Probabilistic 3D-modelling of genomes and genomic domains by integrating high-throughput imaging and Hi-C using machine learning
biorxiv(2022)
摘要
Among the existing techniques for interrogating the genome structure, Hi-C assays have become the most performed experiments and constitute the majority of the publicly available datasets. As a result, there is a continuous demand to create and improve algorithms and methods to assist the scientific community in the interpretation of Hi-C experimental data. Here we introduce probabilistic TADbit (pTADbit), a new approach that combines Deep Learning and restraint-based modelling to infer the three-dimensional (3D) structure of genome and genomic domains interrogated by Hi-C experiments. pTADbit uses thousands of microscopy-based distances between genomic loci to train a neural network model that aims at predicting the population distribution of the spatial distance between two genomic loci based solely on their Hi-C interaction frequency. pTADbit produces more accurate chromatin models compared to the original TADbit as well as other available 3D modeling methods, while drastically reducing the required computation time. The resulting ensemble of models not only agree consistently with independent measures obtained by imaging experiments but also better capture the heterogeneity of the cell population. The development of pTADbit lays the basis for the integration of data produced from high-throughput imaging assays into the 3D modelling genomes and genomic domains.
### Competing Interest Statement
The authors have declared no competing interest.
更多查看译文
关键词
genomes,genomic domains,imaging,d-modelling,high-throughput
AI 理解论文
溯源树
样例
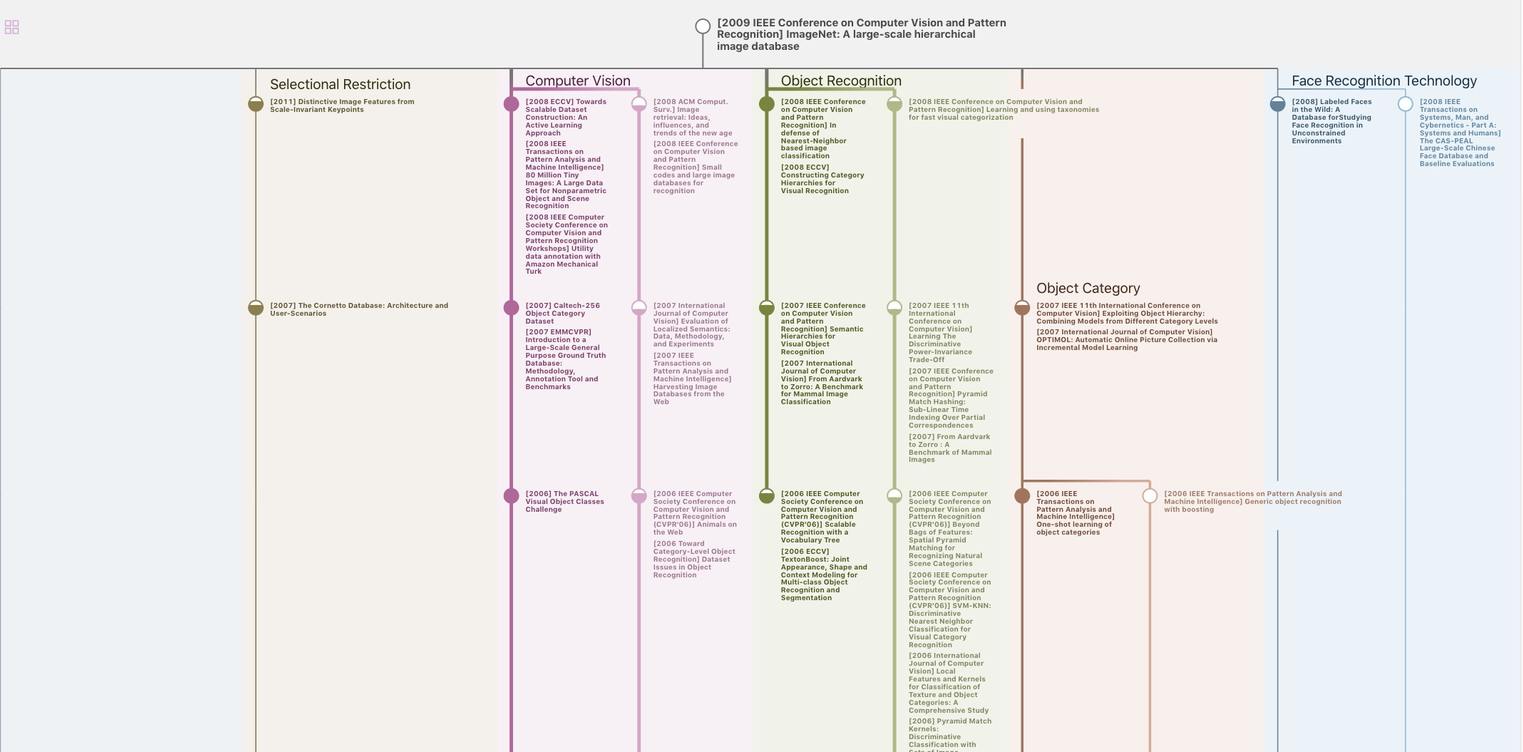
生成溯源树,研究论文发展脉络
Chat Paper
正在生成论文摘要