Ultra-Robust Real-Time Estimation of Gait Phase
IEEE TRANSACTIONS ON NEURAL SYSTEMS AND REHABILITATION ENGINEERING(2022)
摘要
An ultra-robust accurate gait phase estimator is developed by training a time-delay neural network (D67) on data collected from the hip and knee joint angles of 14 participants during treadmill and overground walking. Collected data include normal gait at speeds ranging from 0.1m/s to 1.9m/s and conditions such as long stride, short stride, asymmetric walking, stop-start, and abrupt speed changes. Spatial analysis of our method indicates an average RMSE of 1.74 +/- 0.23% and 2.35 +/- 0.52% in gait phase estimation of test participants in the treadmill and overground walking, respectively. The temporal analysis reveals that D67 detects heel-strike events with an average MAE of 1.70 +/- 0.54% and 2.74 +/- 0.92% of step duration on test participants in the treadmill and overground walking, respectively. Both spatial and temporal performances are uniform across participants and gait conditions. Further analyses indicate the robustness of the D67 to smooth and abrupt speed changes, limping, variation of stride length, and sudden start or stop of walking. The performance of the D67 is also compared to the state-of-the-art techniques confirming the superior and comparable performance of the D67 to techniques without and with a ground contact sensor, respectively. The estimator is finally tested on a participant walking with an active exoskeleton, demonstrating the robustness of D67 in interaction with an exoskeleton without being trained on any data from the test subject with or without an exoskeleton.
更多查看译文
关键词
Legged locomotion,Exoskeletons,Hip,Training,Phase estimation,Estimation,Belts,Gait phase,gait variability,pathological gait,rehabilitation,exoskeleton
AI 理解论文
溯源树
样例
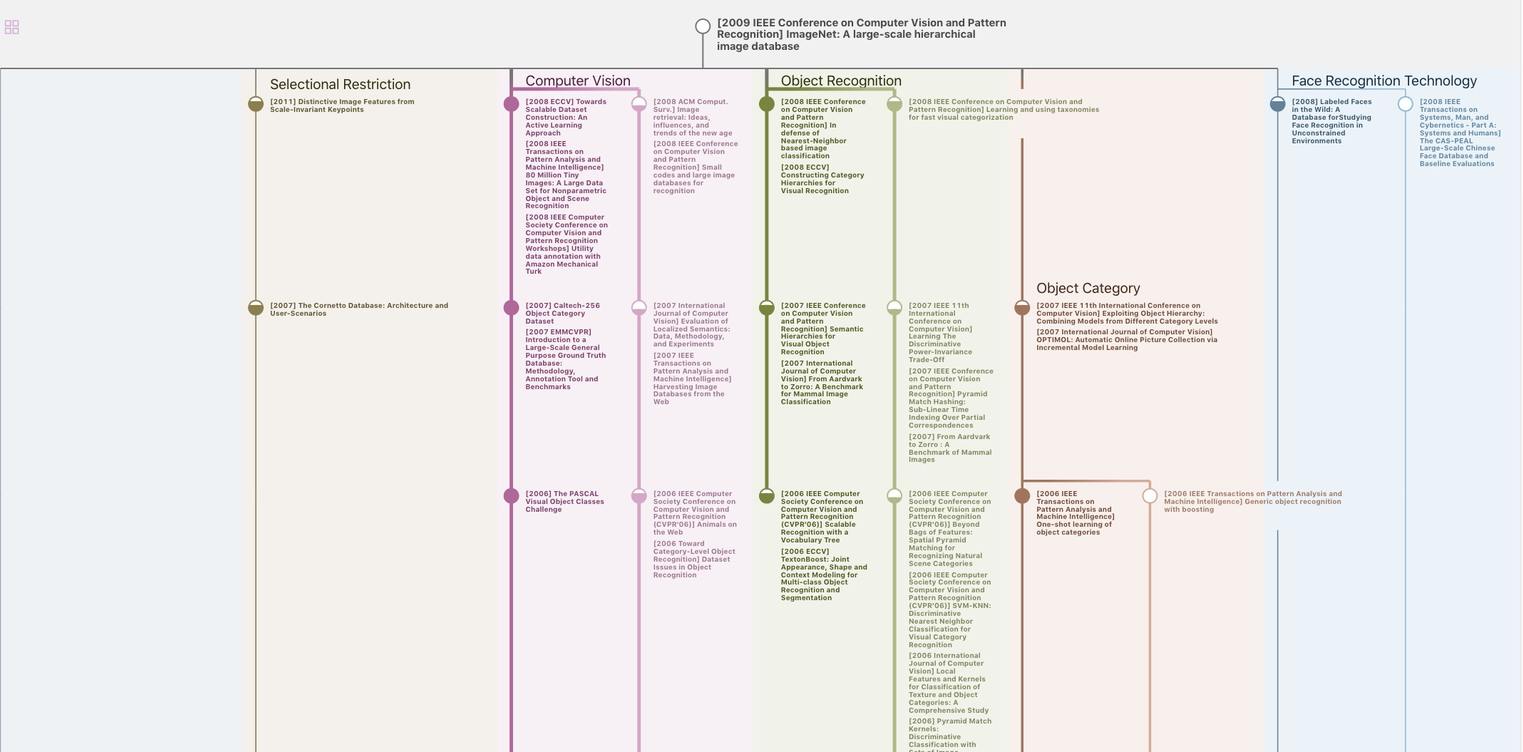
生成溯源树,研究论文发展脉络
Chat Paper
正在生成论文摘要