Evaluation and comparison of unsupervised methods for the extraction of spatial patterns from mass spectrometry imaging data (MSI)
SCIENTIFIC REPORTS(2022)
摘要
For the extraction of spatially important regions from mass spectrometry imaging (MSI) data, different clustering methods have been proposed. These clustering methods are based on certain assumptions and use different criteria to assign pixels into different classes. For high-dimensional MSI data, the curse of dimensionality also limits the performance of clustering methods which are usually overcome by pre-processing the data using dimension reduction techniques. In summary, the extraction of spatial patterns from MSI data can be done using different unsupervised methods, but the robust evaluation of clustering results is what is still missing. In this study, we have performed multiple simulations on synthetic and real MSI data to validate the performance of unsupervised methods. The synthetic data were simulated mimicking important spatial and statistical properties of real MSI data. Our simulation results confirmed that K-means clustering with correlation distance and Gaussian Mixture Modeling clustering methods give optimal performance in most of the scenarios. The clustering methods give efficient results together with dimension reduction techniques. From all the dimension techniques considered here, the best results were obtained with the minimum noise fraction (MNF) transform. The results were confirmed on both synthetic and real MSI data. However, for successful implementation of MNF transform the MSI data requires to be of limited dimensions.
更多查看译文
关键词
mass spectrometry imaging data,mass spectrometry,extraction,unsupervised methods,spatial patterns
AI 理解论文
溯源树
样例
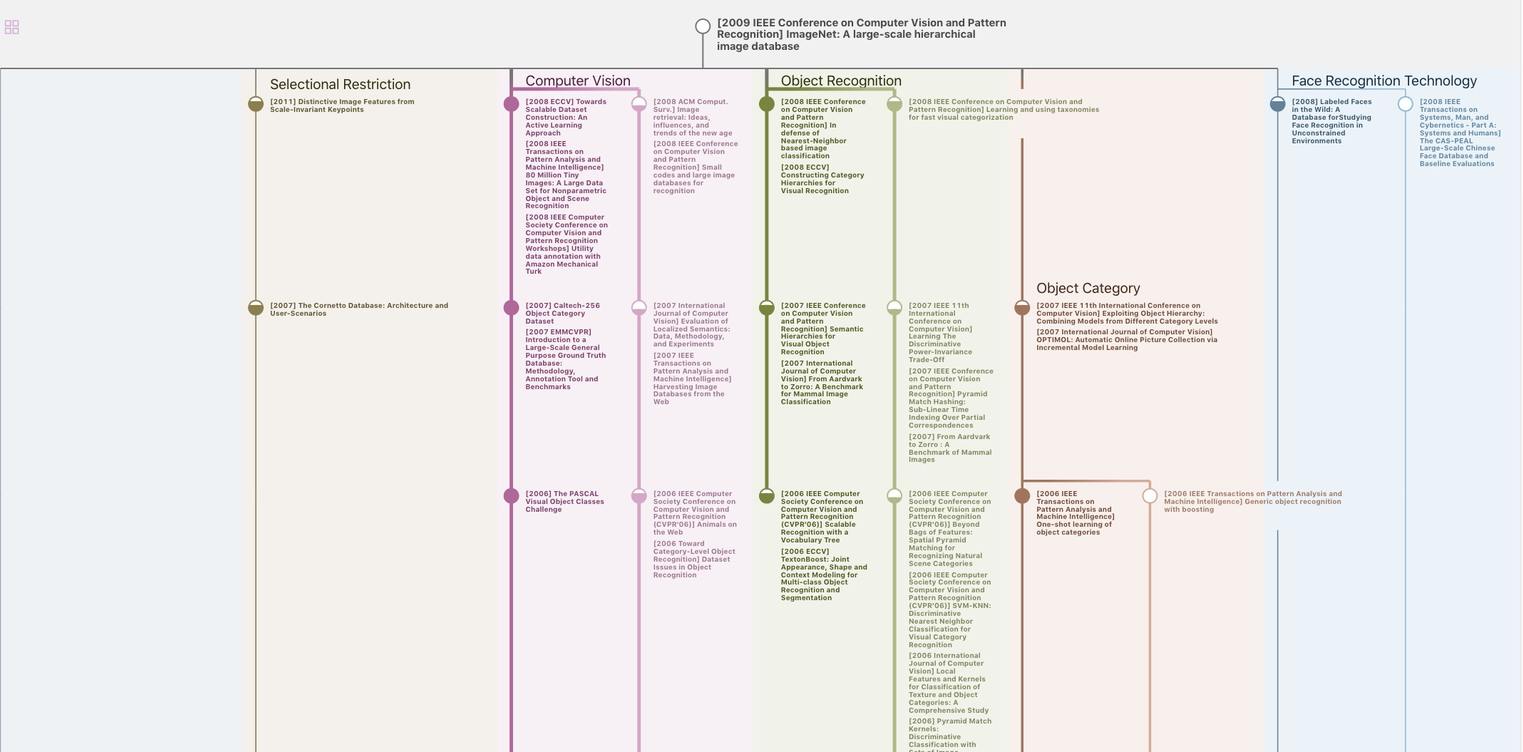
生成溯源树,研究论文发展脉络
Chat Paper
正在生成论文摘要