Bayesian Machine Learning for Extracting Parasitic Parameters of GSG Calibration Standards
2022 Asia-Pacific International Symposium on Electromagnetic Compatibility (APEMC)(2022)
摘要
This paper proposes a method to extract the parasitic parameters of the calibration standards by using the Bayesian optimization algorithm. After several rounds of optimization, the parasitic parameters are optimized so that the calculated value is closest to the full-wave simulation value. The advantage is that only one S-parameter of a known device needs to be used to obtain the parasitic parameters of all calibration standards. Compared with traditional measurement methods, it has the advantages taking less measurement times and coverage of frequency bandwidth. To prove the validity of the technique, the S-parameter of the 4mm microstrip line was simulated as a reference. The extracted parasitic parameters were used in the 16-term error model, the calibration and simulation results are in good agreement up to 100GHz.
更多查看译文
关键词
bayesian machine learning,parasitic parameters,machine learning
AI 理解论文
溯源树
样例
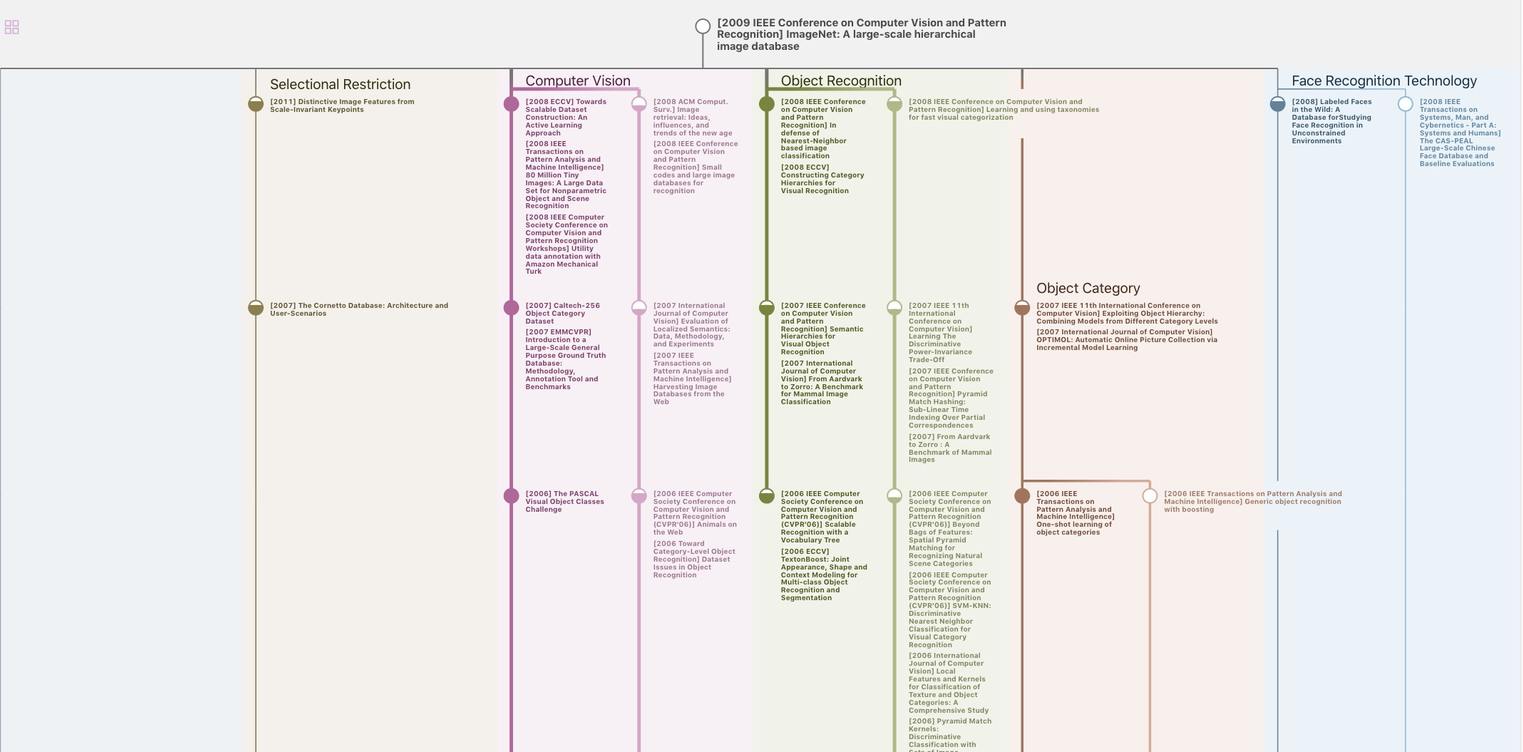
生成溯源树,研究论文发展脉络
Chat Paper
正在生成论文摘要