Reward-Penalty Weighted Ensemble for Emotion State Classification from Multi-Modal Data Streams.
Int. J. Neural Syst.(2022)
摘要
Researchers have shown the limitations of using the single-modal data stream for emotion classification. Multi-modal data streams are therefore deemed necessary to improve the accuracy and performance of online emotion classifiers. An online decision ensemble is a widely used approach to classify emotions in real-time using multi-modal data streams. There is a plethora of online ensemble approaches; these approaches use a fixed parameter ([Formula: see text]) to adjust the weights of each classifier (called penalty) in case of wrong classification and no reward for a good performing classifier. Also, the performance of the ensemble depends on the [Formula: see text], which is set using trial and error. This paper presents a new Reward-Penalty-based Weighted Ensemble (RPWE) for real-time multi-modal emotion classification using multi-modal physiological data streams. The proposed RPWE is thoroughly tested using two prevalent benchmark data sets, DEAP and AMIGOS. The first experiment confirms the impact of the base stream classifier with RPWE for emotion classification in real-time. The RPWE is compared with different popular and widely used online ensemble approaches using multi-modal data streams in the second experiment. The average balanced accuracy, F1-score results showed the usefulness and robustness of RPWE in emotion classification in real-time from the multi-modal data stream.
更多查看译文
关键词
Affective computing,data stream ensemble,e-learning,multi-modal data stream,real-time emotion classification
AI 理解论文
溯源树
样例
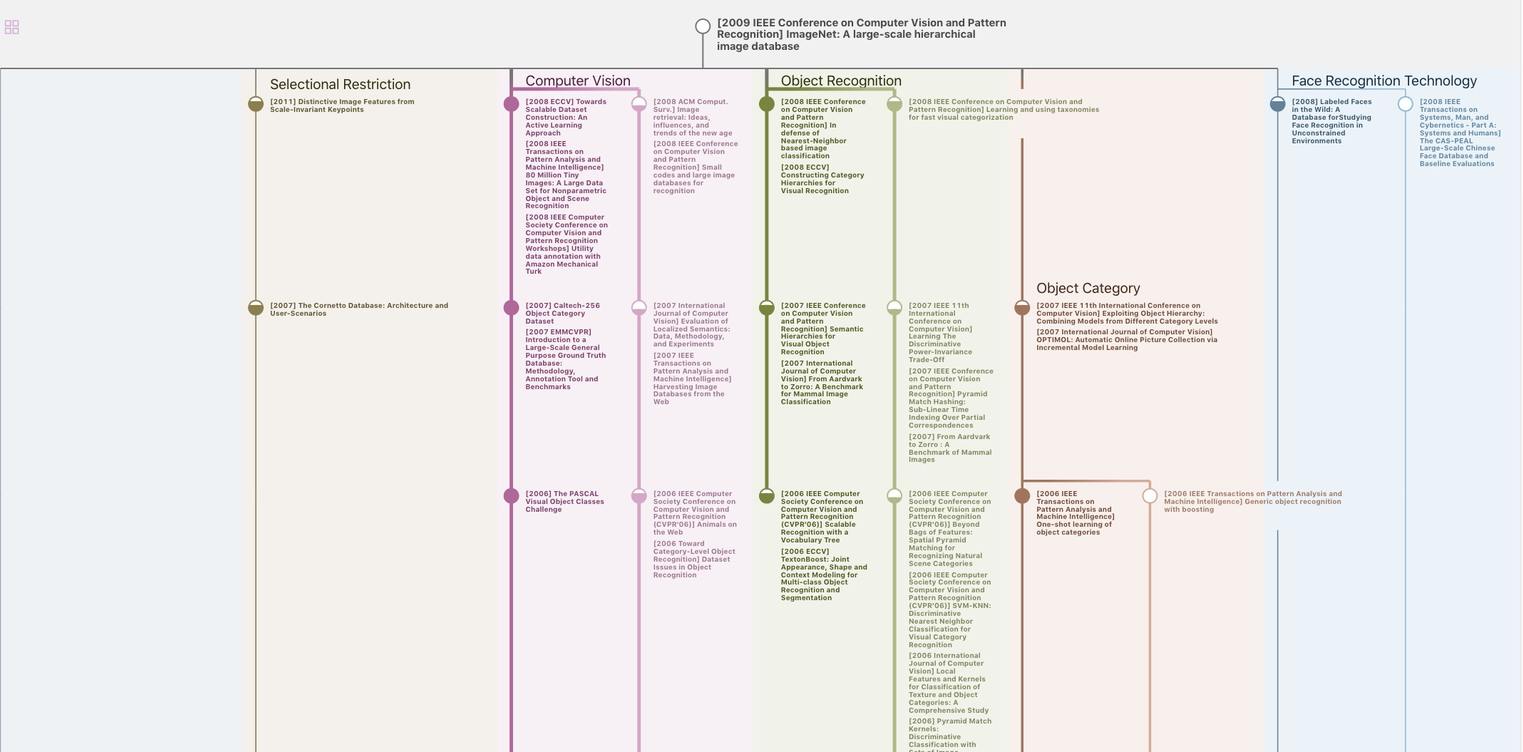
生成溯源树,研究论文发展脉络
Chat Paper
正在生成论文摘要