Coupling machine learning and epidemiological modelling to characterise optimal fungicide doses when fungicide resistance is partial or quantitative
Journal of the Royal Society, Interface(2022)
摘要
Increasing fungicide dose tends to lead to better short-term control of plant diseases. However, high doses select more rapidly for fungicide resistant strains, reducing long-term disease control. When resistance is qualitative and complete – i.e. resistant strains are unaffected by the chemical and resistance requires only a single genetic change – using the lowest possible dose ensuring sufficient control is well-known as the optimal resistance management strategy. However, partial resistance (where resistant strains are still partially suppressed by the fungicide) and quantitative resistance (where a range of resistant strains are present) remain ill-understood. Here we use a model of quantitative fungicide resistance (parameterised for the economically-important fungal pathogen Zymoseptoria tritici ) which handles qualitative partial resistance as a special case. We show that – for both qualitative partial resistance and quantitative resistance – although low doses are optimal for resistance management, for some model parameterisations the benefit does not outweigh the improvement in control from increasing doses. Via a machine learning approach (a gradient-boosted trees model combined with Shapley values to facilitate interpretability) we interpret the effect of parameters controlling pathogen mutation and characterising the fungicide, in addition to the timescale of interest.
### Competing Interest Statement
The authors have declared no competing interest.
更多查看译文
关键词
fungicide resistance,optimal fungicide doses,epidemiological modelling,machine learning
AI 理解论文
溯源树
样例
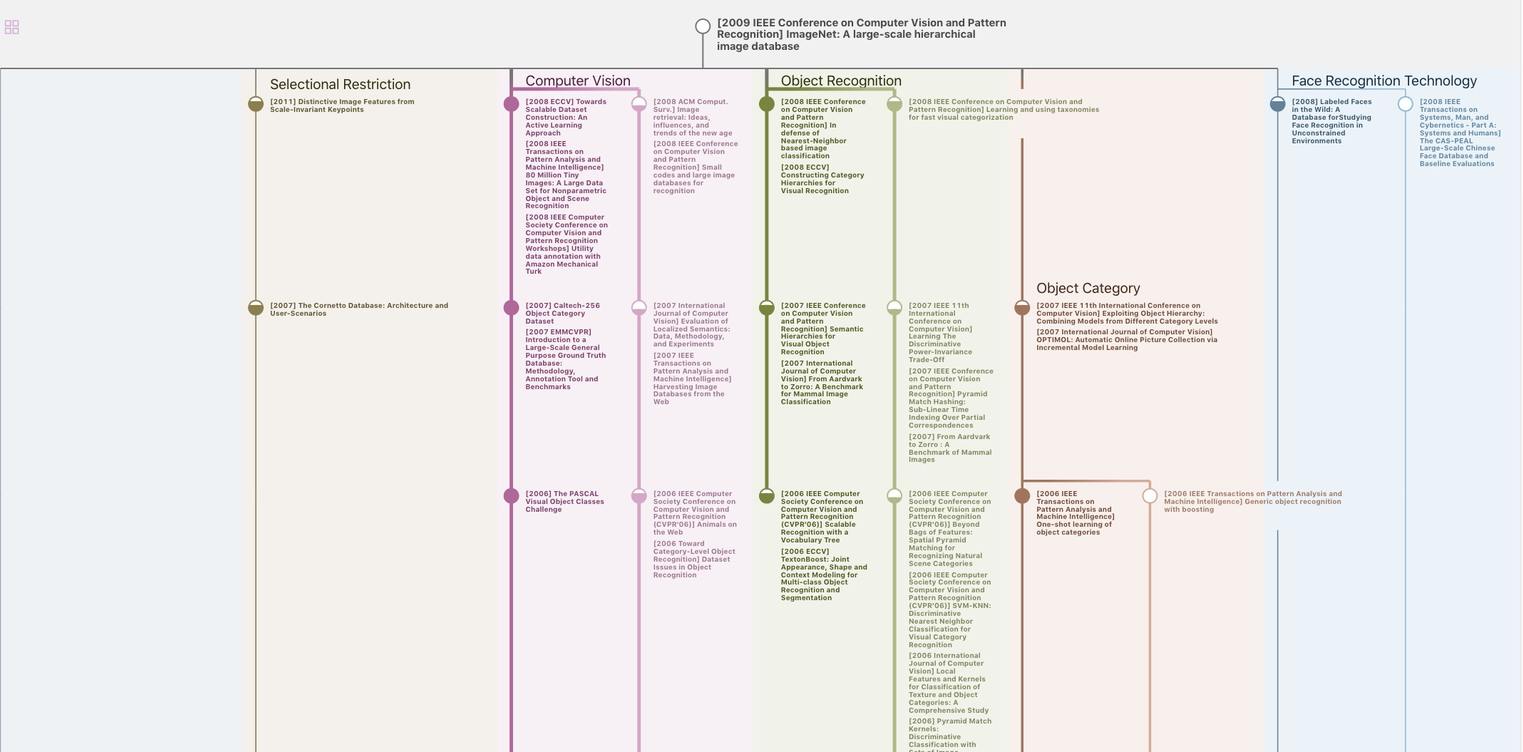
生成溯源树,研究论文发展脉络
Chat Paper
正在生成论文摘要