Towards ML-Based Diagnostics of Focused Laser Pulse
arxiv(2023)
摘要
Currently, machine learning (ML) methods are widely used to process the results of physical experiments. In some cases, due to the limited amount of real experimental data, ML-models can be pre-trained on synthetic data simulated based on the analytical theory and then fine-tuned using real experimental data. A limitation of this approach is the presence of the latent parameters of the analytical model, which values are difficult or impossible to estimate. Setting these parameters incorrectly may induce a dataset shift even when applied to synthetic data. To overcome this problem, we train the ML-model on a dataset with randomly varied latent parameters of the analythical model to force the ML-model to concentrate on more general patterns that depend weakly on the latent parameters. We applied this approach to the problem of tight focusing of a laser pulse with the complex structure of the wavefront. We observed good accuracy of reconstructing of the tilt parameters when training and testing the ML-model on datasets generated for different values of the latent parameters. This confirms that the ML-model was able to select relevant information without over-fitting for specific features inherent in certain values of the latent parameters. We believe that this approach will enrich possible applications of ML-methods to an experimental diagnostics of laser pulses.
更多查看译文
关键词
tight focusing,deep learning,convolutional neural network,dataset shift
AI 理解论文
溯源树
样例
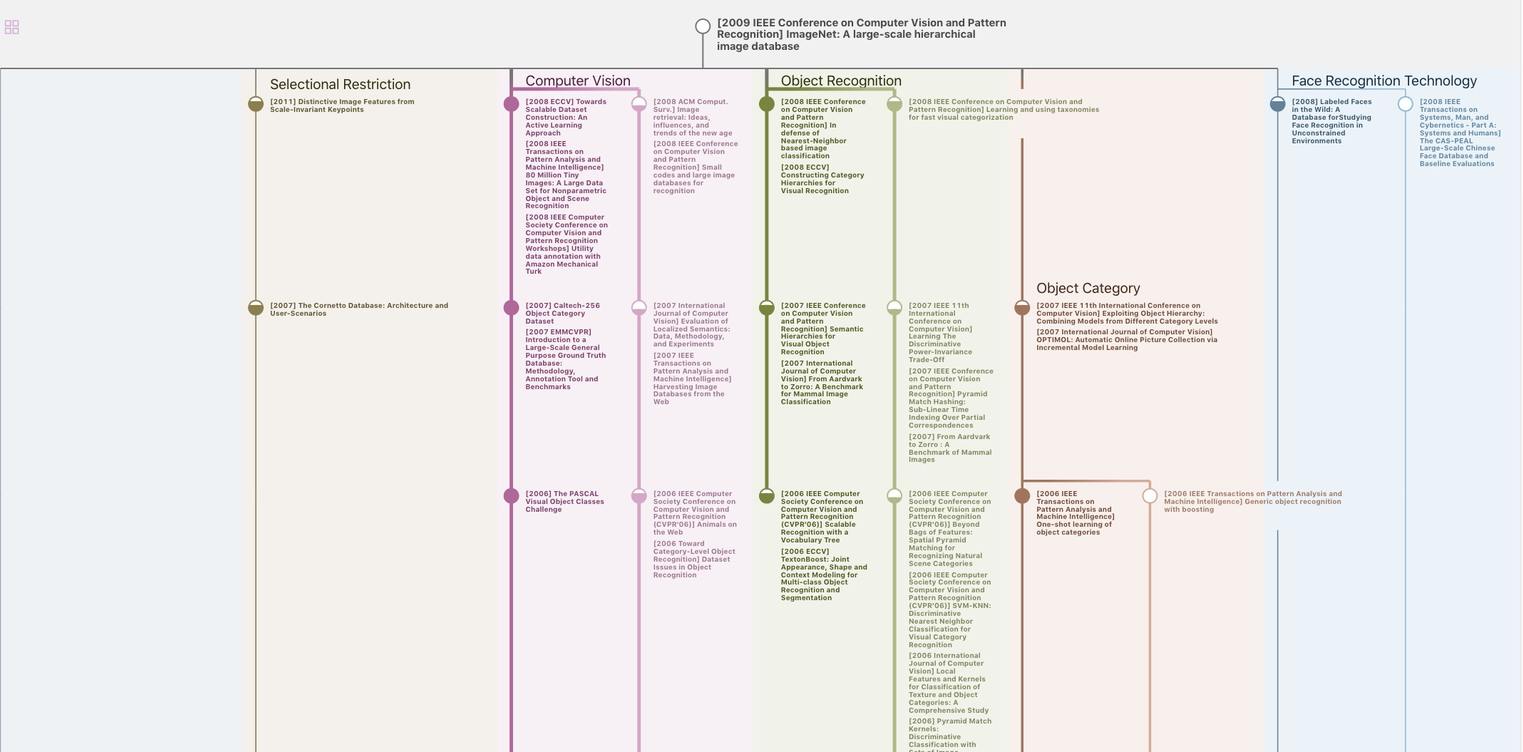
生成溯源树,研究论文发展脉络
Chat Paper
正在生成论文摘要