Default risk prediction and feature extraction using a penalized deep neural network
Statistics and Computing(2022)
摘要
Online peer-to-peer lending platforms provide loans directly from lenders to borrowers without passing through traditional financial institutions. For lenders on these platforms to avoid loss, it is crucial that they accurately assess default risk so that they can make appropriate decisions. In this study, we develop a penalized deep learning model to predict default risk based on survival data. As opposed to simply predicting whether default will occur, we focus on predicting the probability of default over time. Moreover, by adding an additional one-to-one layer in the neural network, we achieve feature selection and estimation simultaneously by incorporating an L_1 -penalty into the objective function. The minibatch gradient descent algorithm makes it possible to handle massive data. An analysis of a real-world loan data and simulations demonstrate the model’s competitive practical performance, which suggests favorable potential applications in peer-to-peer lending platforms.
更多查看译文
关键词
Feature extraction,Loan data,Neural network,Risk prediction,Survival analysis
AI 理解论文
溯源树
样例
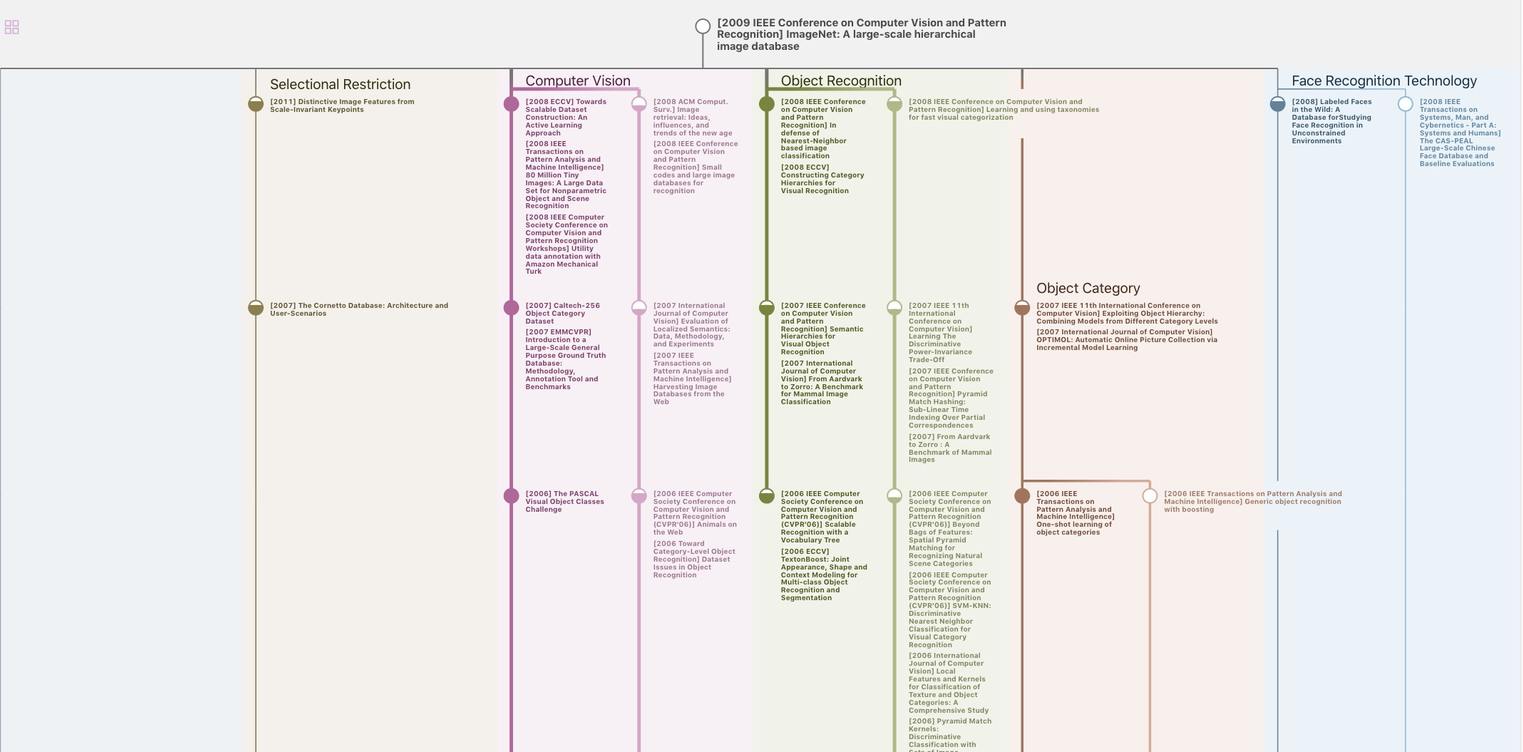
生成溯源树,研究论文发展脉络
Chat Paper
正在生成论文摘要