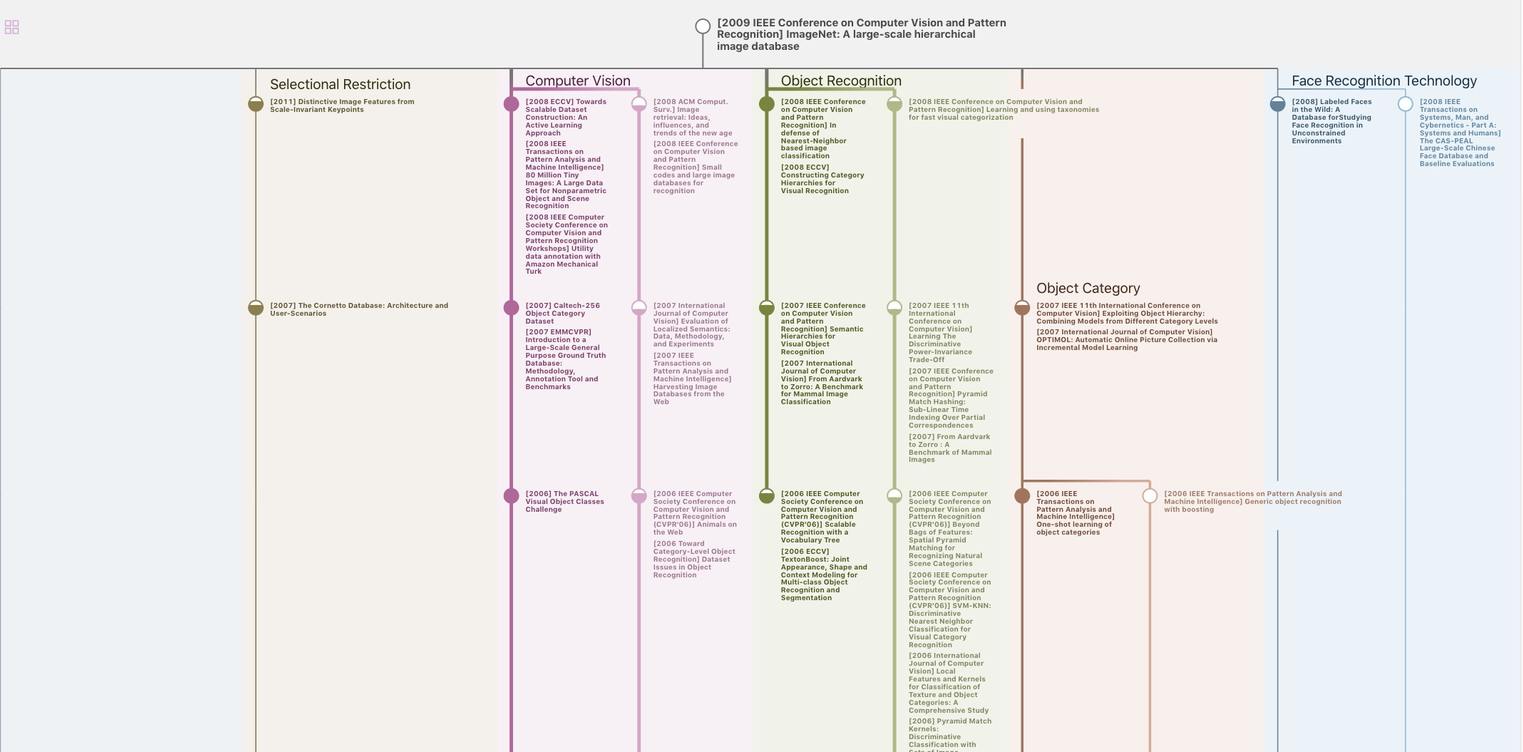
生成溯源树,研究论文发展脉络
Chat Paper
正在生成论文摘要