Extremal Points and Sparse Optimization for Generalized Kantorovich–Rubinstein Norms
Foundations of Computational Mathematics(2023)
摘要
A precise characterization of the extremal points of sublevel sets of nonsmooth penalties provides both detailed information about minimizers, and optimality conditions in general classes of minimization problems involving them. Moreover, it enables the application of fully corrective generalized conditional gradient methods for their efficient solution. In this manuscript, this program is adapted to the minimization of a smooth convex fidelity term which is augmented with an unbalanced transport regularization term given in the form of a generalized Kantorovich–Rubinstein norm for Radon measures. More precisely, we show that the extremal points associated to the latter are given by all Dirac delta functionals supported in the spatial domain as well as certain dipoles, i.e., pairs of Diracs with the same mass but with different signs. Subsequently, this characterization is used to derive precise first-order optimality conditions as well as an efficient solution algorithm for which linear convergence is proved under natural assumptions. This behavior is also reflected in numerical examples for a model problem.
更多查看译文
关键词
Extremal points,Unbalanced optimal transport,Sparse optimization,Conditional gradient methods,Inverse problems,Optimal design,Kantorovich–Rubinstein duality
AI 理解论文
溯源树
样例
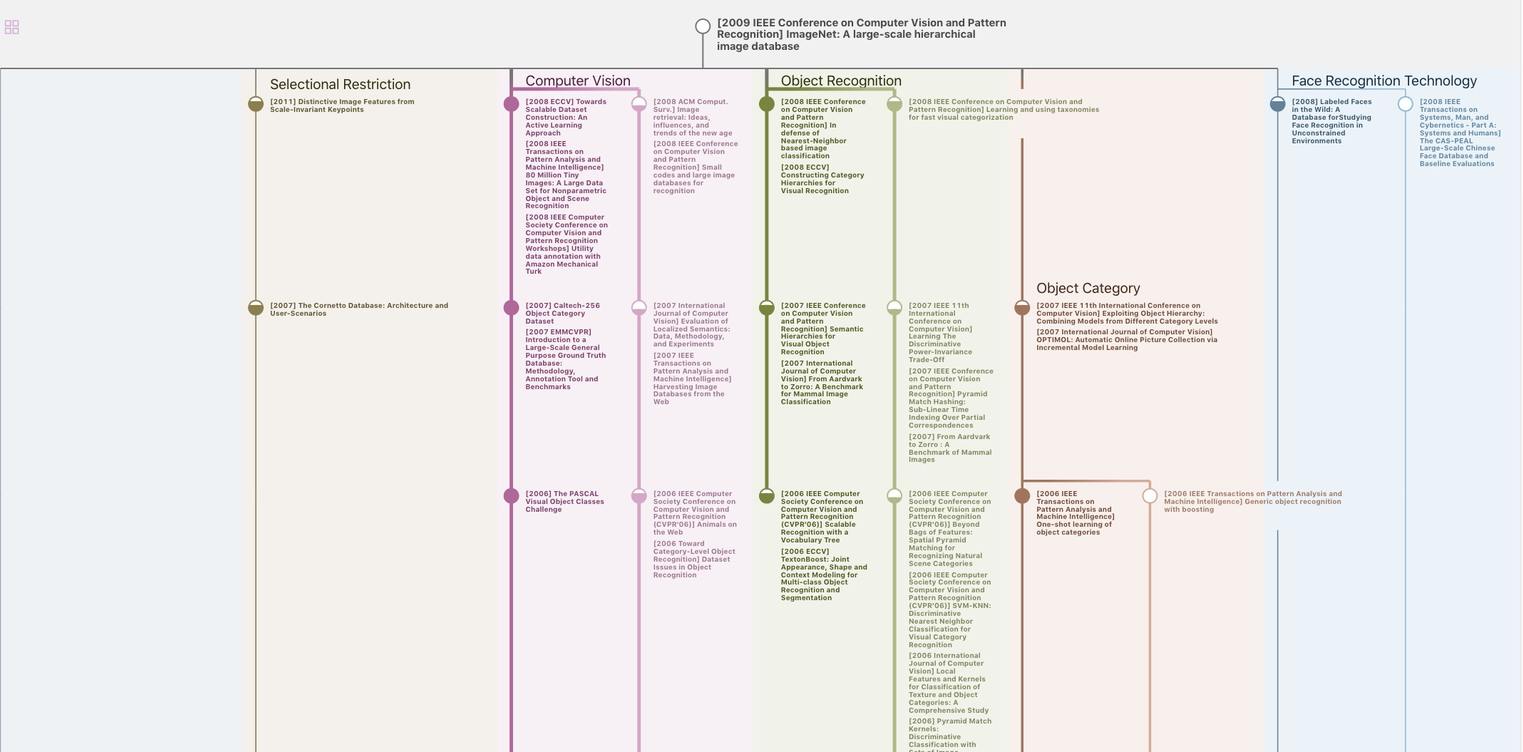
生成溯源树,研究论文发展脉络
Chat Paper
正在生成论文摘要