Large-Sample Properties of Non-Stationary Source Separation for Gaussian Signals
arxiv(2022)
摘要
Non-stationary source separation is a well-established branch of blind source separation with many different methods. However, for none of these methods large-sample results are available. To bridge this gap, we develop large-sample theory for NSS-JD, a popular method of non-stationary source separation based on the joint diagonalization of block-wise covariance matrices. We work under an instantaneous linear mixing model for independent Gaussian non-stationary source signals together with a very general set of assumptions: besides boundedness conditions, the only assumptions we make are that the sources exhibit finite dependency and that their variance functions differ sufficiently to be asymptotically separable. The consistency of the unmixing estimator and its convergence to a limiting Gaussian distribution at the standard square root rate are shown to hold under the previous conditions. Simulation experiments are used to verify the theoretical results and to study the impact of block length on the separation.
更多查看译文
关键词
gaussian
AI 理解论文
溯源树
样例
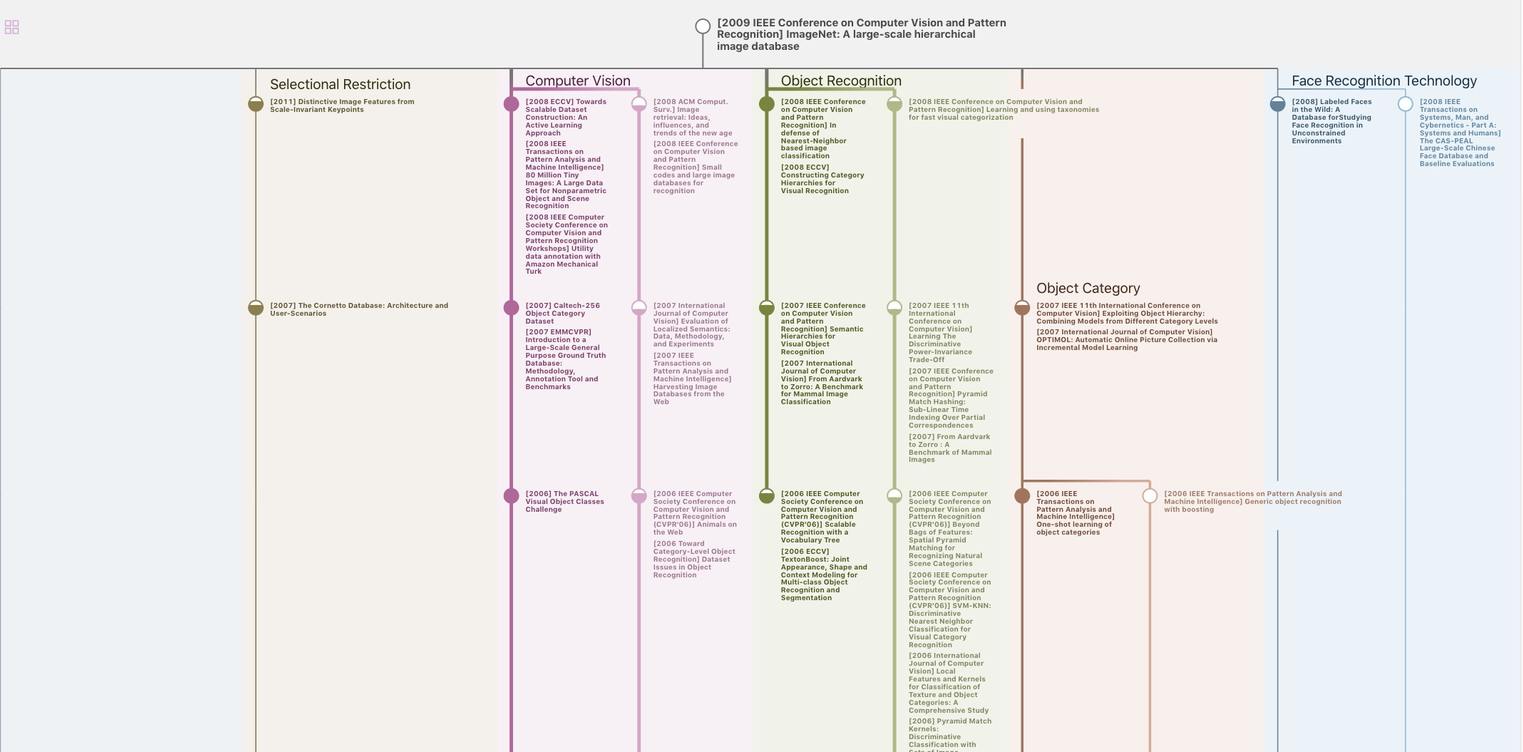
生成溯源树,研究论文发展脉络
Chat Paper
正在生成论文摘要