State of Health Trajectory Prediction Based on Multi-Output Gaussian Process Regression for Lithium-Ion Battery
BATTERIES-BASEL(2022)
摘要
Lithium-ion battery state of health (SOH) accurate prediction is of great significance to ensure the safe reliable operation of electric vehicles and energy storage systems. However, safety issues arising from the inaccurate estimation and prediction of battery SOH have caused widespread concern in academic and industrial communities. In this paper, a method is proposed to build an accurate SOH prediction model for battery packs based on multi-output Gaussian process regression (MOGPR) by employing the initial cycle data of the battery pack and the entire life cycling data of battery cells. Firstly, a battery aging experimental platform is constructed to collect battery aging data, and health indicators (HIs) that characterize battery aging are extracted. Then, the correlation between the HIs and the battery capacity is evaluated by the Pearson correlation analysis method, and the HIs that own a strong correlation to the battery capacity are screened. Finally, two MOGPR models are constructed to predict the HIs and SOH of the battery pack. Based on the first MOGPR model and the early HIs of the battery pack, the future cycle HIs can be predicted. In addition, the predicted HIs and the second MOGPR model are used to predict the SOH of the battery pack. The experimental results verify that the approach has a competitive performance; the mean and maximum values of the mean absolute error (MAE) and root mean square error (RMSE) are 1.07% and 1.42%, and 1.77% and 2.45%, respectively.
更多查看译文
关键词
lithium-ion battery,health indicators,state of health,multi-output Gaussian process regression,health prediction
AI 理解论文
溯源树
样例
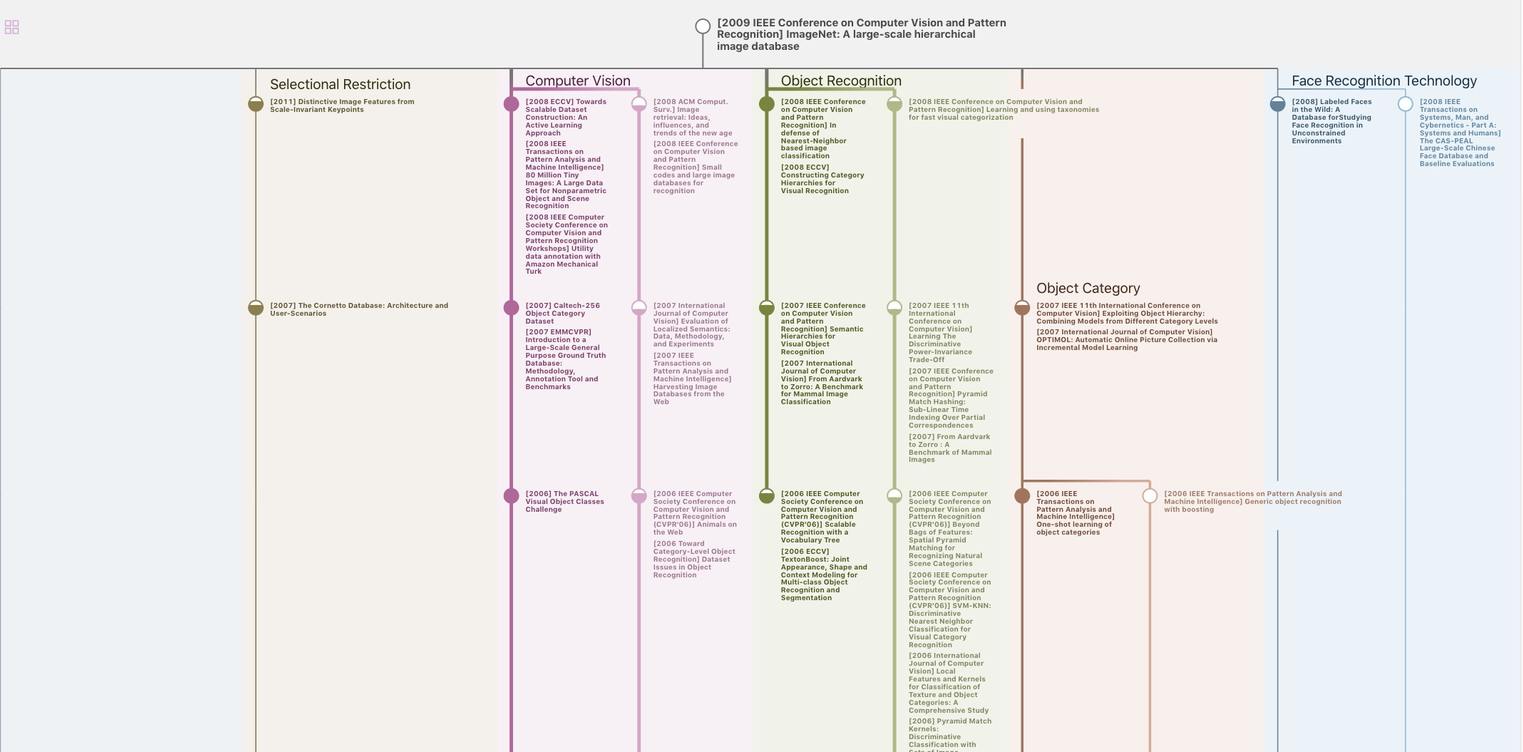
生成溯源树,研究论文发展脉络
Chat Paper
正在生成论文摘要