An End-to-End Transformer with Progressive Tri-Modal Attention for Multi-modal Emotion Recognition
PATTERN RECOGNITION AND COMPUTER VISION, PRCV 2023, PT VII(2024)
摘要
Recent works on multi-modal emotion recognition move towards end-to-end models, which can extract the task-specific features supervised by the target task compared with the two-phase pipeline. In this paper, we propose a novel multi-modal end-to-end transformer for emotion recognition, which can effectively model the tri-modal features interaction among the textual, acoustic, and visual modalities at the low-level and high-level. At the low-level, we propose the progressive tri-modal attention, which can model the tri-modal feature interactions by adopting a two-pass strategy and can further leverage such interactions to significantly reduce the computation and memory complexity through reducing the input token length. At the high-level, we introduce the tri-modal feature fusion layer to explicitly aggregate the semantic representations of three modalities. The experimental results on the CMU-MOSEI and IEMOCAP datasets show that ME2ET achieves the state-of-the-art performance. The further in-depth analysis demonstrates the effectiveness, efficiency, and interpretability of the proposed tri-modal attention, which can help our model to achieve better performance while significantly reducing the computation and memory cost (Our code is available at https://github.com/SCIR-MSA-Team/UFMAC.).
更多查看译文
关键词
Multi-modal emotion recognition,Multi-modal transformer,Feature fusion
AI 理解论文
溯源树
样例
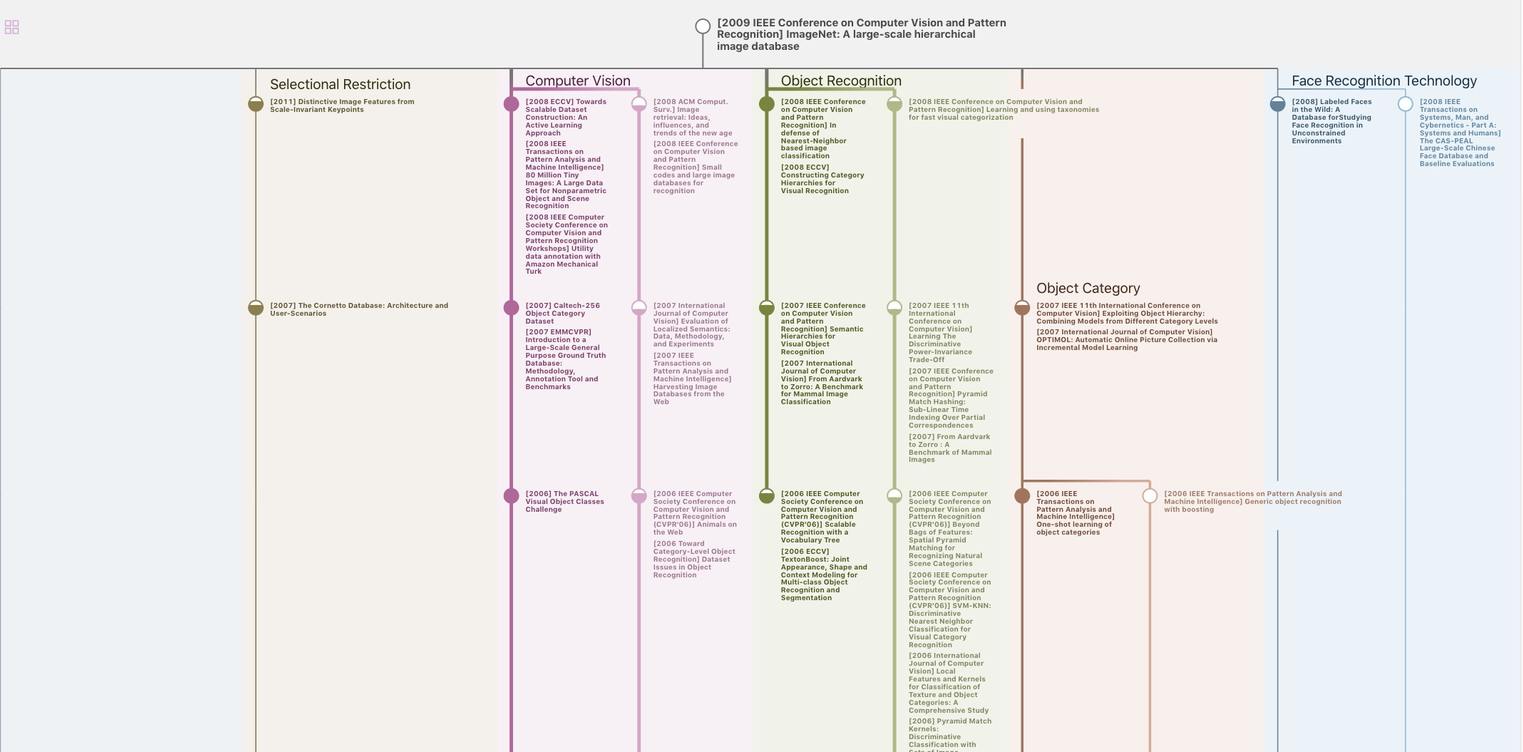
生成溯源树,研究论文发展脉络
Chat Paper
正在生成论文摘要