Finite- and Large- Sample Inference for Model and Coefficients in High-dimensional Linear Regression with Repro Samples
arxiv(2022)
摘要
In this paper, we present a new and effective simulation-based approach to conduct both finite- and large-sample inference for high-dimensional linear regression models. This approach is developed under the so-called repro samples framework, in which we conduct statistical inference by creating and studying the behavior of artificial samples that are obtained by mimicking the sampling mechanism of the data. We obtain confidence sets for (a) the true model corresponding to the nonzero coefficients, (b) a single or any collection of regression coefficients, and (c) both the model and regression coefficients jointly. We also extend our approaches to drawing inferences on functions of the regression coefficients. The proposed approach fills in two major gaps in the high-dimensional regression literature: (1) lack of effective approaches to address model selection uncertainty and provide valid inference for the underlying true model; (2) lack of effective inference approaches that guarantee finite-sample performances. We provide both finite-sample and asymptotic results to theoretically guarantee the performances of the proposed methods. In addition, our numerical results demonstrate that the proposed methods are valid and achieve better coverage with smaller confidence sets than the existing state-of-art approaches, such as debiasing and bootstrap approaches.
更多查看译文
关键词
samples,model,high-dimensional
AI 理解论文
溯源树
样例
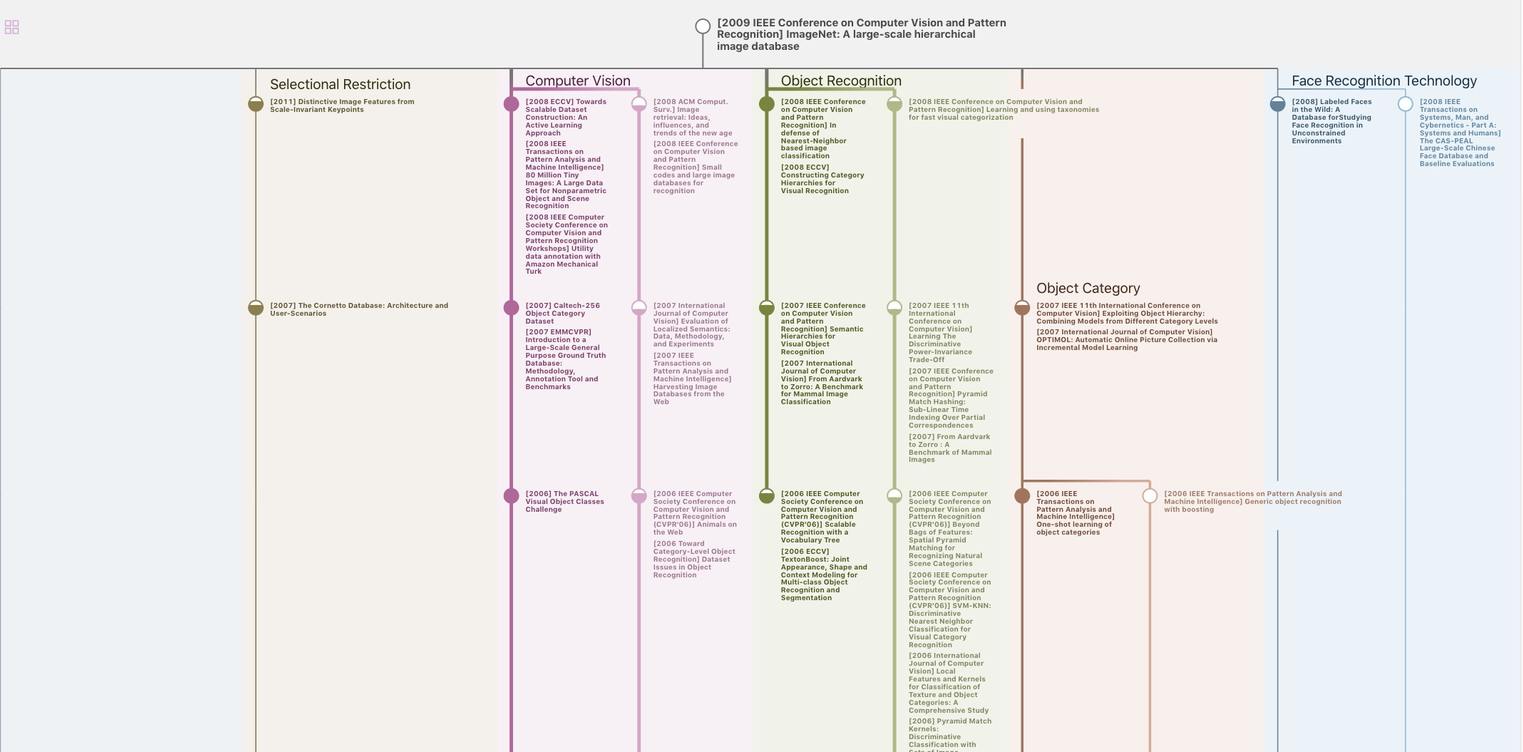
生成溯源树,研究论文发展脉络
Chat Paper
正在生成论文摘要