Short-Term Load Forecasting Based on Radam Optimized CNN-BiLSTM-AE Hybrid Model
2022 Power System and Green Energy Conference (PSGEC)(2022)
摘要
Reliable short term load forecasting (STLF) is significant in the operation and planning of power systems. With the increasing demand for the accuracy of load prediction in modern society, neural network models are basically regarded as the mainstream model in the field of load prediction. However, based on a single AI model, the algorithm cannot adequately capture the potential characteristics of the load sequence. In the following study, we proposed a CNN-BiLSTM-AE model to improve the accuracy of load prediction further. The model applies the convolution layer of CNN to capture the spatial feature of power load data, and BiLSTM is used to capture temporal features that satisfy the power load data. Both the short and the long time series are input into the encoder, which can abstract features of diverse degrees and produce latent vectors related to them. Then, the input sequence is rebuilt in the decoder to guarantee that the potential vectors are meaningful and improve the exactness of short-term prediction. In order to further enhance the accuracy of prediction, a new optimizer Radam and attention mechanism are proposed in the experiment.
更多查看译文
关键词
Convolution network,Attention mechanism,Hybrid model.Radam,STLF
AI 理解论文
溯源树
样例
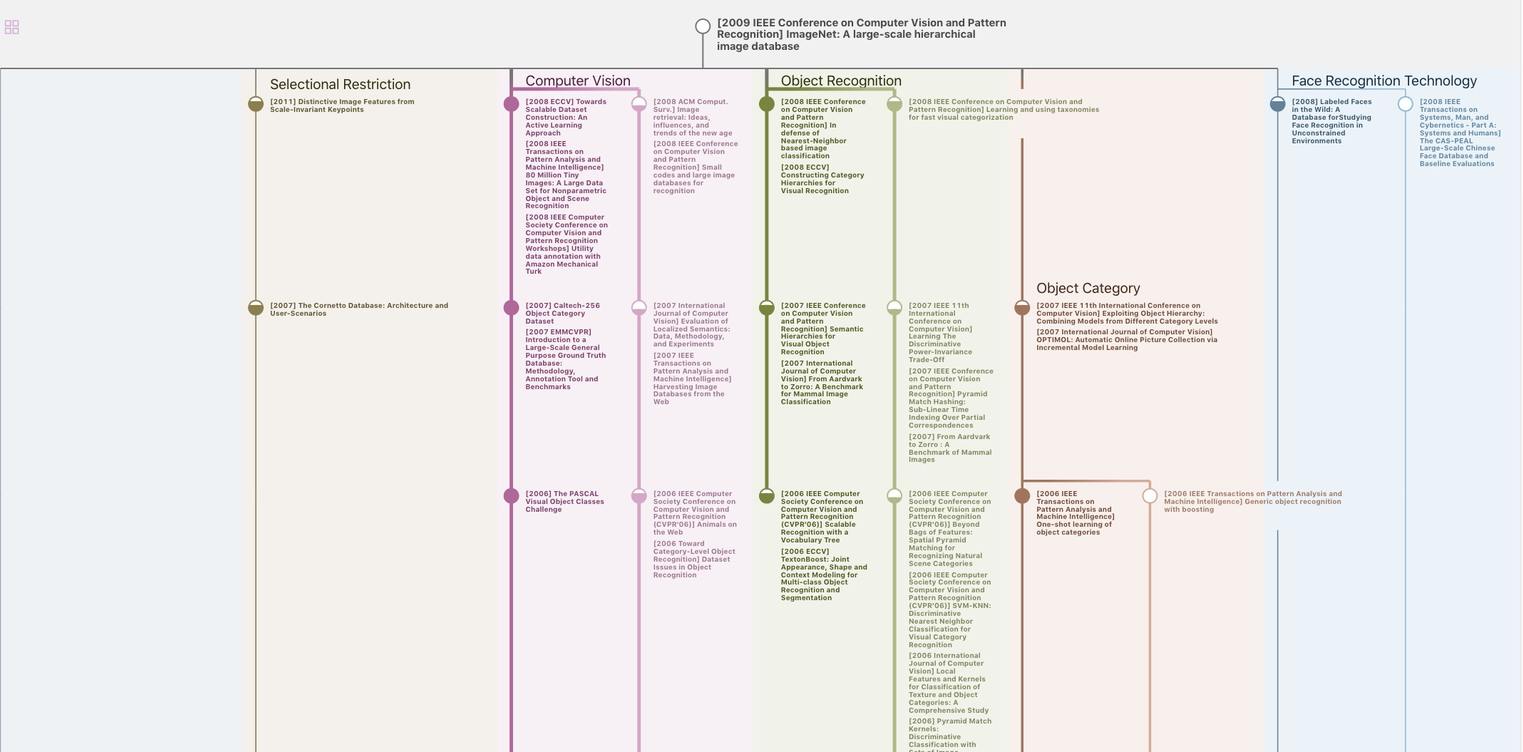
生成溯源树,研究论文发展脉络
Chat Paper
正在生成论文摘要